On the Convergence and Calibration of Deep Learning with Differential Privacy.
Transactions on machine learning research(2023)
摘要
Differentially private (DP) training preserves the data privacy usually at the cost of slower convergence (and thus lower accuracy), as well as more severe mis-calibration than its non-private counterpart. To analyze the convergence of DP training, we formulate a continuous time analysis through the lens of neural tangent kernel (NTK), which characterizes the per-sample gradient clipping and the noise addition in DP training, for arbitrary network architectures and loss functions. Interestingly, we show that the noise addition only affects the privacy risk but not the convergence or calibration, whereas the per-sample gradient clipping (under both flat and layerwise clipping styles) only affects the convergence and calibration. Furthermore, we observe that while DP models trained with small clipping norm usually achieve the best accurate, but are poorly calibrated and thus unreliable. In sharp contrast, DP models trained with large clipping norm enjoy the same privacy guarantee and similar accuracy, but are significantly more calibrated. Our code can be found at https://github.com/woodyx218/opacus_global_clipping.
更多查看译文
关键词
differential
AI 理解论文
溯源树
样例
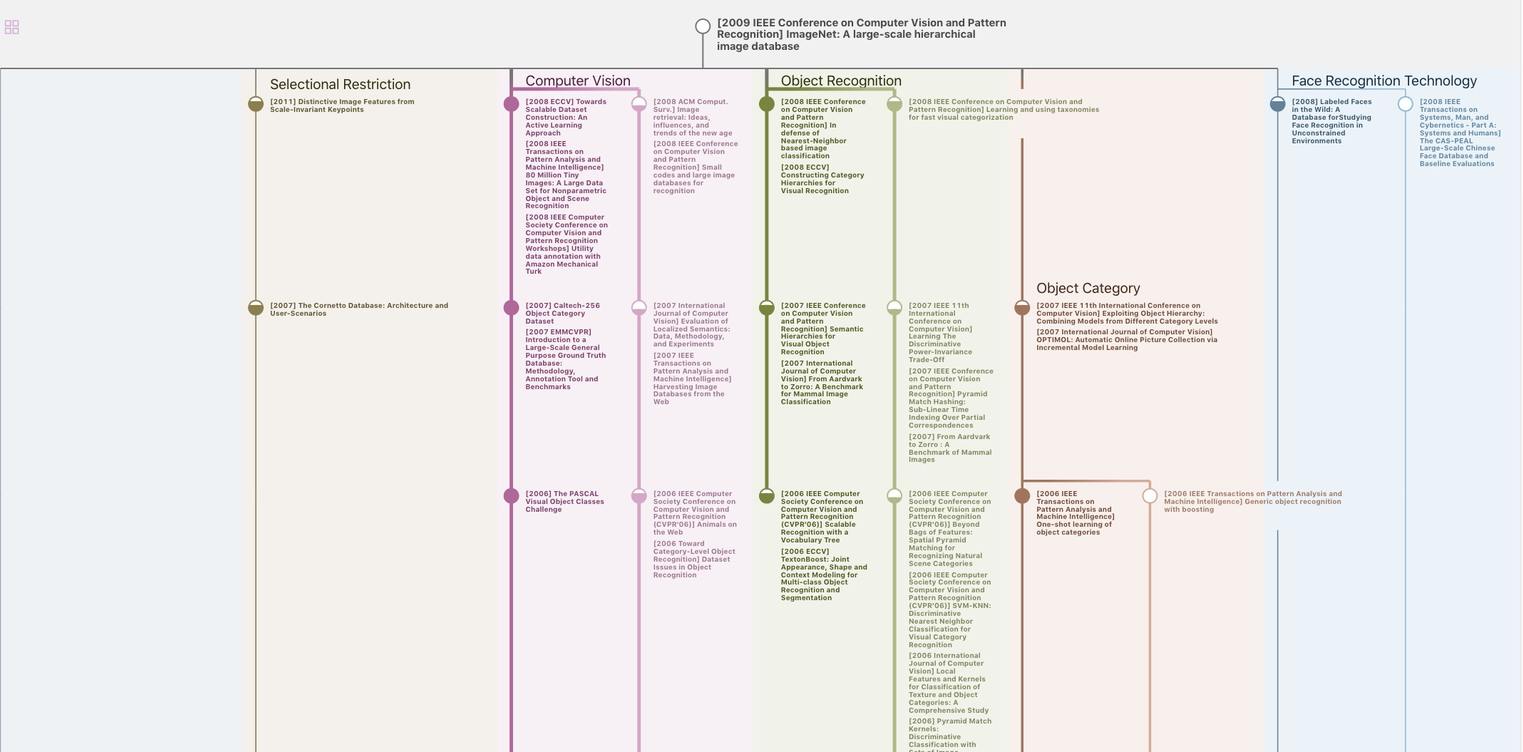
生成溯源树,研究论文发展脉络
Chat Paper
正在生成论文摘要