Is Attention Always Needed? A Case Study on Language Identification from Speech
Social Science Research Network(2022)
摘要
Language Identification (LID) is a crucial preliminary process in the field of Automatic Speech Recognition (ASR) that involves the identification of a spoken language from audio samples. Contemporary systems that can process speech in multiple languages require users to expressly designate one or more languages prior to utilization. The LID task assumes a significant role in scenarios where ASR systems are unable to comprehend the spoken language in multilingual settings, leading to unsuccessful speech recognition outcomes. The present study introduces convolutional recurrent neural network (CRNN) based LID, designed to operate on the Mel-frequency Cepstral Coefficient (MFCC) characteristics of audio samples. Furthermore, we replicate certain state-of-the-art methodologies, specifically the Convolutional Neural Network (CNN) and Attention-based Convolutional Recurrent Neural Network (CRNN with attention), and conduct a comparative analysis with our CRNN-based approach. We conducted comprehensive evaluations on thirteen distinct Indian languages and our model resulted in over 98\% classification accuracy. The LID model exhibits high-performance levels ranging from 97% to 100% for languages that are linguistically similar. The proposed LID model exhibits a high degree of extensibility to additional languages and demonstrates a strong resistance to noise, achieving 91.2% accuracy in a noisy setting when applied to a European Language (EU) dataset.
更多查看译文
关键词
language identification,speech,attention
AI 理解论文
溯源树
样例
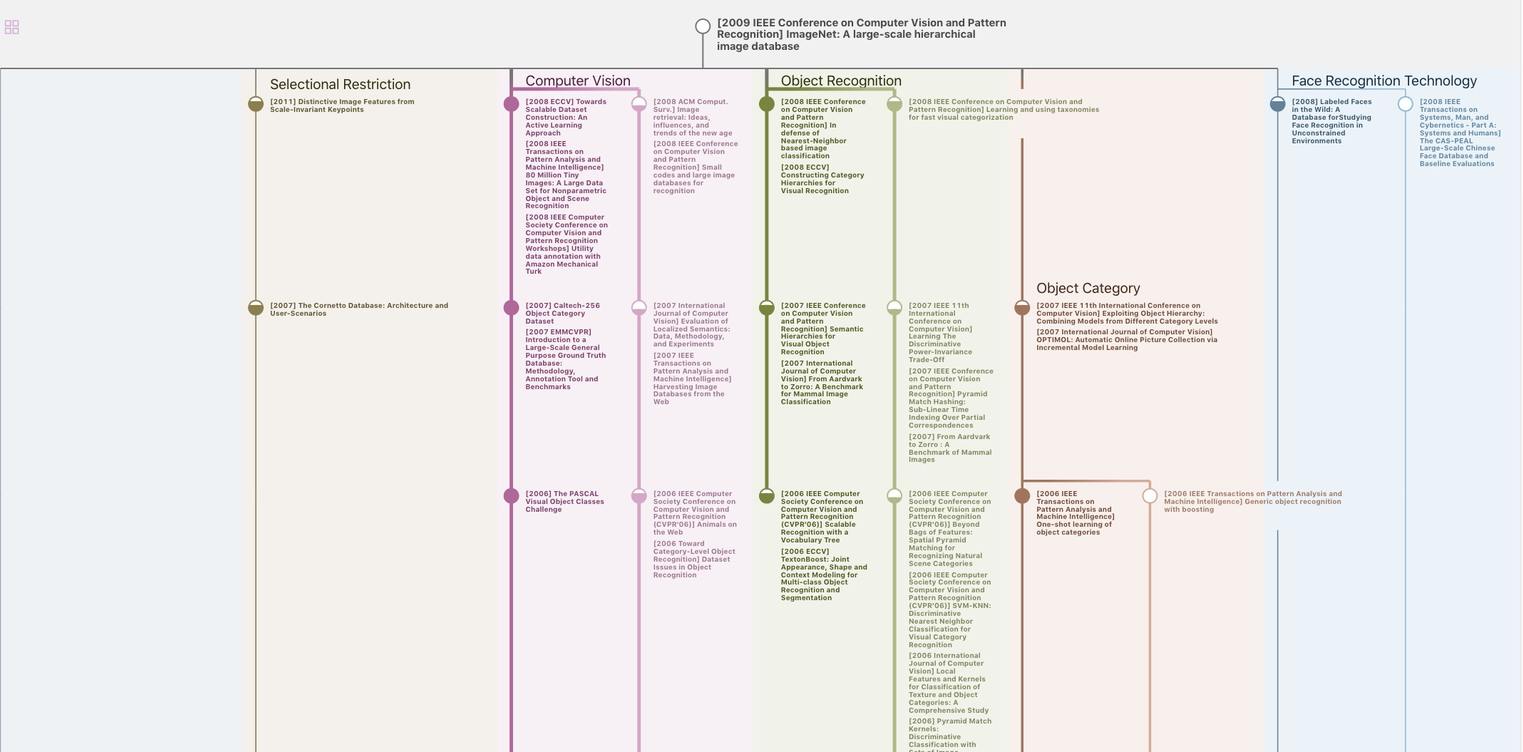
生成溯源树,研究论文发展脉络
Chat Paper
正在生成论文摘要