Dropout is all you need: robust two-qubit gate with reinforcement learning
arxiv(2023)
摘要
In the realm of quantum control, reinforcement learning, a prominent branch
of machine learning, emerges as a competitive candidate for computer-assisted
optimal design for experiments. This study investigates the extent to which
guidance from human experts is necessary for the effective implementation of
reinforcement learning in designing quantum control protocols. Specifically, we
focus on the engineering of a robust two-qubit gate within a nuclear magnetic
resonance system, utilizing a combination of analytical solutions as prior
knowledge and techniques from the field of computer science. Through extensive
benchmarking of different models, we identify dropout, a widely-used method for
mitigating overfitting in machine learning, as an especially robust approach.
Our findings demonstrate the potential of incorporating computer science
concepts to propel the development of advanced quantum technologies.
更多查看译文
AI 理解论文
溯源树
样例
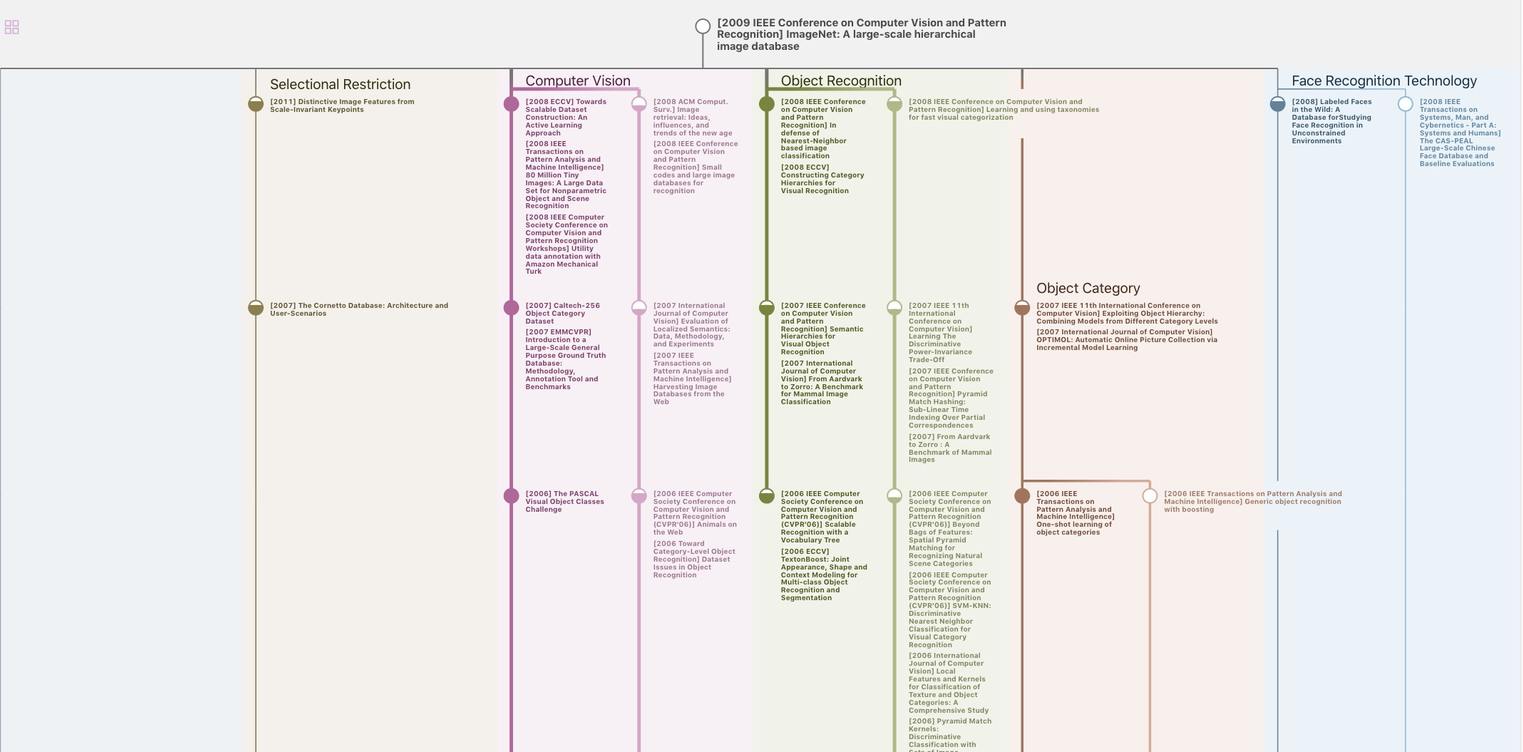
生成溯源树,研究论文发展脉络
Chat Paper
正在生成论文摘要