Sharing in a Trustless World: Privacy-Preserving Data Analytics with Potentially Cheating Participants
arXiv (Cornell University)(2021)
摘要
Lack of trust between organisations and privacy concerns about their data are impediments to an otherwise potentially symbiotic joint data analysis. We propose DataRing, a data sharing system that allows mutually mistrusting participants to query each others' datasets in a privacy-preserving manner while ensuring the correctness of input datasets and query answers even in the presence of (cheating) participants deviating from their true datasets. By relying on the assumption that if only a small subset of rows of the true dataset are known, participants cannot submit answers to queries deviating significantly from their true datasets. We employ differential privacy and a suite of cryptographic tools to ensure individual privacy for each participant's dataset and data confidentiality from the system. Our results show that the evaluation of 10 queries on a dataset with 10 attributes and 500,000 records is achieved in 90.63 seconds. DataRing could detect cheating participant that deviates from its true dataset in few queries with high accuracy.
更多查看译文
关键词
trustless world,sharing,data,privacy-preserving
AI 理解论文
溯源树
样例
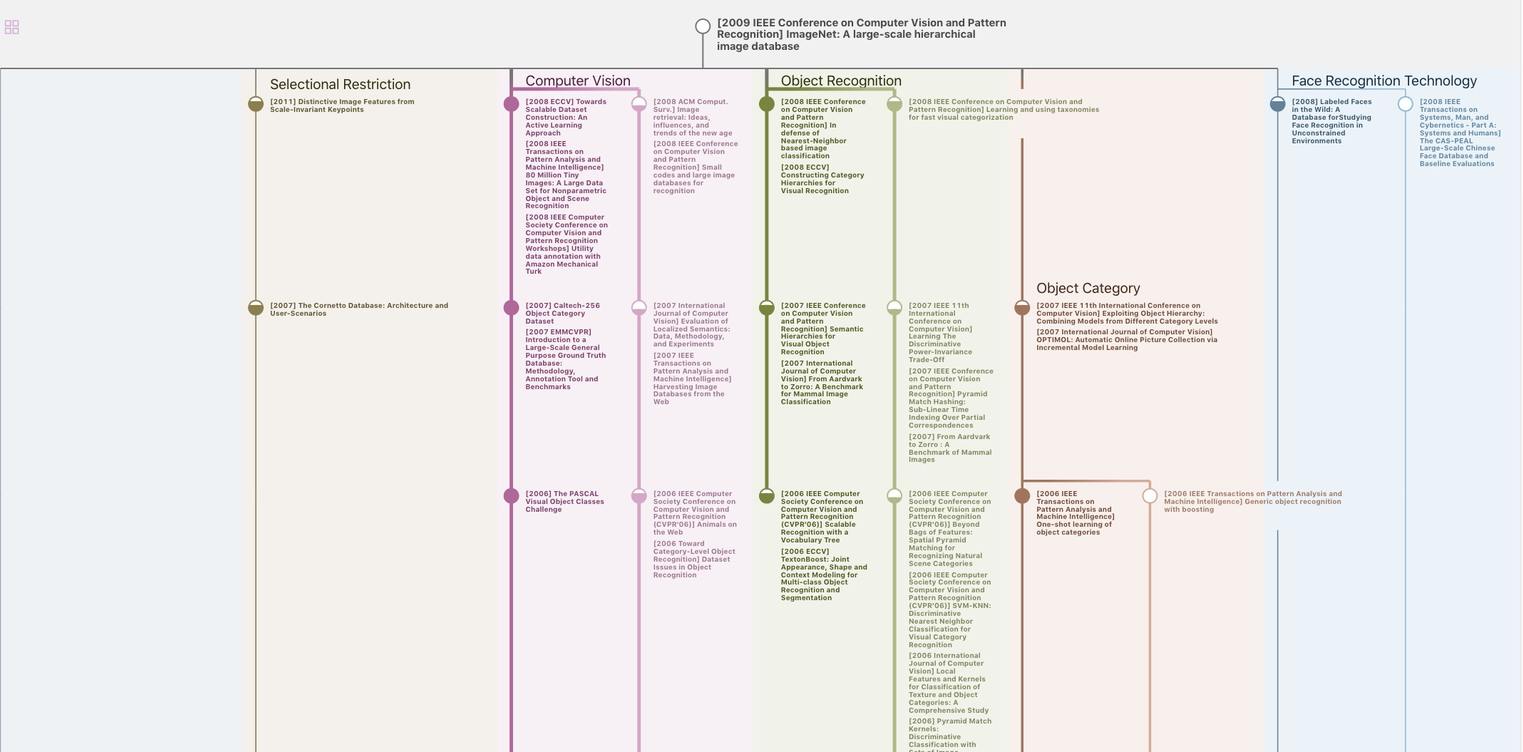
生成溯源树,研究论文发展脉络
Chat Paper
正在生成论文摘要