DCNGAN: A Deformable Convolutional-Based GAN with QP Adaptation for Perceptual Quality Enhancement of Compressed Video
arXiv (Cornell University)(2022)
摘要
In this paper, we propose a deformable convolution-based generative adversarial network (DCNGAN) for perceptual quality enhancement of compressed videos. DCNGAN is also adaptive to the quantization parameters (QPs). Compared with optical flows, deformable convolutions are more effective and efficient to align frames. Deformable convolutions can operate on multiple frames, thus leveraging more temporal information, which is beneficial for enhancing the perceptual quality of compressed videos. Instead of aligning frames in a pairwise manner, the deformable convolution can process multiple frames simultaneously, which leads to lower computational complexity. Experimental results demonstrate that the proposed DCNGAN outperforms other state-of-the-art compressed video quality enhancement algorithms.
更多查看译文
关键词
perceptual quality enhancement,dcngan,qp adaptation,convolutional-based
AI 理解论文
溯源树
样例
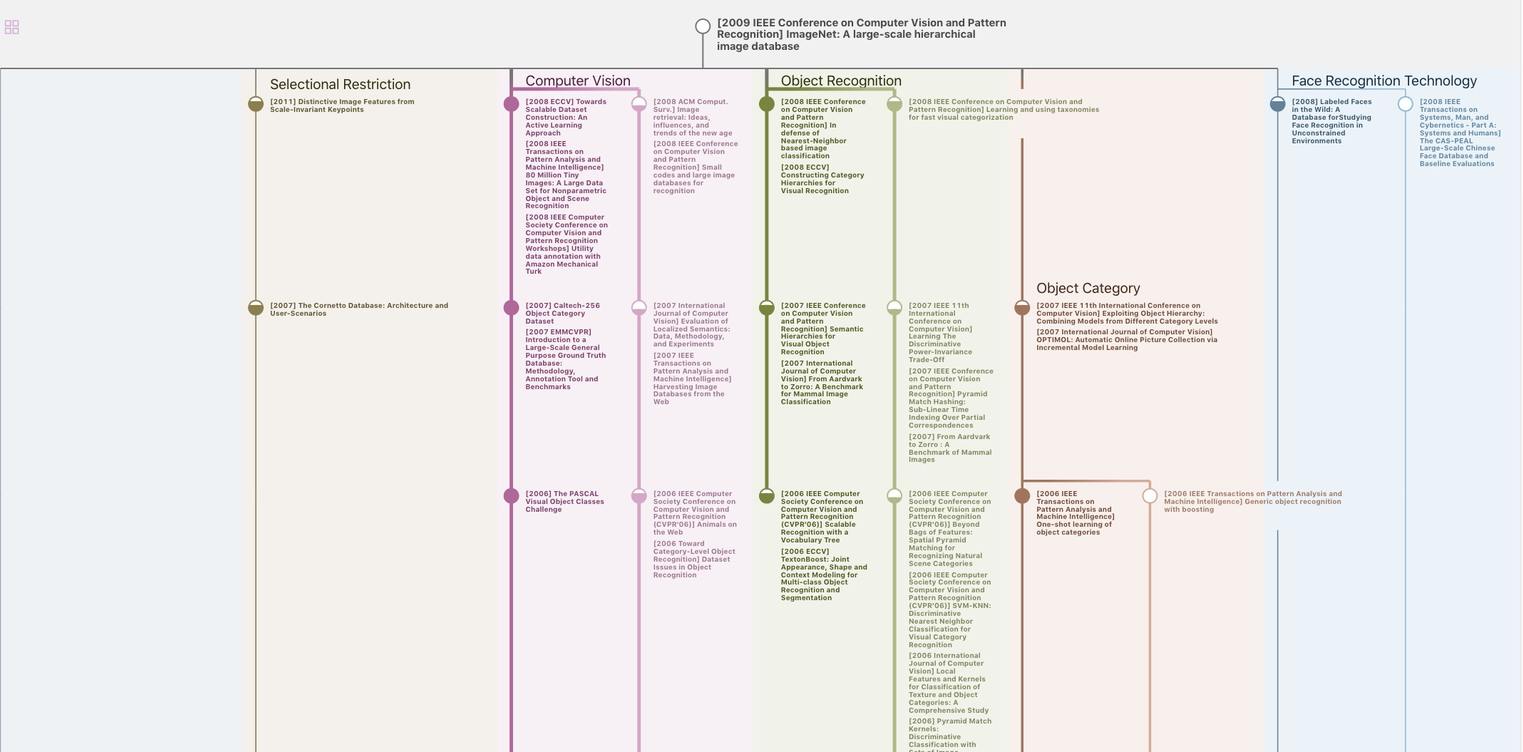
生成溯源树,研究论文发展脉络
Chat Paper
正在生成论文摘要