LLM360: Towards Fully Transparent Open-Source LLMs
CoRR(2023)
摘要
The recent surge in open-source Large Language Models (LLMs), such as LLaMA,
Falcon, and Mistral, provides diverse options for AI practitioners and
researchers. However, most LLMs have only released partial artifacts, such as
the final model weights or inference code, and technical reports increasingly
limit their scope to high-level design choices and surface statistics. These
choices hinder progress in the field by degrading transparency into the
training of LLMs and forcing teams to rediscover many details in the training
process. We present LLM360, an initiative to fully open-source LLMs, which
advocates for all training code and data, model checkpoints, and intermediate
results to be made available to the community. The goal of LLM360 is to support
open and collaborative AI research by making the end-to-end LLM training
process transparent and reproducible by everyone. As a first step of LLM360, we
release two 7B parameter LLMs pre-trained from scratch, Amber and CrystalCoder,
including their training code, data, intermediate checkpoints, and analyses (at
https://www.llm360.ai). We are committed to continually pushing the boundaries
of LLMs through this open-source effort. More large-scale and stronger models
are underway and will be released in the future.
更多查看译文
AI 理解论文
溯源树
样例
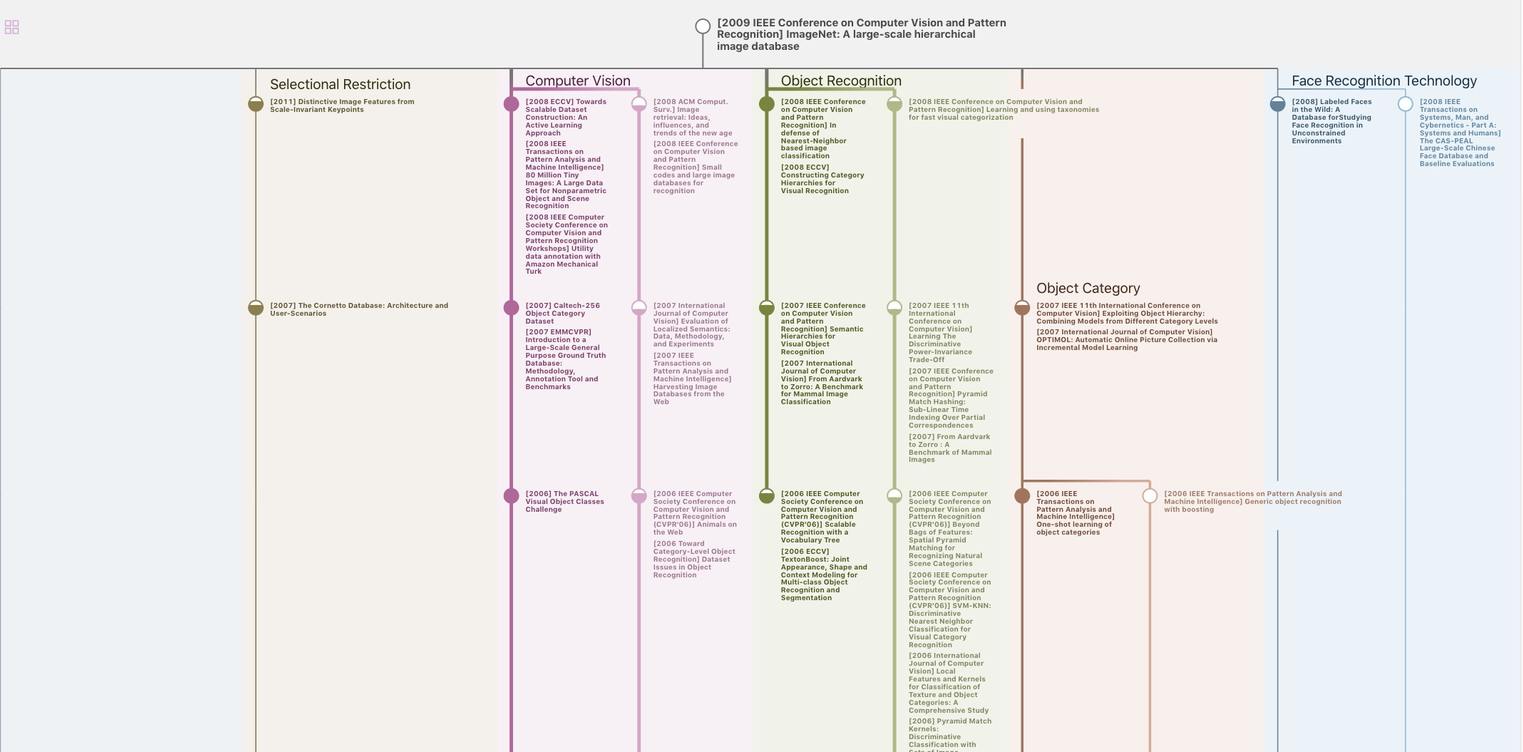
生成溯源树,研究论文发展脉络
Chat Paper
正在生成论文摘要