Benchmarking saliency methods for chest X-ray interpretation
medRxiv (Cold Spring Harbor Laboratory)(2021)
摘要
Abstract Saliency methods, which “explain” deep neural networks by producing heat maps that highlight the areas of the medical image that influence model prediction, are often presented to clinicians as an aid in diagnostic decision-making. Although many saliency methods have been proposed for medical imaging interpretation, rigorous investigation of the accuracy and reliability of these strategies is necessary before they are integrated into the clinical setting. In this work, we quantitatively evaluate seven saliency methods—including Grad-CAM, Grad-CAM++, and Integrated Gradients—across multiple neural network architectures using two evaluation metrics. We establish the first human benchmark for chest X-ray segmentation in a multilabel classification set up, and examine under what clinical conditions saliency maps might be more prone to failure in localizing important pathologies compared to a human expert benchmark. We find that (i) while Grad-CAM generally localized pathologies better than the other evaluated saliency methods, all seven performed significantly worse compared with the human benchmark; (ii) the gap in localization performance between Grad-CAM and the human benchmark was largest for pathologies that were smaller in size and had shapes that were more complex; (iii) model confidence was positively correlated with Grad-CAM localization performance. While it is difficult to know whether poor localization performance is attributable to the model or to the saliency method, our work demonstrates that several important limitations of saliency methods must be addressed before we can rely on them for deep learning explainability in medical imaging.
更多查看译文
关键词
saliency methods,chest,x-ray
AI 理解论文
溯源树
样例
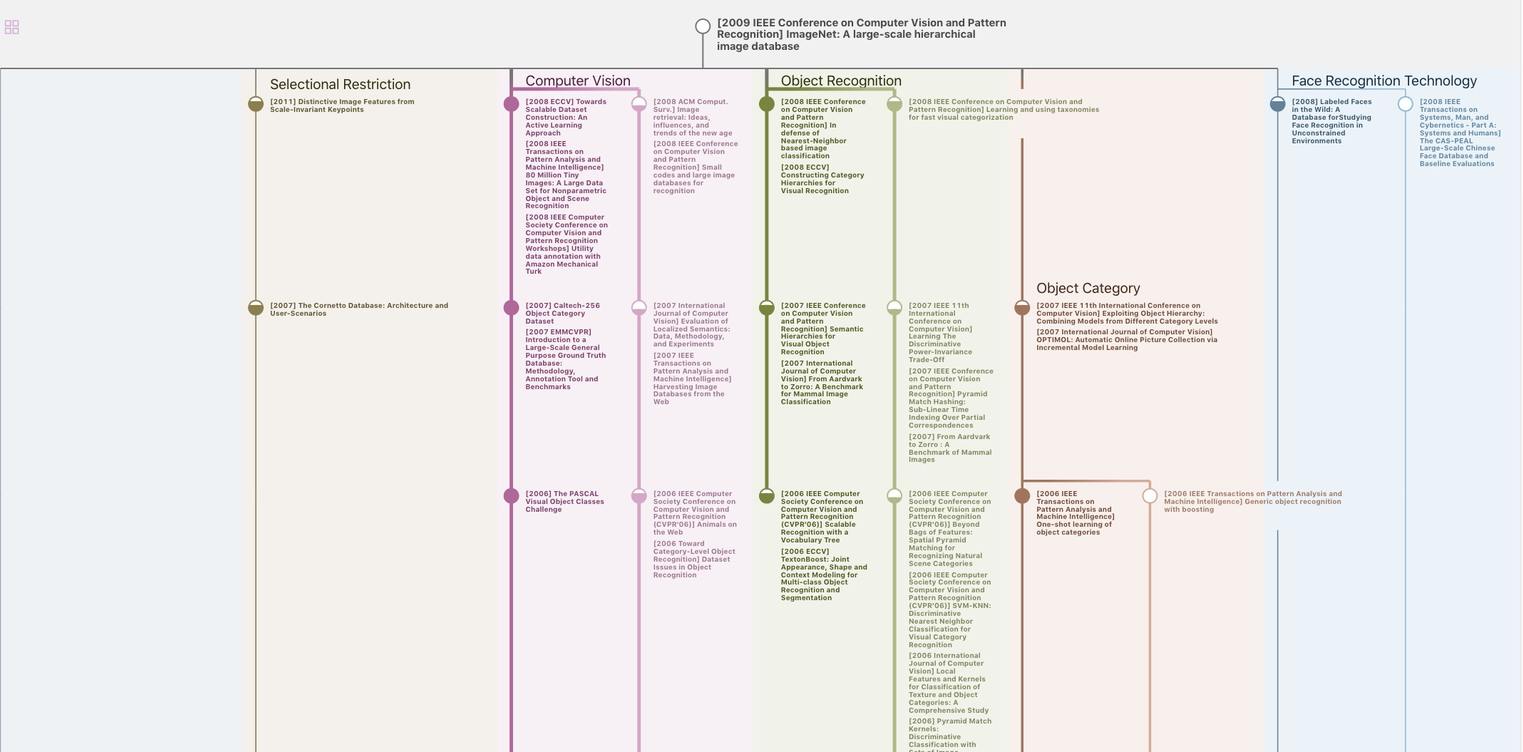
生成溯源树,研究论文发展脉络
Chat Paper
正在生成论文摘要