Identifiability of interaction kernels in mean-field equations of interacting particles
Foundations of data science(2023)
摘要
This study examines the identifiability of interaction kernels in mean-field equations of interacting particles or agents, an area of growing interest across various scientific and engineering fields. The main focus is identifying data-dependent function spaces where a quadratic loss functional possesses a unique minimizer. We consider two data-adaptive $ L^2 $ spaces: one weighted by a data-adaptive measure and the other using the Lebesgue measure. In each $ L^2 $ space, we show that the function space of identifiability is the closure of the RKHS associated with the integral operator of inversion. Alongside prior research, our study completes a full characterization of identifiability in interacting particle systems with either finite or infinite particles, highlighting critical differences between these two settings. Moreover, the identifiability analysis has important implications for computational practice. It shows that the inverse problem is ill-posed, necessitating regularization. Our numerical demonstrations show that the weighted $ L^2 $ space is preferable over the unweighted $ L^2 $ space, as it yields more accurate regularized estimators.
更多查看译文
关键词
interaction kernels,particles,mean-field
AI 理解论文
溯源树
样例
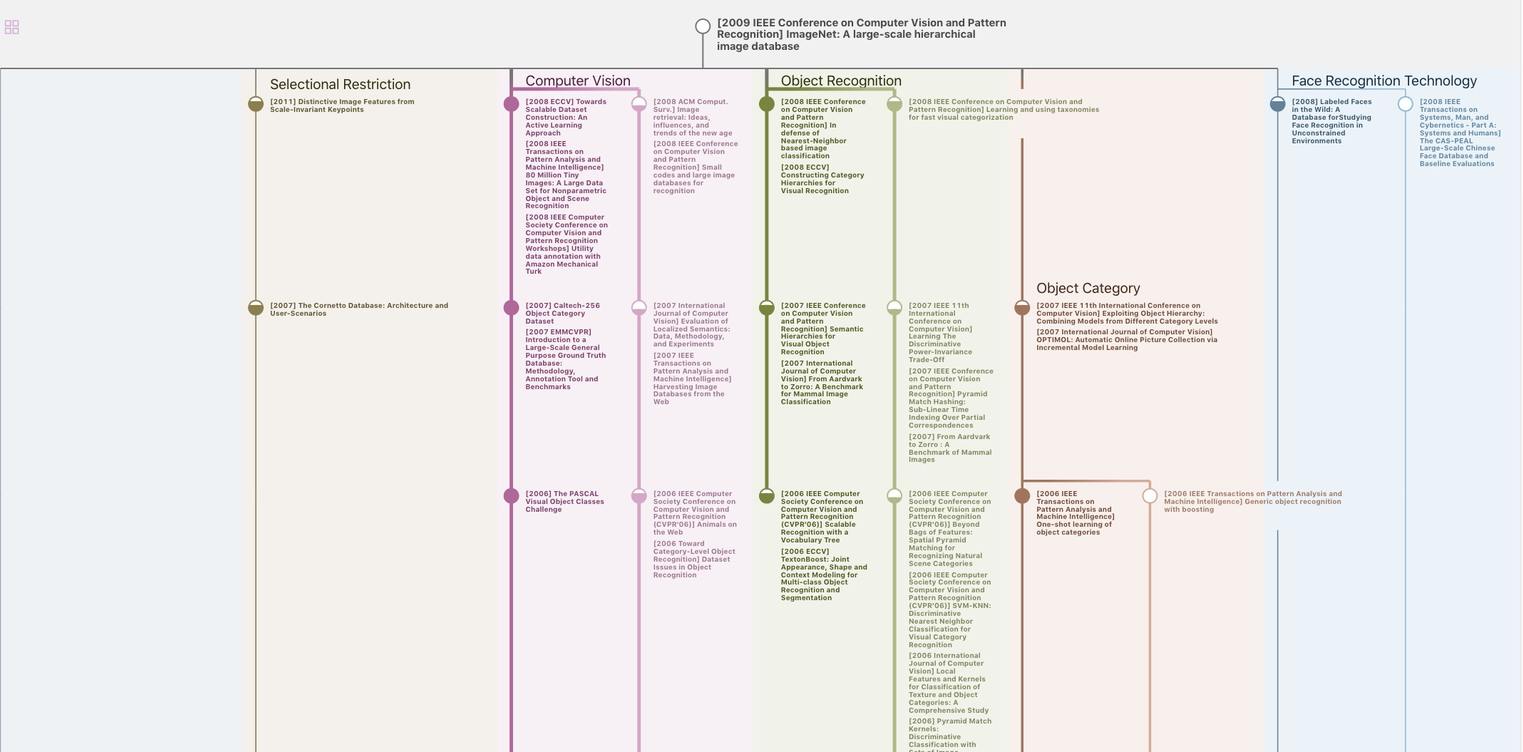
生成溯源树,研究论文发展脉络
Chat Paper
正在生成论文摘要