Joint Dynamic Models and Statistical Inference for Recurrent Competing Risks, Longitudinal Marker, and Health Status
arXiv (Cornell University)(2021)
摘要
Consider a subject or unit in a longitudinal biomedical, public health, engineering, economic or social science study which is being monitored over a possibly random duration. Over time this unit experiences recurrent events of several types and a longitudinal marker transitions over a discrete state-space. In addition, its "health" status also transitions over a discrete state-space with at least one absorbing state. A vector of covariates will also be associated with this unit. Of major interest for this unit is the time-to-absorption of its health status process, which could be viewed as the unit's lifetime. Aside from being affected by its covariate vector, there could be associations among the recurrent competing risks processes, the longitudinal marker process, and the health status process in the sense that the time-evolution of each process is associated with the other processes. To obtain more realistic models and enhance inferential performance, a joint dynamic stochastic model for these components is proposed and statistical inference methods are developed. This joint model, formulated via counting processes and continuous-time Markov chains, has the potential of facilitating `personalized' interventions. This could enhance, for example, the implementation and adoption of precision medicine in medical settings. Semi-parametric and likelihood-based inferential methods for the model parameters are developed when a sample of these units is available. Finite-sample and asymptotic properties of estimators of model parameters, both finite- and infinite-dimensional, are obtained analytically or through simulation studies. The developed procedures are illustrated using a real data set.
更多查看译文
关键词
joint dynamic models,competing
AI 理解论文
溯源树
样例
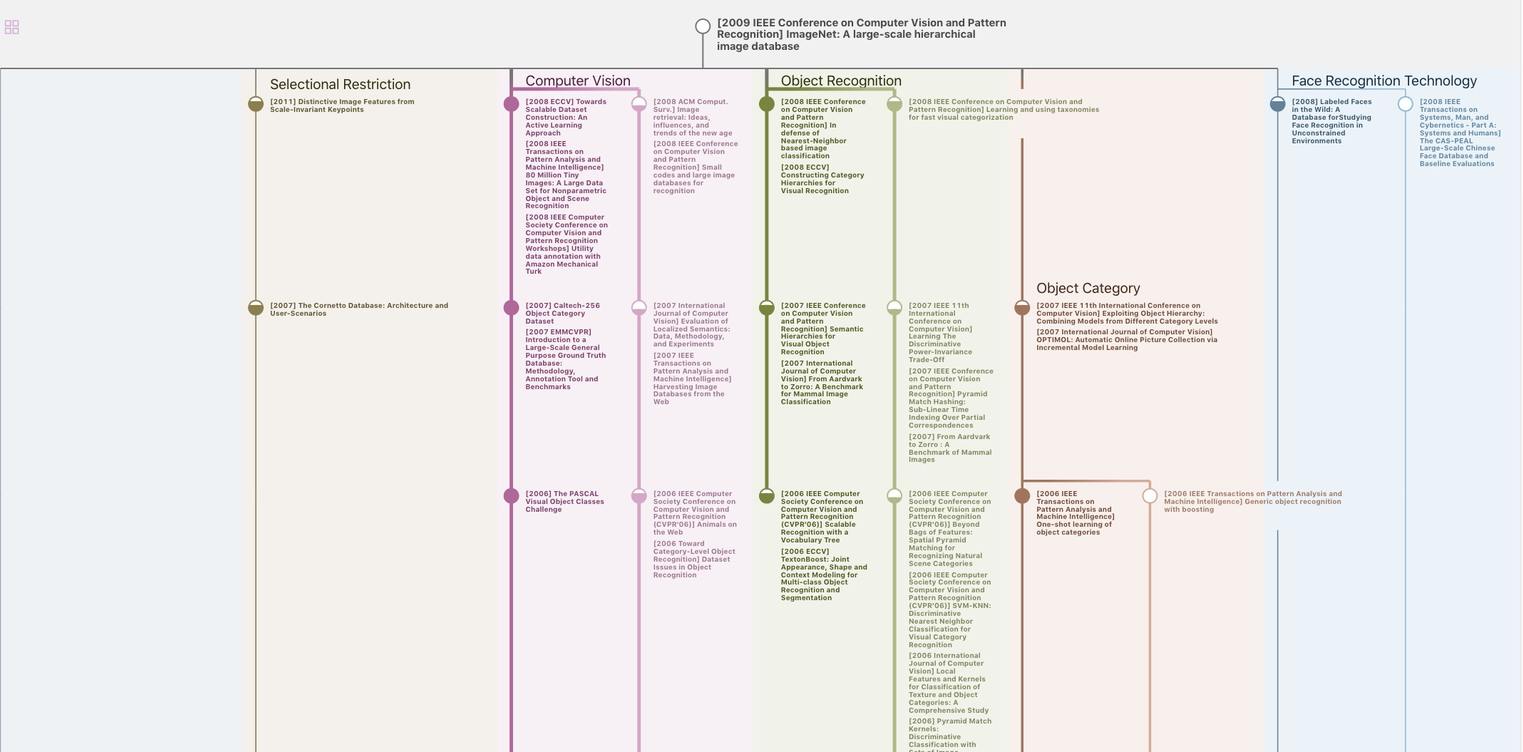
生成溯源树,研究论文发展脉络
Chat Paper
正在生成论文摘要