Multi-Objective Bayesian Optimization over High-Dimensional Search Spaces.
arXiv (Cornell University)(2021)
摘要
The ability to optimize multiple competing objective functions with high sample efficiency is imperative in many applied problems across science and industry. Multi-objective Bayesian optimization (BO) achieves strong empirical performance on such problems, but even with recent methodological advances, it has been restricted to simple, low-dimensional domains. Most existing BO methods exhibit poor performance on search spaces with more than a few dozen parameters. In this work we propose MORBO, a method for multi-objective Bayesian optimization over high-dimensional search spaces. MORBO performs local Bayesian optimization within multiple trust regions simultaneously, allowing it to explore and identify diverse solutions even when the objective functions are difficult to model globally. We show that MORBO significantly advances the state-of-the-art in sample-efficiency for several high-dimensional synthetic and real-world multi-objective problems, including a vehicle design problem with 222 parameters, demonstrating that MORBO is a practical approach for challenging and important problems that were previously out of reach for BO methods.
更多查看译文
关键词
optimization,search,multi-objective,high-dimensional
AI 理解论文
溯源树
样例
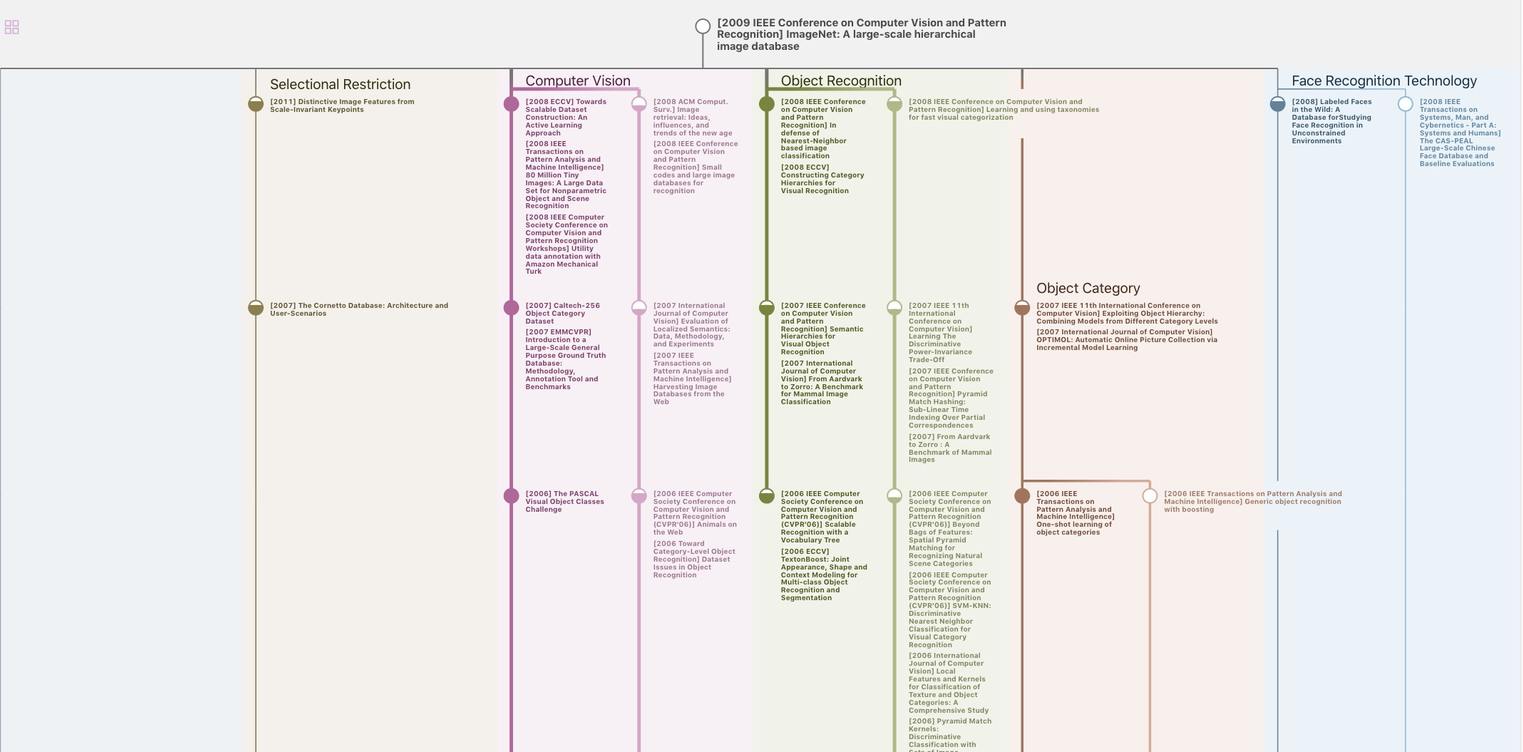
生成溯源树,研究论文发展脉络
Chat Paper
正在生成论文摘要