On the approximability of random-hypergraph MAX-3-XORSAT problems with quantum algorithms
arxiv(2023)
摘要
Constraint satisfaction problems are an important area of computer science.
Many of these problems are in the complexity class NP which is exponentially
hard for all known methods, both for worst cases and often typical.
Fundamentally, the lack of any guided local minimum escape method ensures the
hardness of both exact and approximate optimization classically, but the
intuitive mechanism for approximation hardness in quantum algorithms based on
Hamiltonian time evolution is poorly understood. We explore this question using
the prototypically hard MAX-3-XORSAT problem class. We conclude that the
mechanisms for quantum exact and approximation hardness are fundamentally
distinct. We qualitatively identify why traditional methods such as quantum
adiabatic optimization are not good approximation algorithms. We propose a new
spectral folding optimization method that does not suffer from these issues and
study it analytically and numerically. We consider random rank-3 hypergraphs
including extremal planted solution instances, where the ground state satisfies
an anomalously high fraction of constraints compared to truly random problems.
We show that, if we define the energy to be E = N_unsat-N_sat, then
spectrally folded quantum optimization will return states with energy E ≤ A
E_GS (where E_GS is the ground state energy) in polynomial time, where
conservatively, A ≃ 0.6. We thoroughly benchmark variations of
spectrally folded quantum optimization for random classically
approximation-hard (planted solution) instances in simulation, and find
performance consistent with this prediction. We do not claim that this
approximation guarantee holds for all possible hypergraphs, though our
algorithm's mechanism can likely generalize widely. These results suggest that
quantum computers are more powerful for approximate optimization than had been
previously assumed.
更多查看译文
AI 理解论文
溯源树
样例
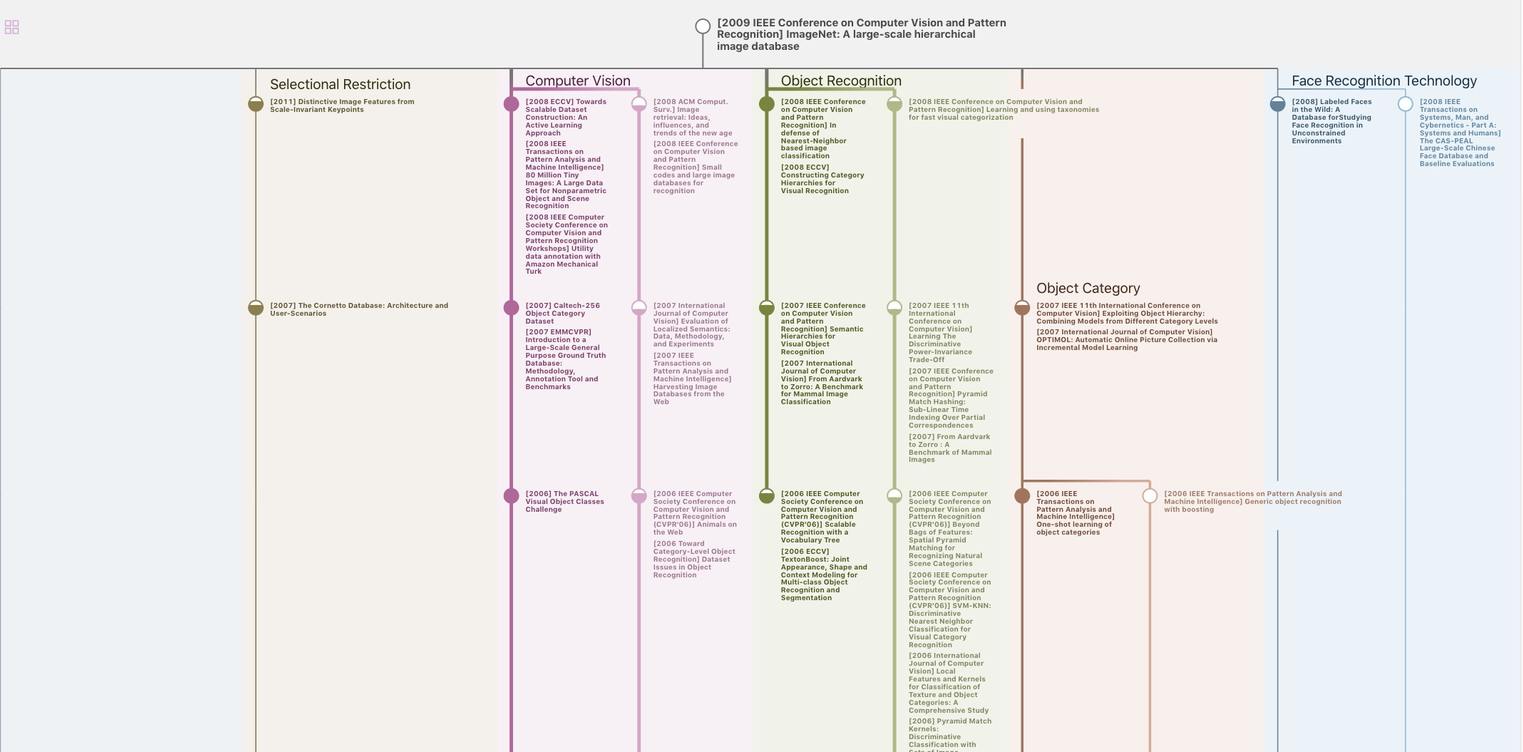
生成溯源树,研究论文发展脉络
Chat Paper
正在生成论文摘要