Anomalib: A Deep Learning Library for Anomaly Detection
2022 IEEE International Conference on Image Processing (ICIP)(2022)
摘要
This paper introduces anomalib 1 , a novel library for unsupervised anomaly detection and localization. With reproducibility and modularity in mind, this open-source library provides algorithms from the literature and a set of tools to design custom anomaly detection algorithms via a plug-and-play approach. Anomalib comprises state-of-the-art anomaly detection algorithms that achieve top performance on the benchmarks and that can be used off-the-shelf. In addition, the library provides components to design custom algorithms that could be tailored towards specific needs. Additional tools, including experiment trackers, visualizers, and hyper-parameter optimizers, make it simple to design and implement anomaly detection models. The library also supports OpenVINO model-optimization and quantization for real-time deployment. Overall, anomalib is an extensive library for the design, implementation, and deployment of unsupervised anomaly detection models from data to the edge.
更多查看译文
关键词
deep learning library,deep learning,detection
AI 理解论文
溯源树
样例
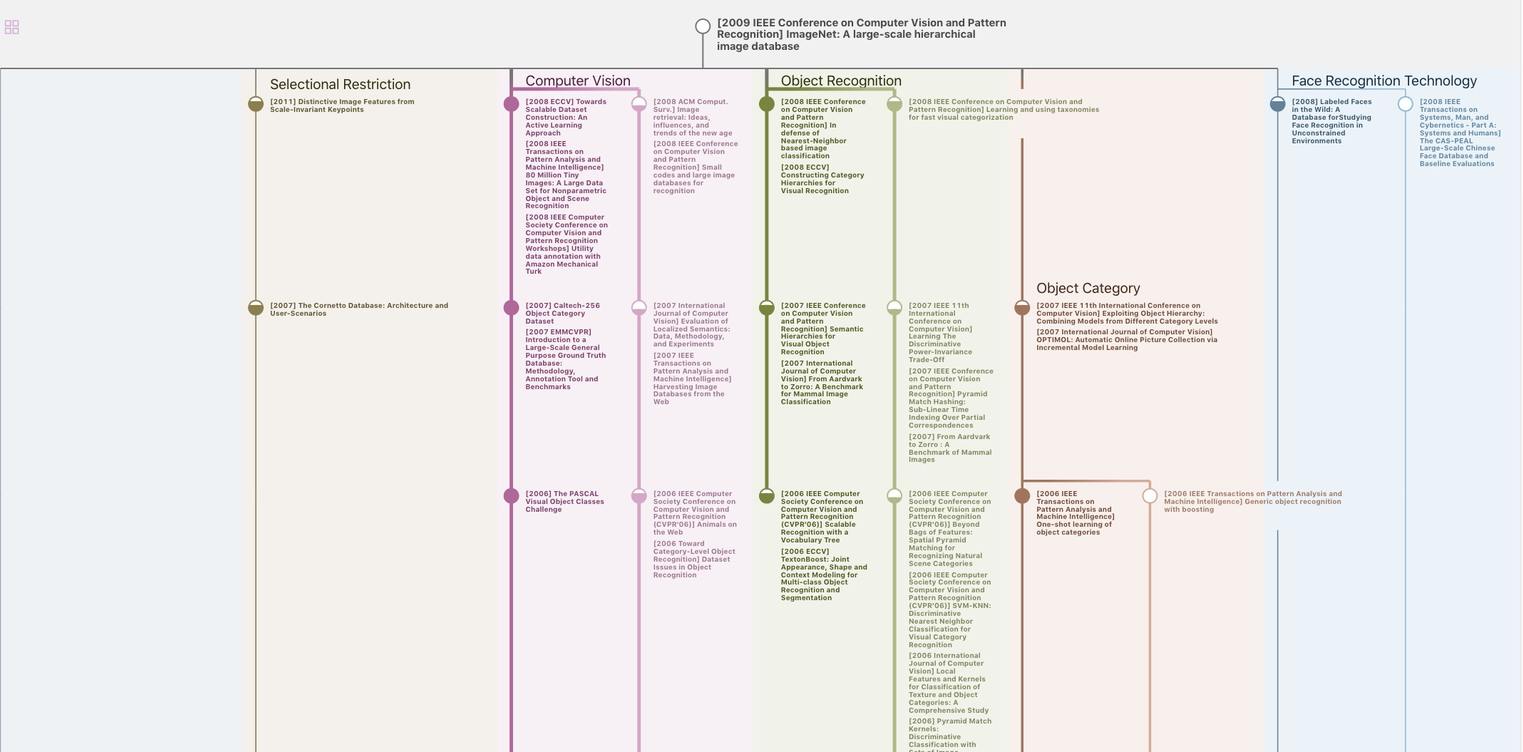
生成溯源树,研究论文发展脉络
Chat Paper
正在生成论文摘要