Cross-modal Semantically Augmented Network for Image-text Matching
ACM TRANSACTIONS ON MULTIMEDIA COMPUTING COMMUNICATIONS AND APPLICATIONS(2024)
摘要
Image-text matching plays an important role in solving the problem of cross-modal information processing. Since there are nonnegligible semantic differences between heterogenous pairwise data, a crucial challenge is how to learn a unified representation. Existing methods mainly rely on the alignment between regional image features and corresponding entity words. However, the regional features in the image are often more concerned with the foreground entity information, and the attribute information of the entities and the relational information are ignored. How to effectively integrate entity-attribute alignment and relationship alignment has not been fully studied. Therefore, we propose a Cross-Modal Semantically Augmented Network for Image-Text Matching (CMSAN), which combines the relationships between entities in the image with the semantics of relational words in the text. CMSAN (1) proposes an adaptive word-type prediction model that classifies the words into four types, i.e., entity word, attribute word, relation word, and unnecessary word. It can align different image features at multiple levels. CMSAN (2) designs a sophisticated relationship alignment module and an entity-attribute alignment module that maximizes the exploitation of the semantic information, which enables the model to have more discriminative power and further improves the matching accuracy.
更多查看译文
关键词
Image-text matching,cross-modal semantically augmented,adaptive word-type prediction model,relationship alignment
AI 理解论文
溯源树
样例
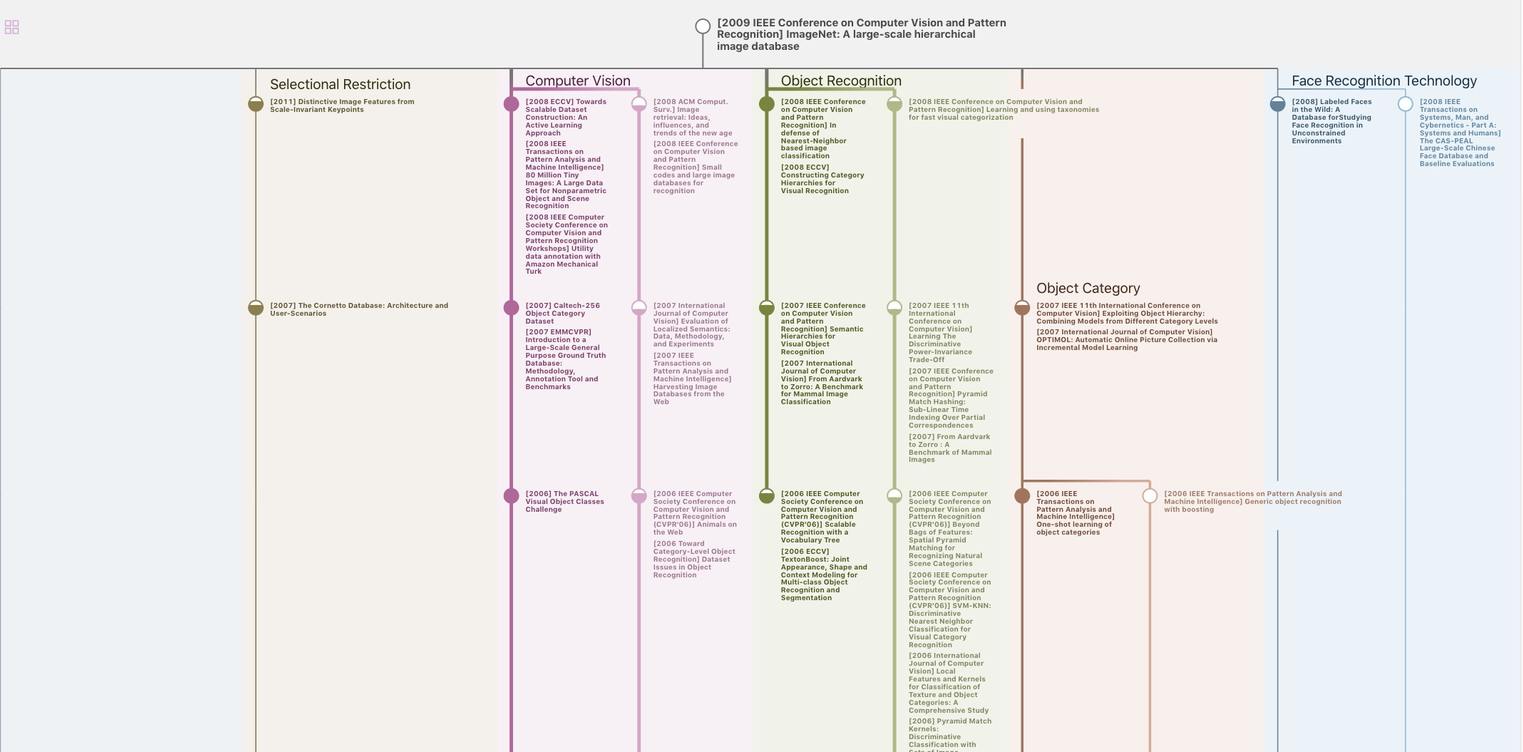
生成溯源树,研究论文发展脉络
Chat Paper
正在生成论文摘要