Cyber attacker's next action prediction on dynamic real-time behavior model
COMPUTERS & ELECTRICAL ENGINEERING(2024)
摘要
This paper addresses the critical need for enhanced threat analysis amid escalating cyber threats. Recognizing limitations in current network-level Attack Graphs (AGs), including scalability issues and the challenge of detecting zero-day attacks, we introduce a two-phase approach. Firstly, we dynamically model the attacker's real-time behavior within the target system, resulting in a minimal AG with action states and their system impact. In the second phase, a Hidden Markov Model (HMM) predicts the next action based on the real-time behavior model, refined through Honeypot data for continuous accuracy. The minimal AG not only predicts but also forecasts the impact of the next action, facilitating robust security decisions. Experimental evaluations using Cowrie Honeypot logs demonstrate its efficacy, outperforming conventional algorithms. This innovative approach significantly advances cyber threat analysis, enhancing security decision-making within a concise framework.
更多查看译文
关键词
Attacker behavior modeling,Action prediction,Hidden Markov Model,Host-based cyber attack,Honeypot,Real-time monitoring,Real-time prediction
AI 理解论文
溯源树
样例
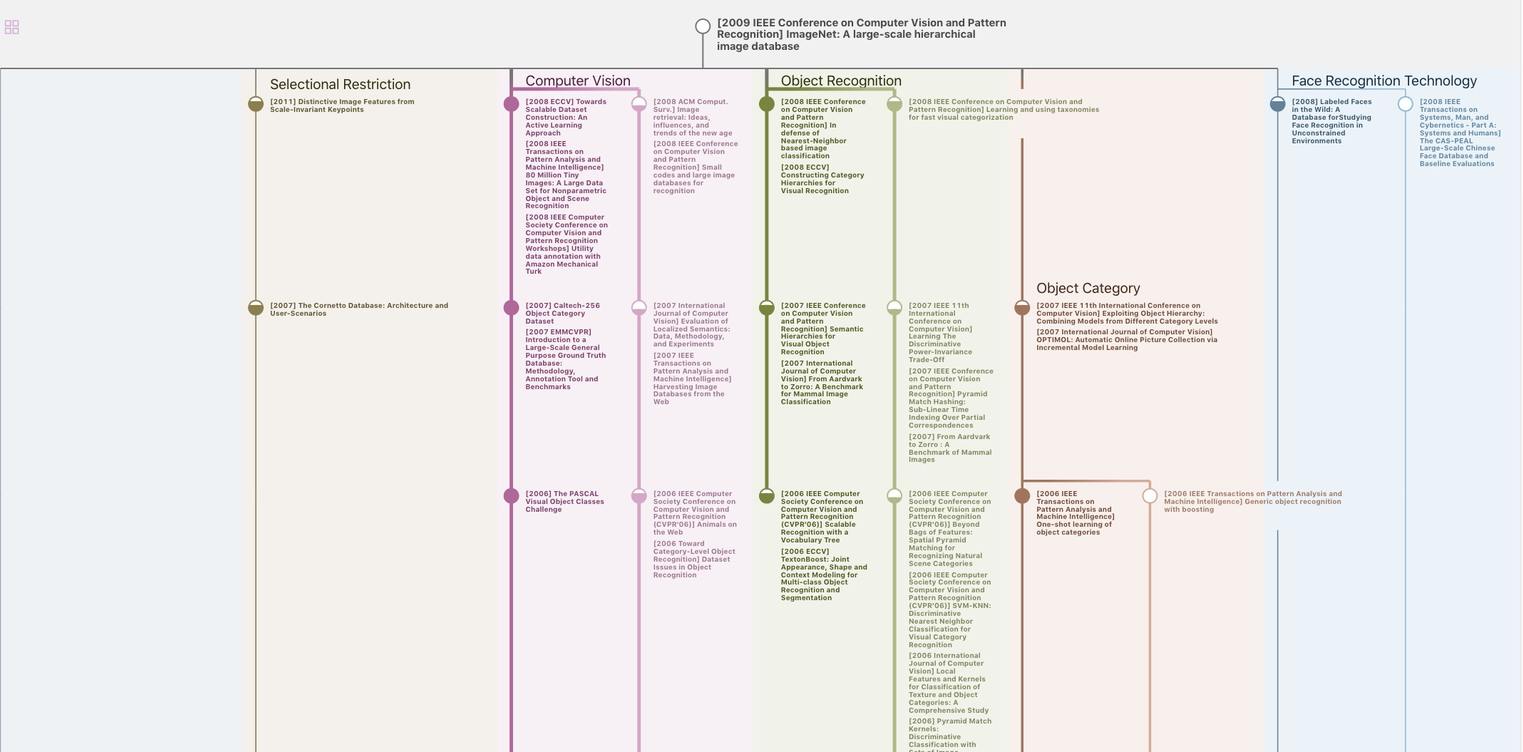
生成溯源树,研究论文发展脉络
Chat Paper
正在生成论文摘要