Dr. MTL: Driver Recommendation using Federated Multi-Task Learning
2023 IEEE 98TH VEHICULAR TECHNOLOGY CONFERENCE, VTC2023-FALL(2023)
摘要
Online vehicle-for-hire service firms such as Ola, Uber, Gett, Lyft, Hailo, Didi, and GrabCab rely on driver recommendations. Several factors should be considered when suggesting a driver for the trip, including ride cost, driver-vehicle-passenger safety, reliability, robustness, and comfort. Multi-task learning has become more widespread in recommender systems in recent years. Our study employs federated multi-objective multitask learning to develop a driver recommendation system that considers drivers' cognitive-physical stress and driving behavior while respecting users' privacy. We use publicly available datasets from UAH-DriveSet, HCI Lab, and PhysioNet for training, testing, and evaluating our system. Our main contribution is a unique framework for driver suggestion based on data-driven federated multi-task learning that achieves the best F-measure accuracy. Our proposed system outperforms baseline and state-of-the-art deep learning models. It detects driver stress and behavior with 95% and 96% F-measures, respectively.
更多查看译文
关键词
driver recommendation,federated multi-task learning,road safety,intelligent transportation
AI 理解论文
溯源树
样例
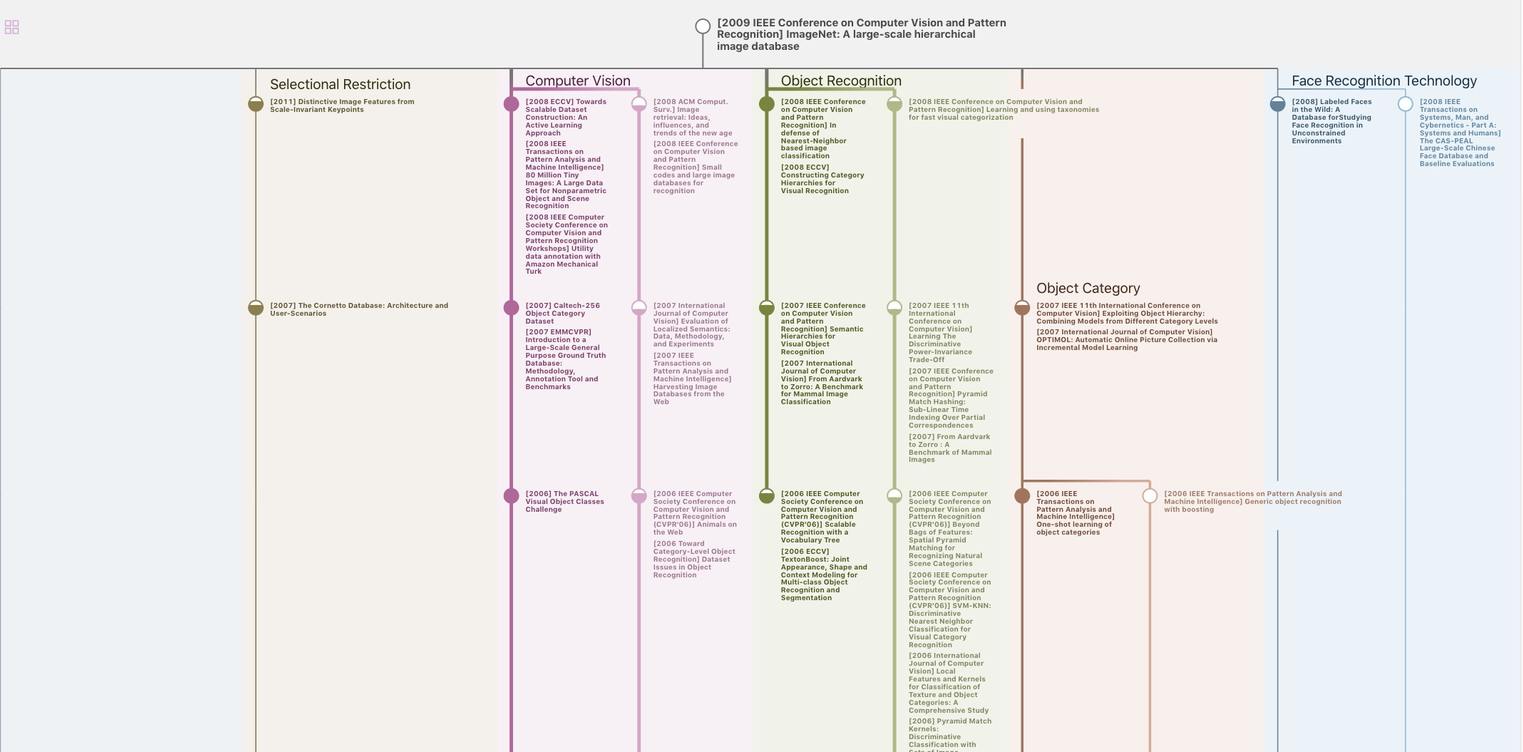
生成溯源树,研究论文发展脉络
Chat Paper
正在生成论文摘要