WALNUT: A Benchmark on Weakly Supervised Learning for Natural Language Understanding.
arXiv (Cornell University)(2021)
摘要
Building quality machine learning models for natural language understanding (NLU) tasks relies heavily on labeled data. Weak supervision has been shown to provide valuable supervision when large amount of labeled data is unavailable or expensive to obtain. Existing works studying weak supervision for NLU either mostly focus on a specific task or simulate weak supervision signals from ground-truth labels. To date a benchmark for NLU with real world weak supervision signals for a collection of NLU tasks is still not available. In this paper, we propose such a benchmark, named WALNUT, to advocate and facilitate research on weak supervision for NLU. WALNUT consists of NLU tasks with different types, including both document-level prediction tasks and token-level prediction tasks and for each task contains weak labels generated by multiple real-world weak sources. We conduct baseline evaluations on the benchmark to systematically test the value of weak supervision for NLU tasks, with various weak supervision methods and model architectures. We demonstrate the benefits of weak supervision for low-resource NLU tasks and expect WALNUT to stimulate further research on methodologies to best leverage weak supervision. The benchmark and code for baselines will be publicly available at this http URL.
更多查看译文
关键词
weakly supervised learning,natural language understanding,walnut,benchmark
AI 理解论文
溯源树
样例
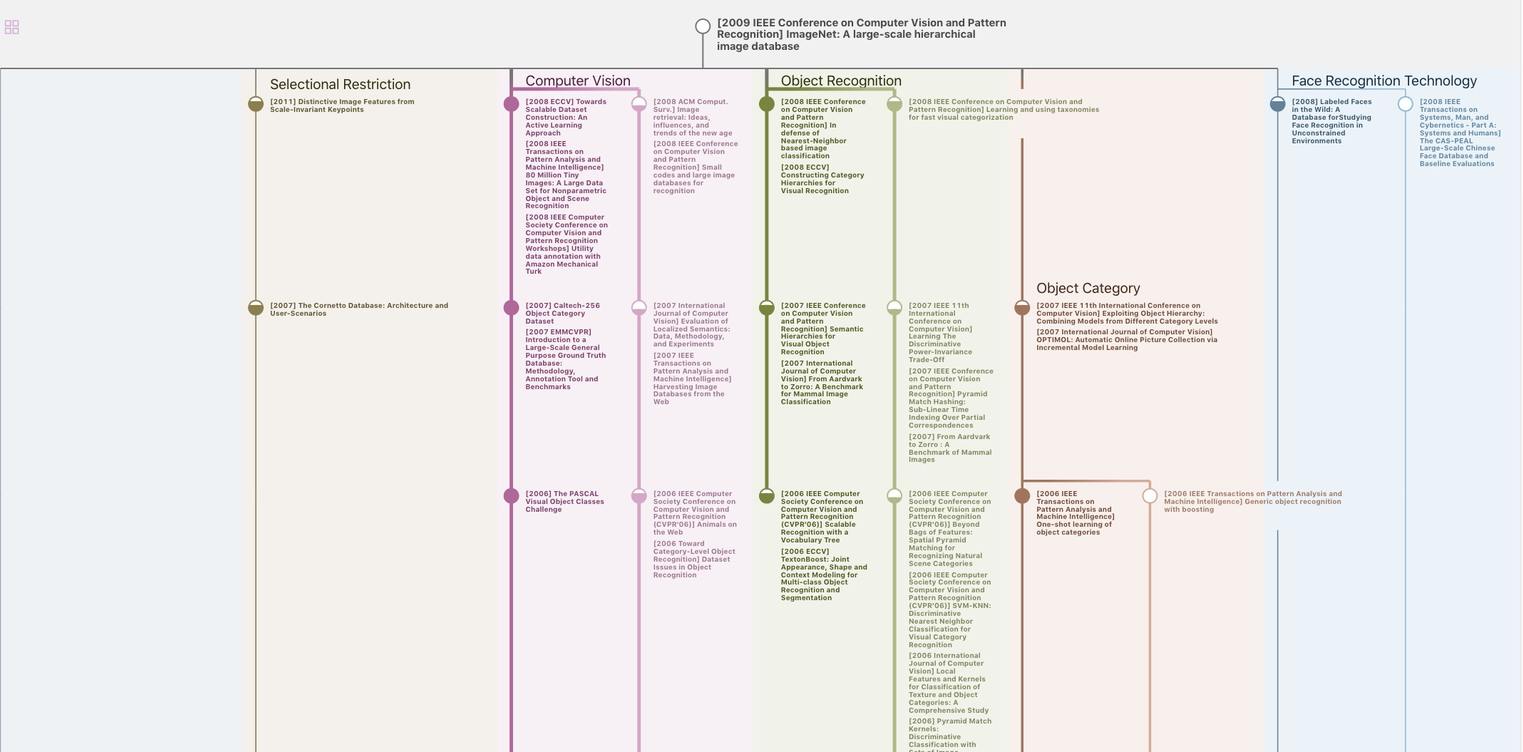
生成溯源树,研究论文发展脉络
Chat Paper
正在生成论文摘要