ОЦЕНКА ВЛИЯНИЯ РАЗЛИЧНЫХ НЕАРХИТЕКТУРНЫХ ИЗМЕНЕНИЙ ПРЕДСКАЗАТЕЛЬНОЙ МОДЕЛИ НА КАЧЕСТВО КЛАССИФИКАЦИИ ЭКГ
Труды Института системного программирования РАН(2021)
摘要
Recording and analyzing 12-lead electrocardiograms is the most common procedure for detecting heart disease. Recently, various deep learning methods have been proposed for the automatic diagnosis by an electrocardiogram. The proposed methods can provide a second opinion for the doctor and help detect pathologies at an early stage. Various methods are proposed in the paper to improve the quality of prediction of ECG recording pathologies. Techniques include adding patient metadata, ECG noise reduction, and self-adaptive learning. The significance of data parameters in training a classification model is also explored. Among the considered parameters, the influence of various ECG leads, the length of the electrocardiogram and the volume of the training sample is studied. The experiments carried out show the relevance of the described approaches and offer an optimal estimate of the input data parameters.
更多查看译文
关键词
различных неархитектурных изменений,модели,на
AI 理解论文
溯源树
样例
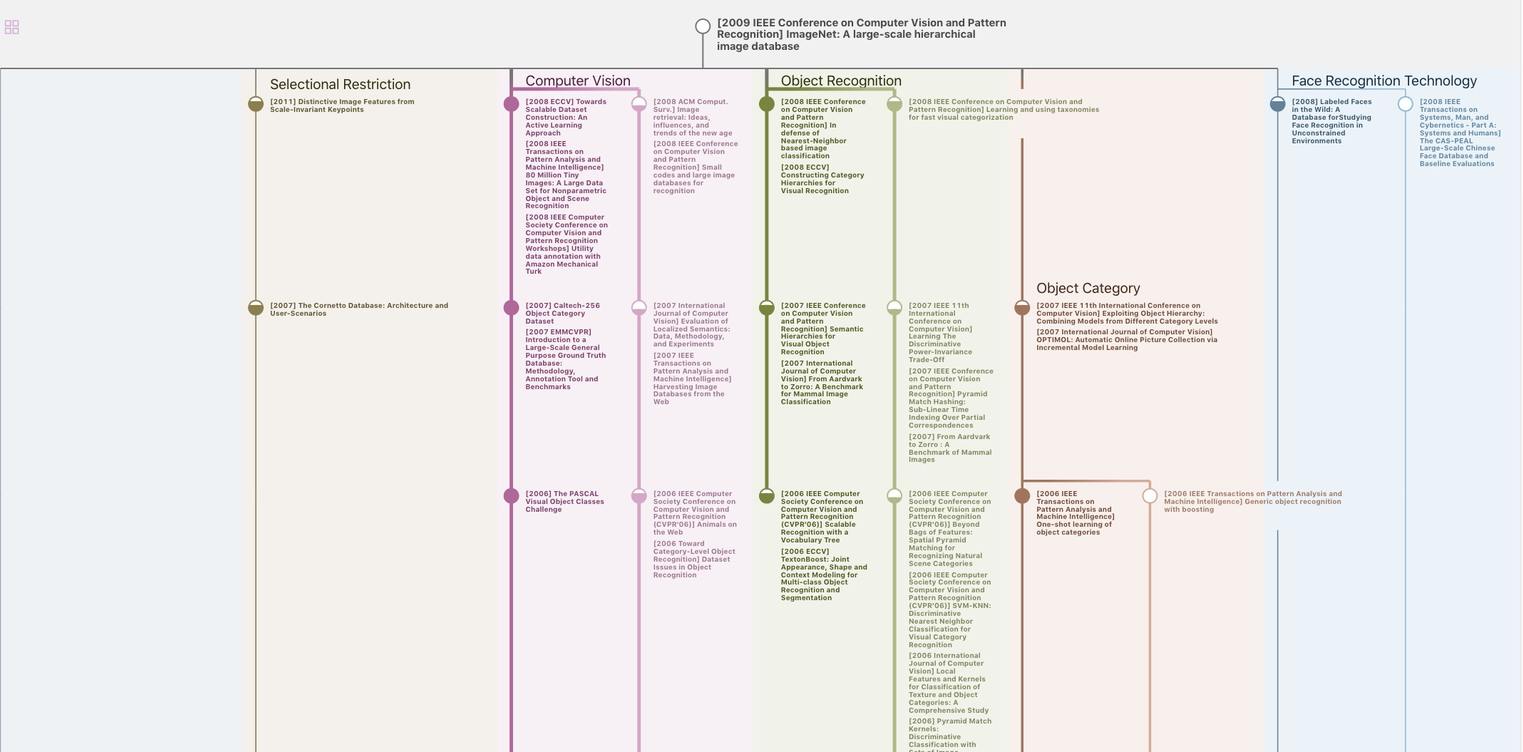
生成溯源树,研究论文发展脉络
Chat Paper
正在生成论文摘要