SVT-Net: Super Light-Weight Sparse Voxel Transformer for Large Scale Place Recognition
arXiv (Cornell University)(2021)
摘要
Point cloud-based large scale place recognition is fundamental for many applications like Simultaneous Localization and Mapping (SLAM). Although many models have been proposed and have achieved good performance by learning short-range local features, long-range contextual properties have often been neglected. Moreover, the model size has also become a bottleneck for their wide applications. To overcome these challenges, we propose a super light-weight network model termed SVT-Net for large scale place recognition. Specifically, on top of the highly efficient 3D Sparse Convolution (SP-Conv), an Atom-based Sparse Voxel Transformer (ASVT) and a Cluster-based Sparse Voxel Transformer (CSVT) are proposed to learn both short-range local features and long-range contextual features in this model. Consisting of ASVT and CSVT, SVT-Net can achieve state-of-the-art on benchmark datasets in terms of both accuracy and speed with a super-light model size (0.9M). Meanwhile, two simplified versions of SVT-Net are introduced, which also achieve state-of-the-art and further reduce the model size to 0.8M and 0.4M respectively.
更多查看译文
关键词
transformer,recognition,place,svt-net,light-weight
AI 理解论文
溯源树
样例
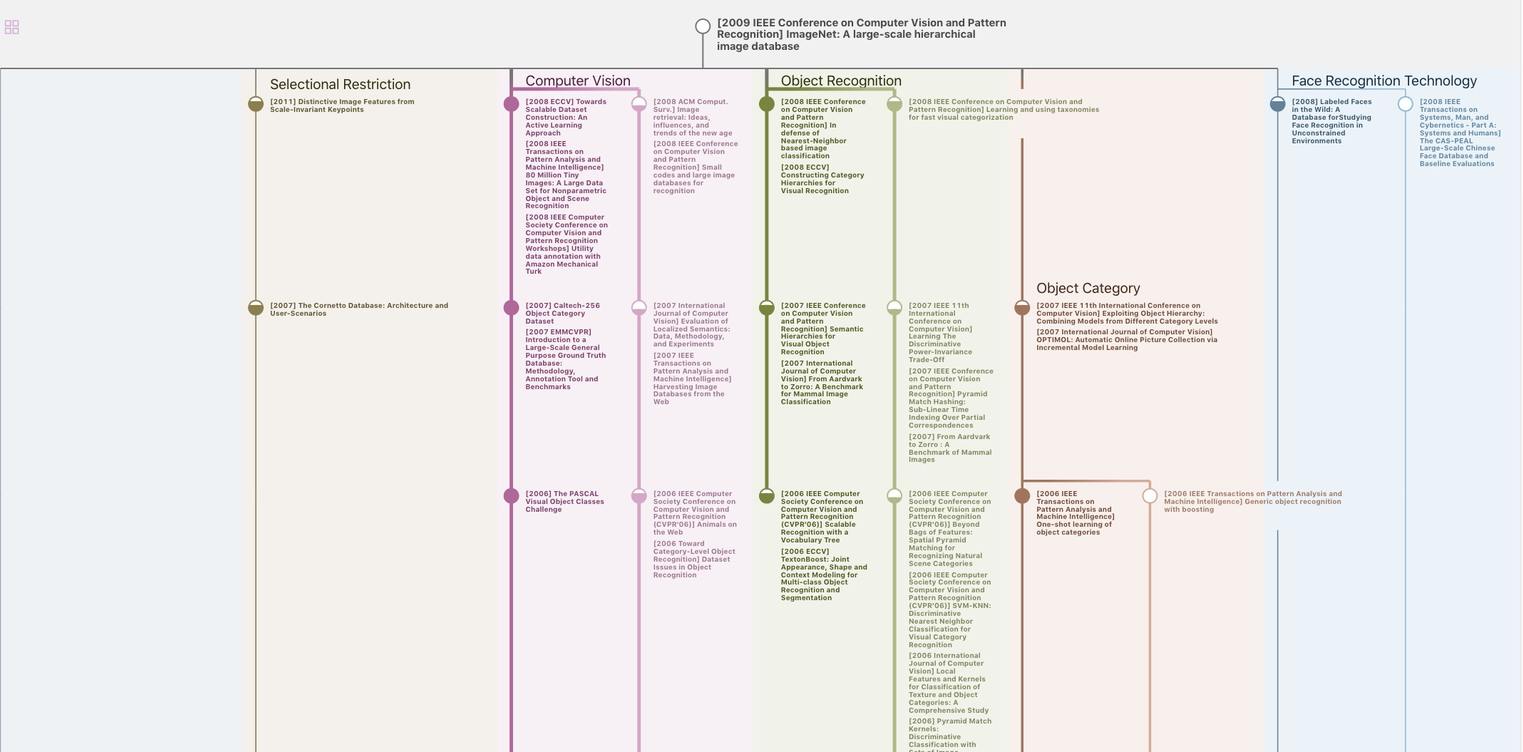
生成溯源树,研究论文发展脉络
Chat Paper
正在生成论文摘要