Efficient Privacy-Preserving ML for IoT: Cluster-Based Split Federated Learning Scheme for Non-IID Data
2023 7th Cyber Security in Networking Conference (CSNet)(2023)
摘要
This paper proposes a scheme addressing the challenges of integrating privacy-preserving distributed machine learning in the Internet of Things (IoT) context while improving the efficiency of the learning process and accelerating the convergence of the model. Specifically, it addresses the presence of non-independent and identically distributed (Non-IID) data and device heterogeneity, which occurs during parallel training and are common characteristics of IoT environments. In this context, SplitFed is a privacy-preserving technique that combines Federated Learning (FL) and Split Learning (SL) to enable fast and privacy-preserving model training on IoT devices. Through parallelism, FL allows fast and parallel model training. Furthermore, SL enables weak devices to train models through model splitting. However, SplitFed suffers from the increasing convergence time due to the FL parallelism introducing Non-IID and stragglers issues. In this paper, we aim to address the aforementioned problems by proposing a clustering parallelization scheme. As such, similar clients are combined based on their weight divergence and considered a single FL unit addressing the Non-IID problem. Moreover, to improve time efficiency and overcome the stragglers' presence, we offer a second clustering stage that separates clients by efficiency and uses FedAsync for model aggregation.
更多查看译文
关键词
Federated Learning,Non-IID,Privacy,Machine Learning,Participants Selection,Split Learning,Clustering
AI 理解论文
溯源树
样例
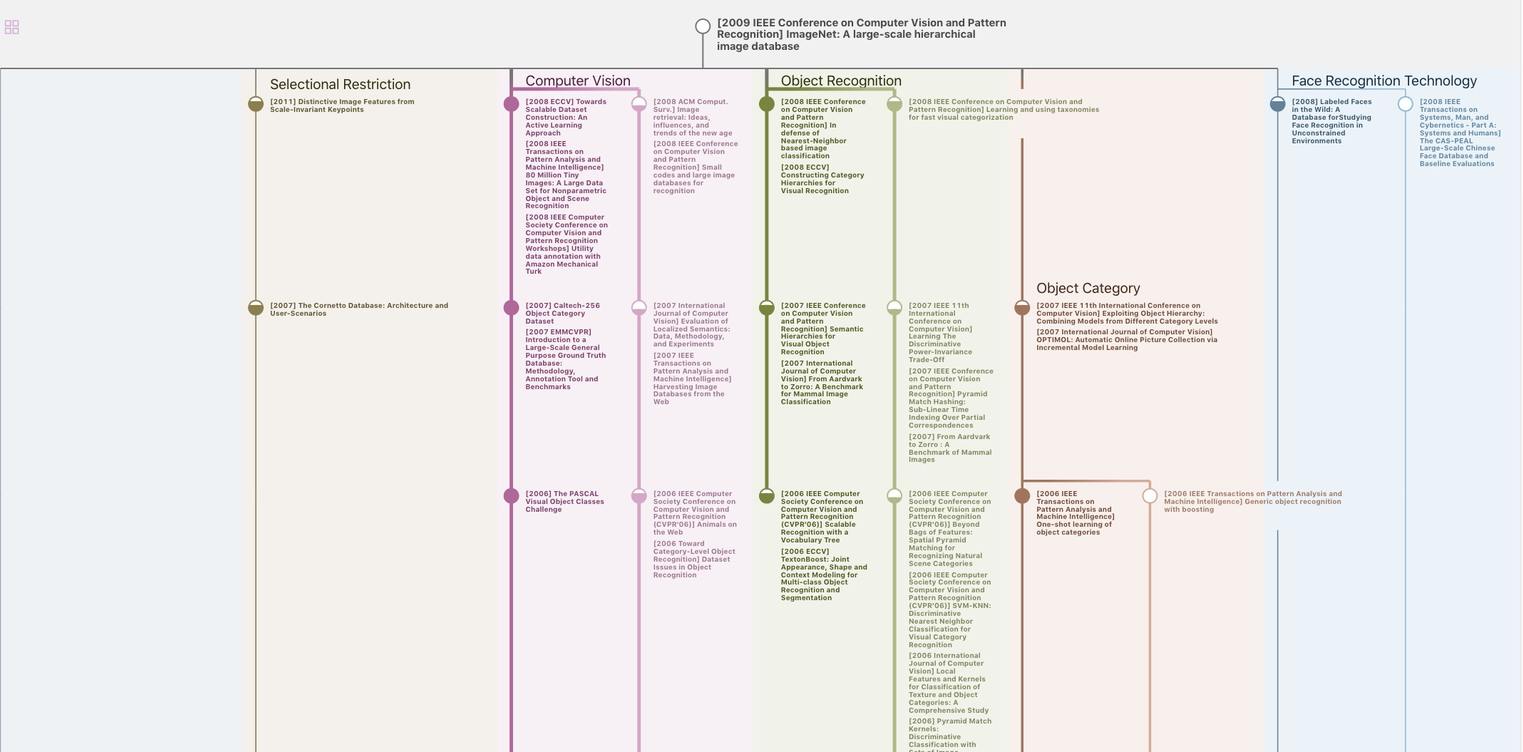
生成溯源树,研究论文发展脉络
Chat Paper
正在生成论文摘要