Cyber Intrusion Detection by Using Deep Neural Networks with Attack-sharing Loss
arXiv (Cornell University)(2021)
摘要
Cyber attacks pose crucial threats to computer system security, and put digital treasuries at excessive risks. This leads to an urgent call for an effective intrusion detection system that can identify the intrusion attacks with high accuracy. It is challenging to classify the intrusion events due to the wide variety of attacks. Furthermore, in a normal network environment, a majority of the connections are initiated by benign behaviors. The class imbalance issue in intrusion detection forces the classifier to be biased toward the majority/benign class, thus leave many attack incidents undetected. Spurred by the success of deep neural networks in computer vision and natural language processing, in this paper, we design a new system named DeepIDEA that takes full advantage of deep learning to enable intrusion detection and classification. To achieve high detection accuracy on imbalanced data, we design a novel attack-sharing loss function that can effectively move the decision boundary towards the attack classes and eliminates the bias towards the majority/benign class. By using this loss function, DeepIDEA respects the fact that the intrusion mis-classification should receive higher penalty than the attack mis-classification. Extensive experimental results on three benchmark datasets demonstrate the high detection accuracy of DeepIDEA. In particular, compared with eight state-of-the-art approaches, DeepIDEA always provides the best class-balanced accuracy.
更多查看译文
关键词
deep neural networks,attack-sharing
AI 理解论文
溯源树
样例
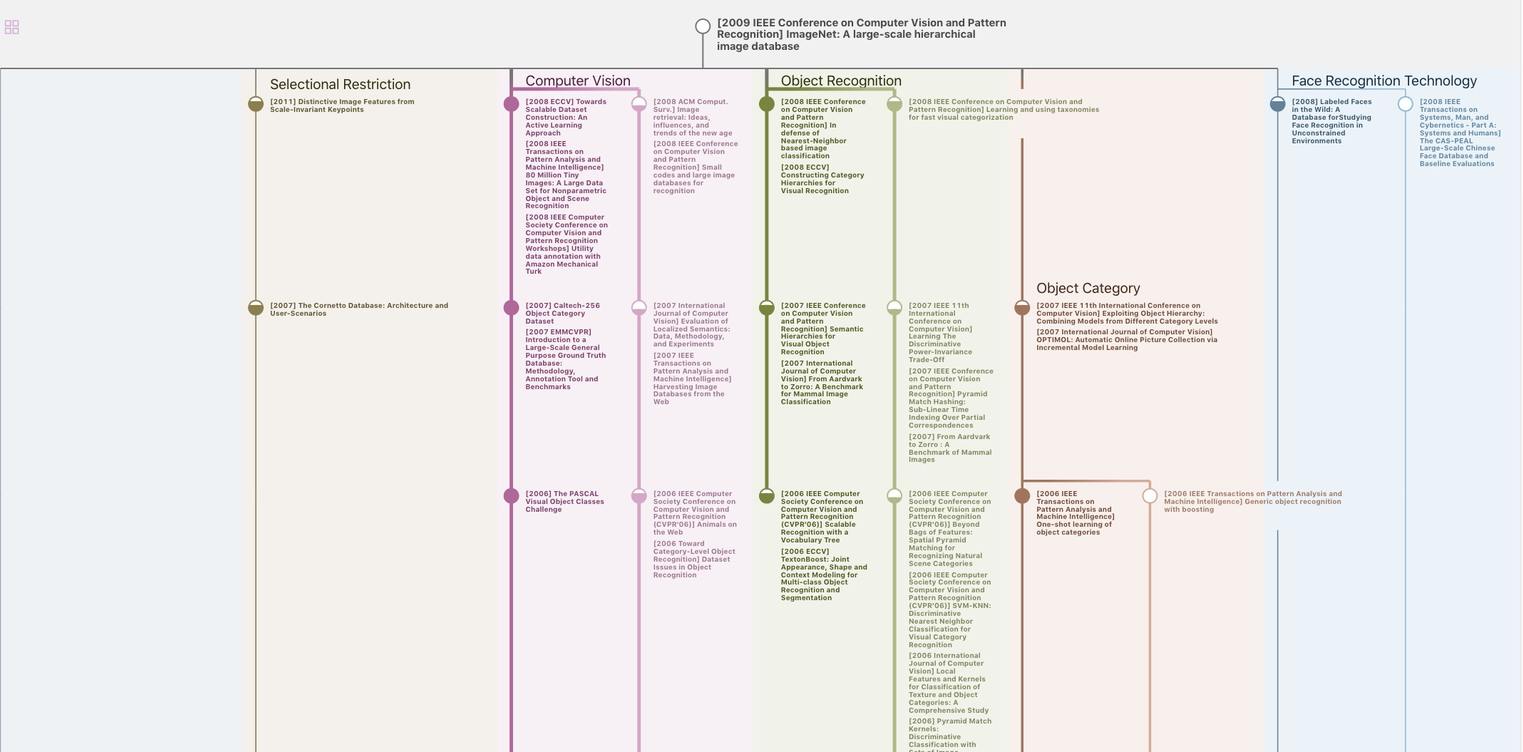
生成溯源树,研究论文发展脉络
Chat Paper
正在生成论文摘要