POLAR: A Polynomial Arithmetic Framework for Verifying Neural-Network Controlled Systems
arXiv (Cornell University)(2021)
摘要
We present POLAR, a polynomial arithmetic-based framework for efficient bounded-time reachability analysis of neural-network controlled systems (NNCSs). Existing approaches that leverage the standard Taylor Model (TM) arithmetic for approximating the neural-network controller cannot deal with non-differentiable activation functions and suffer from rapid explosion of the remainder when propagating the TMs. POLAR overcomes these shortcomings by integrating TM arithmetic with \textbf{Bernstein B{\'e}zier Form} and \textbf{symbolic remainder}. The former enables TM propagation across non-differentiable activation functions and local refinement of TMs, and the latter reduces error accumulation in the TM remainder for linear mappings in the network. Experimental results show that POLAR significantly outperforms the current state-of-the-art tools in terms of both efficiency and tightness of the reachable set overapproximation. The source code can be found in https://github.com/ChaoHuang2018/POLAR_Tool
更多查看译文
关键词
polynomial arithmetic framework,controlled systems,neural-network
neural-network
AI 理解论文
溯源树
样例
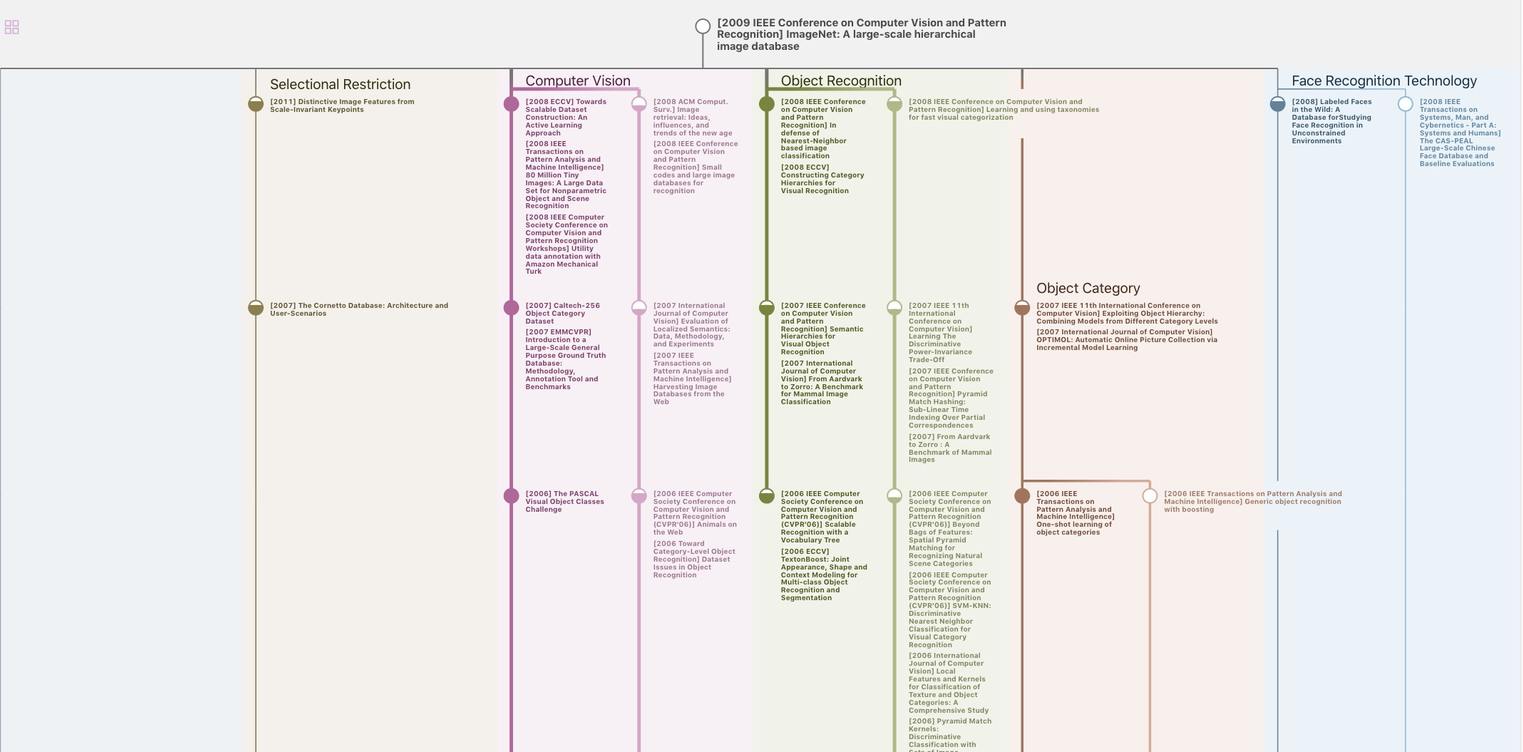
生成溯源树,研究论文发展脉络
Chat Paper
正在生成论文摘要