Crime Prediction with Graph Neural Networks and Multivariate Normal Distributions
arXiv (Cornell University)(2021)
摘要
Existing approaches to the crime prediction problem are unsuccessful in expressing the details since they assign the probability values to large regions. This paper introduces a new architecture with the graph convolutional networks (GCN) and multivariate Gaussian distributions to perform high-resolution forecasting that applies to any spatiotemporal data. We tackle the sparsity problem in high resolution by leveraging the flexible structure of GCNs and providing a subdivision algorithm. We build our model with Graph Convolutional Gated Recurrent Units (Graph-ConvGRU) to learn spatial, temporal, and categorical relations. In each node of the graph, we learn a multivariate probability distribution from the extracted features of GCNs. We perform experiments on real-life and synthetic datasets, and our model obtains the best validation and the best test score among the baseline models with significant improvements. We show that our model is not only generative but also precise.
更多查看译文
关键词
crime prediction,graph neural networks,multivariate normal
AI 理解论文
溯源树
样例
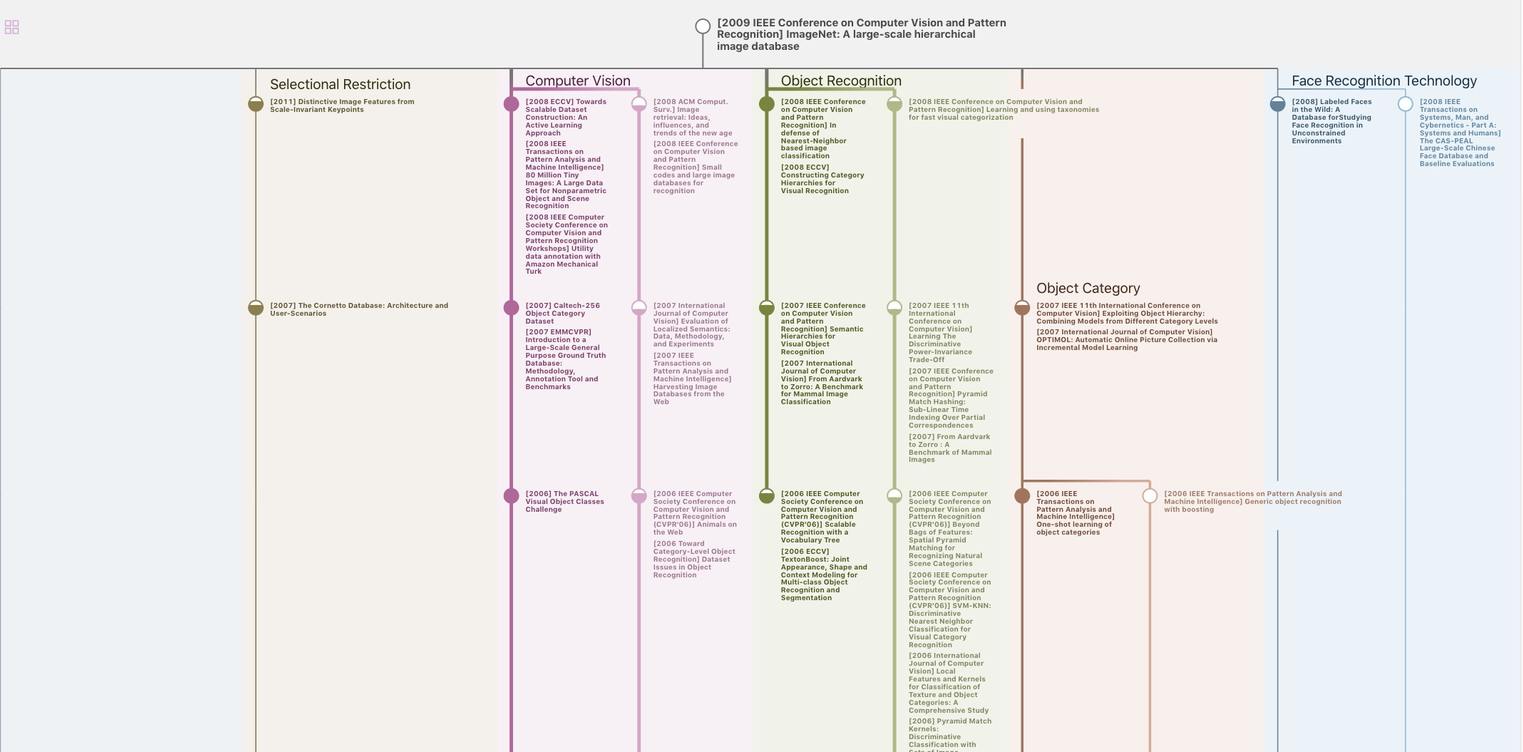
生成溯源树,研究论文发展脉络
Chat Paper
正在生成论文摘要