Accurate Visual-Inertial SLAM by Feature Re-identification
arXiv (Cornell University)(2021)
摘要
We propose a novel feature re-identification method for real-time visual-inertial SLAM. The front-end module of the state-of-the-art visual-inertial SLAM methods (e.g. visual feature extraction and matching schemes) relies on feature tracks across image frames, which are easily broken in challenging scenarios, resulting in insufficient visual measurement and accumulated error in pose estimation. In this paper, we propose an efficient drift-less SLAM method by re-identifying existing features from a spatial-temporal sensitive sub-global map. The re-identified features over a long time span serve as augmented visual measurements and are incorporated into the optimization module which can gradually decrease the accumulative error in the long run, and further build a drift-less global map in the system. Extensive experiments show that our feature re-identification method is both effective and efficient. Specifically, when combining the feature re-identification with the state-of-the-art SLAM method [11], our method achieves 67.3% and 87.5% absolute translation error reduction with only a small additional computational cost on two public SLAM benchmark DBs: EuRoC and TUM-VI respectively.
更多查看译文
关键词
visual-inertial,re-identification
AI 理解论文
溯源树
样例
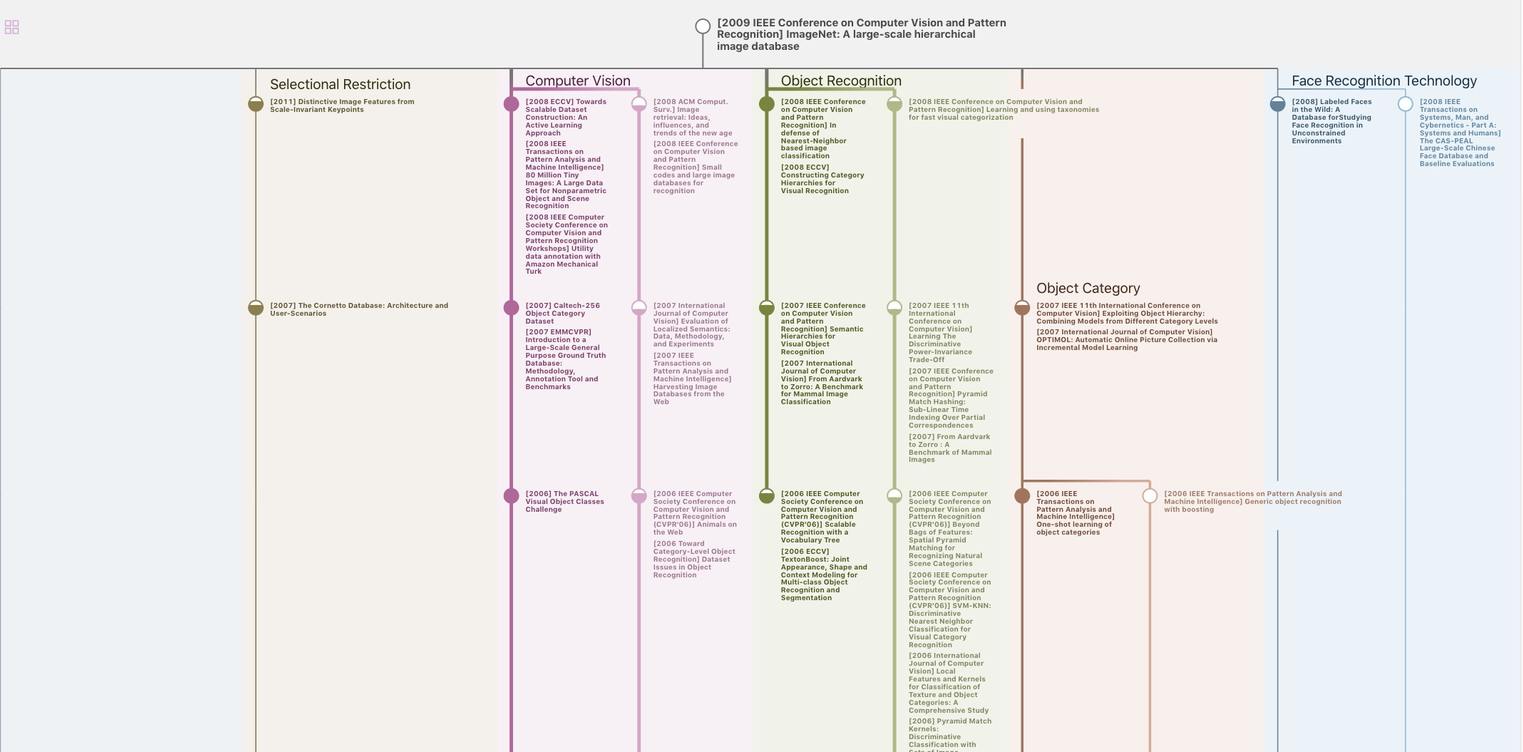
生成溯源树,研究论文发展脉络
Chat Paper
正在生成论文摘要