Relevant Intrinsic Feature Enhancement Network for Few-Shot Semantic Segmentation
AAAI 2024(2024)
摘要
For few-shot semantic segmentation, the primary task is to extract class-specific intrinsic information from limited labeled data. However, the semantic ambiguity and inter-class similarity of previous methods limit the accuracy of pixel-level foreground-background classification. To alleviate these issues, we propose the Relevant Intrinsic Feature Enhancement Network (RiFeNet). To improve the semantic consistency of foreground instances, we propose an unlabeled branch as an efficient data utilization method, which teaches the model how to extract intrinsic features robust to intra-class differences. Notably, during testing, the proposed unlabeled branch is excluded without extra unlabeled data and computation. Furthermore, we extend the inter-class variability between foreground and background by proposing a novel multi-level prototype generation and interaction module. The different-grained complementarity between global and local prototypes allows for better distinction between similar categories. The qualitative and quantitative performance of RiFeNet surpasses the state-of-the-art methods on PASCAL-5i and COCO benchmarks.
更多查看译文
关键词
CV: Segmentation
AI 理解论文
溯源树
样例
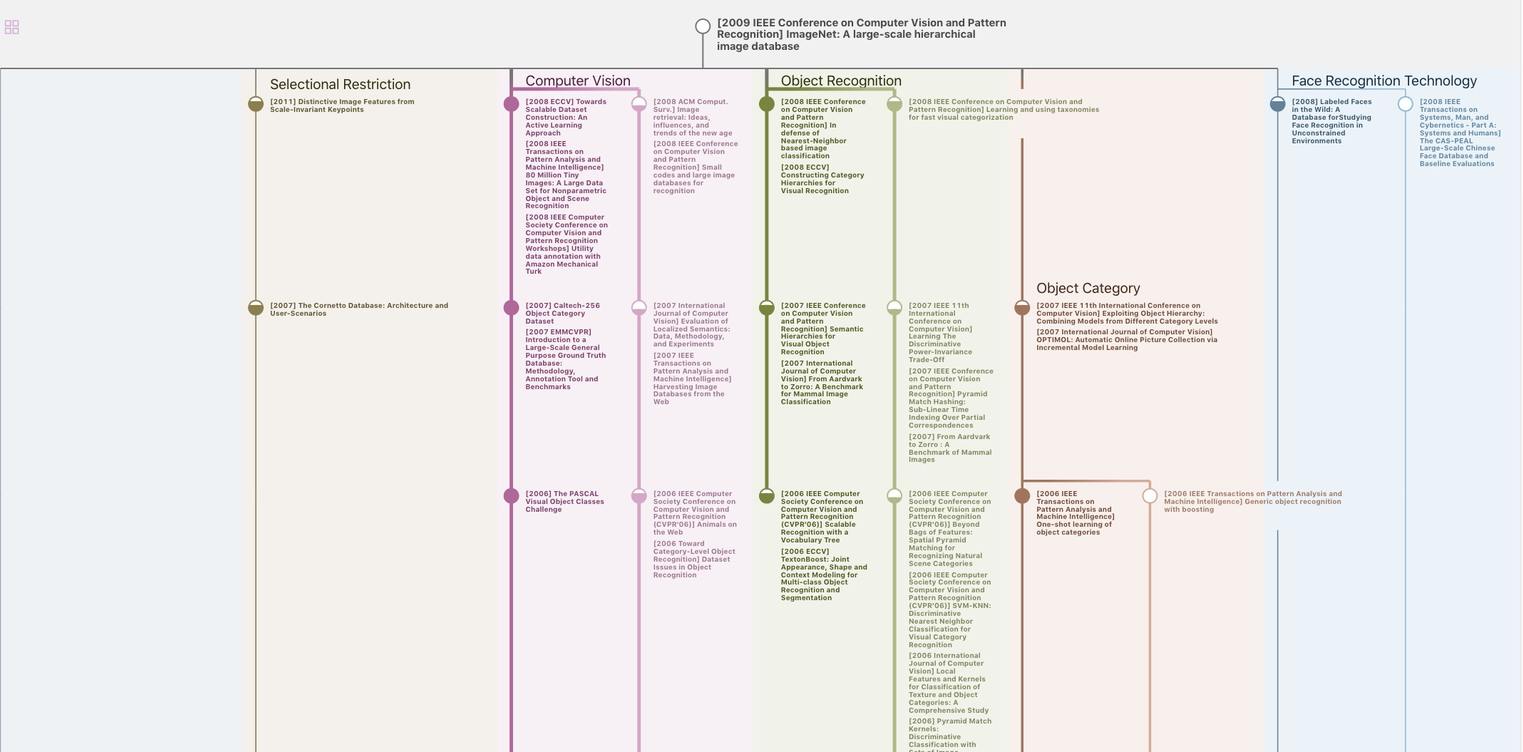
生成溯源树,研究论文发展脉络
Chat Paper
正在生成论文摘要