CrossCLR: Cross-modal Contrastive Learning For Multi-modal Video Representations
arXiv (Cornell University)(2021)
摘要
Contrastive learning allows us to flexibly define powerful losses by contrasting positive pairs from sets of negative samples. Recently, the principle has also been used to learn cross-modal embeddings for video and text, yet without exploiting its full potential. In particular, previous losses do not take the intra-modality similarities into account, which leads to inefficient embeddings, as the same content is mapped to multiple points in the embedding space. With CrossCLR, we present a contrastive loss that fixes this issue. Moreover, we define sets of highly related samples in terms of their input embeddings and exclude them from the negative samples to avoid issues with false negatives. We show that these principles consistently improve the quality of the learned embeddings. The joint embeddings learned with CrossCLR extend the state of the art in video-text retrieval on Youcook2 and LSMDC datasets and in video captioning on Youcook2 dataset by a large margin. We also demonstrate the generality of the concept by learning improved joint embeddings for other pairs of modalities.
更多查看译文
关键词
representations,learning,cross-modal,multi-modal
AI 理解论文
溯源树
样例
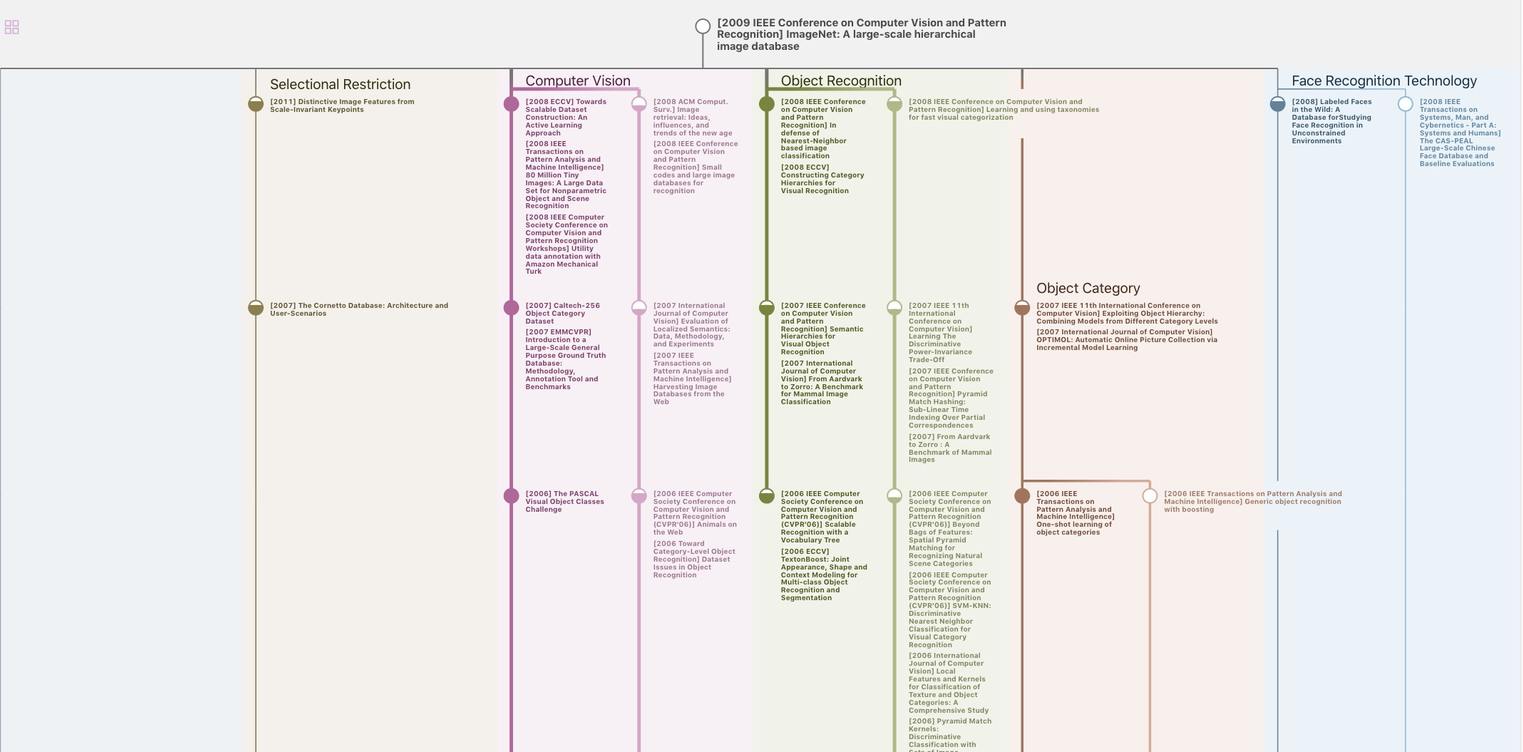
生成溯源树,研究论文发展脉络
Chat Paper
正在生成论文摘要