Predicting Unreliable Predictions by Shattering a Neural Network
arXiv (Cornell University)(2021)
摘要
Piecewise linear neural networks can be split into subfunctions, each with its own activation pattern, domain, and empirical error. Empirical error for the full network can be written as an expectation over empirical error of subfunctions. Constructing a generalization bound on subfunction empirical error indicates that the more densely a subfunction is surrounded by training samples in representation space, the more reliable its predictions are. Further, it suggests that models with fewer activation regions generalize better, and models that abstract knowledge to a greater degree generalize better, all else equal. We propose not only a theoretical framework to reason about subfunction error bounds but also a pragmatic way of approximately evaluating it, which we apply to predicting which samples the network will not successfully generalize to. We test our method on detection of misclassification and out-of-distribution samples, finding that it performs competitively in both cases. In short, some network activation patterns are associated with higher reliability than others, and these can be identified using subfunction error bounds.
更多查看译文
关键词
unreliable predictions,neural network
AI 理解论文
溯源树
样例
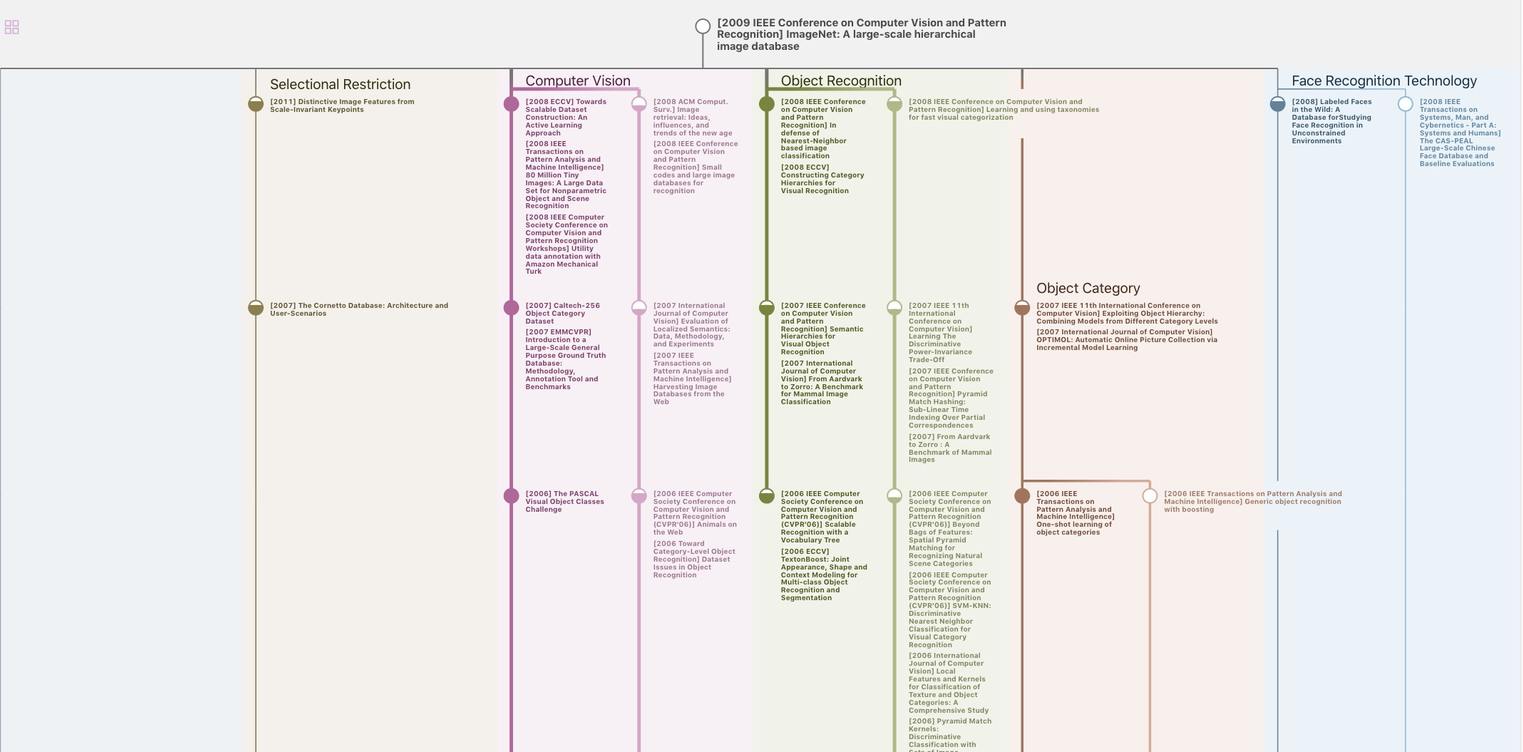
生成溯源树,研究论文发展脉络
Chat Paper
正在生成论文摘要