Robot Sound Interpretation: Learning Visual-Audio Representations for Voice-Controlled Robots.
arXiv (Cornell University)(2021)
摘要
Inspired by sensorimotor theory, we propose a novel pipeline for voice-controlled robots. Previous work relies on explicit labels of sounds and images as well as extrinsic reward functions. Not only do such approaches have little resemblance to human sensorimotor development, but also require hand-tuning rewards and extensive human labor. To address these problems, we learn a representation that associates images and sound commands with minimal supervision. Using this representation, we generate an intrinsic reward function to learn robotic tasks with reinforcement learning. We demonstrate our approach on three robot platforms, a TurtleBot3, a Kuka-IIWA arm, and a Kinova Gen3 robot, which hear a command word, identify the associated target object, and perform precise control to approach the target. We show that our method outperforms previous work across various sound types and robotic tasks empirically. We successfully deploy the policy learned in simulator to a real-world Kinova Gen3.
更多查看译文
关键词
robot sound interpretation,robots,visual-audio,voice-controlled
AI 理解论文
溯源树
样例
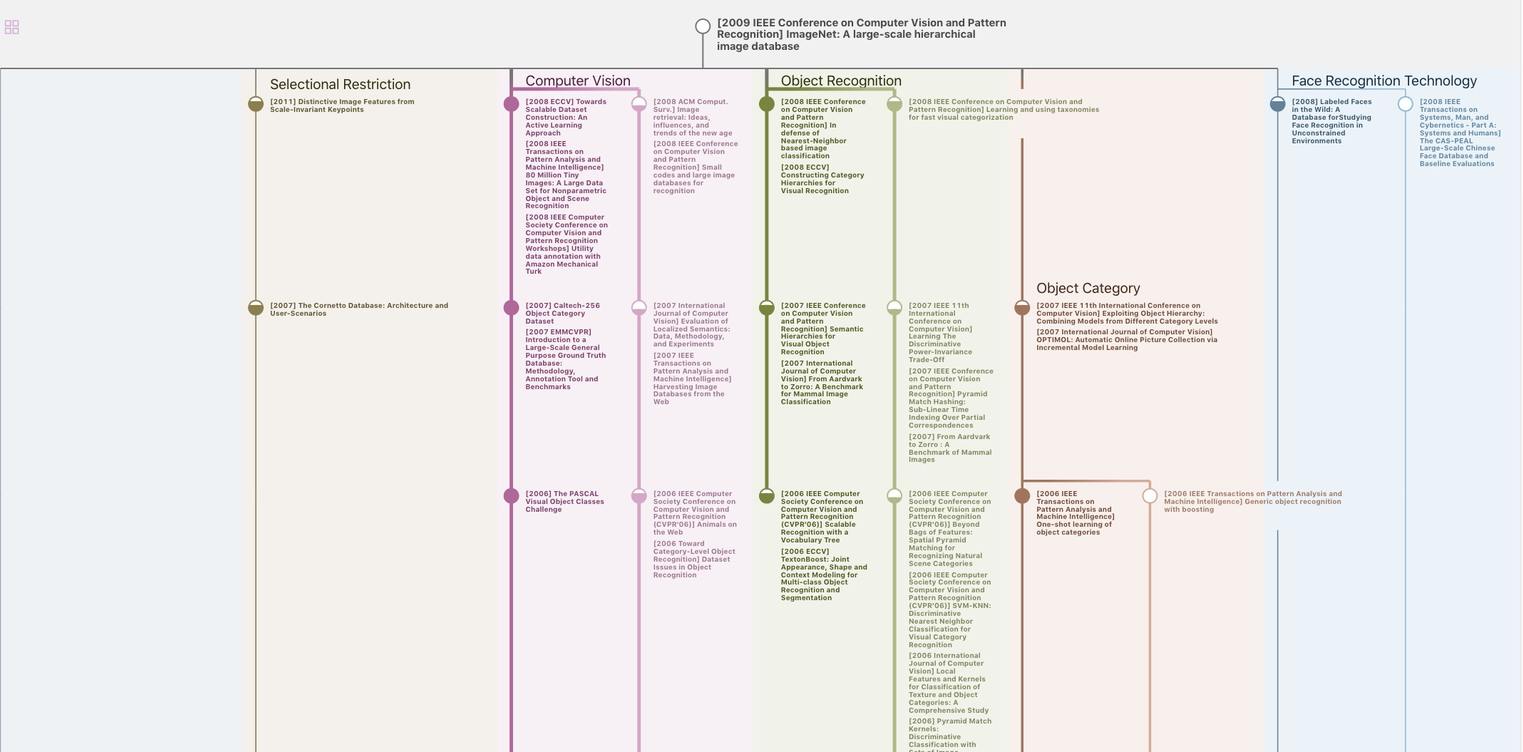
生成溯源树,研究论文发展脉络
Chat Paper
正在生成论文摘要