Logarithmic Unbiased Quantization: Simple 4-bit Training in Deep Learning
arXiv (Cornell University)(2021)
摘要
Quantization of the weights and activations is one of the main methods to reduce the computational footprint of Deep Neural Networks (DNNs) training. Current methods enable 4-bit quantization of the forward phase. However, this constitutes only a third of the training process. Reducing the computational footprint of the entire training process requires the quantization of the neural gradients, i.e., the loss gradients with respect to the outputs of intermediate neural layers. In this work, we examine the importance of having unbiased quantization in quantized neural network training, where to maintain it, and how. Based on this, we suggest a \textit{logarithmic unbiased quantization} (LUQ) method to quantize all both the forward and backward phase to 4-bit, achieving state-of-the-art results in 4-bit training without overhead. For example, in ResNet50 on ImageNet, we achieved a degradation of 1.1\%. We further improve this to degradation of only 0.32\% after three epochs of high precision fine-tunining, combined with a variance reduction method -- where both these methods add overhead comparable to previously suggested methods.
更多查看译文
关键词
learning,deep
AI 理解论文
溯源树
样例
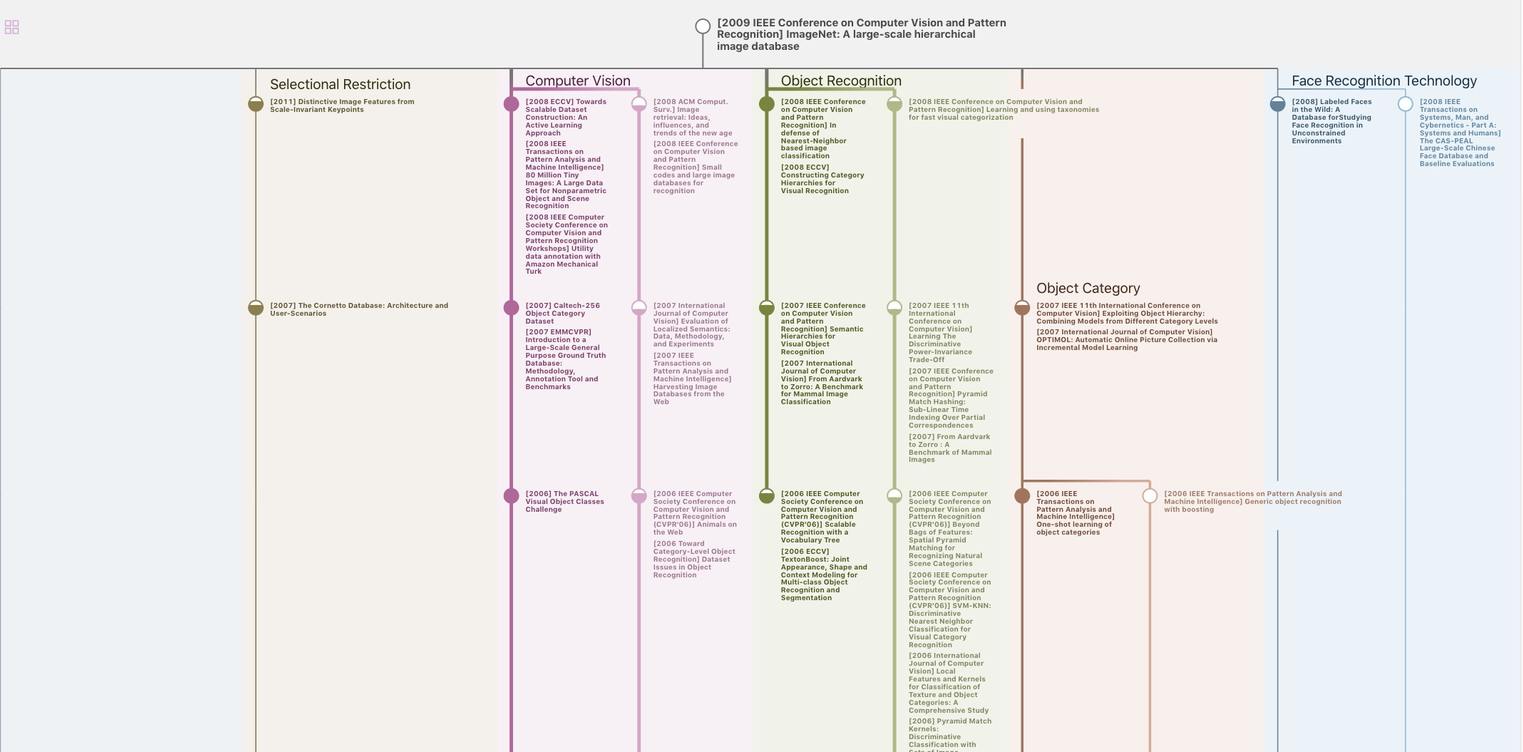
生成溯源树,研究论文发展脉络
Chat Paper
正在生成论文摘要