Learning the Causal Structure of Networked Dynamical Systems under Latent Nodes and Structured Noise
AAAI 2024(2024)
Abstract
This paper considers learning the hidden causal network of a linear networked dynamical system (NDS) from the time series data at some of its nodes -- partial observability. The dynamics of the NDS are driven by colored noise that generates spurious associations across pairs of nodes, rendering the problem much harder. To address the challenge of noise correlation and partial observability, we assign to each pair of nodes a feature vector computed from the time series data of observed nodes. The feature embedding is engineered to yield structural consistency: there exists an affine hyperplane that consistently partitions the set of features, separating the feature vectors corresponding to connected pairs of nodes from those corresponding to disconnected pairs. The causal inference problem is thus addressed via clustering the designed features. We demonstrate with simple baseline supervised methods the competitive performance of the proposed causal inference mechanism under broad connectivity regimes and noise correlation levels, including a real world network. Further, we devise novel technical guarantees of structural consistency for linear NDS under the considered regime.
MoreTranslated text
Key words
ML: Causal Learning,ML: Graph-based Machine Learning,ML: Learning Theory,ML: Probabilistic Circuits and Graphical Models,ML: Time-Series/Data Streams,ML: Transparent, Interpretable, Explainable ML
AI Read Science
Must-Reading Tree
Example
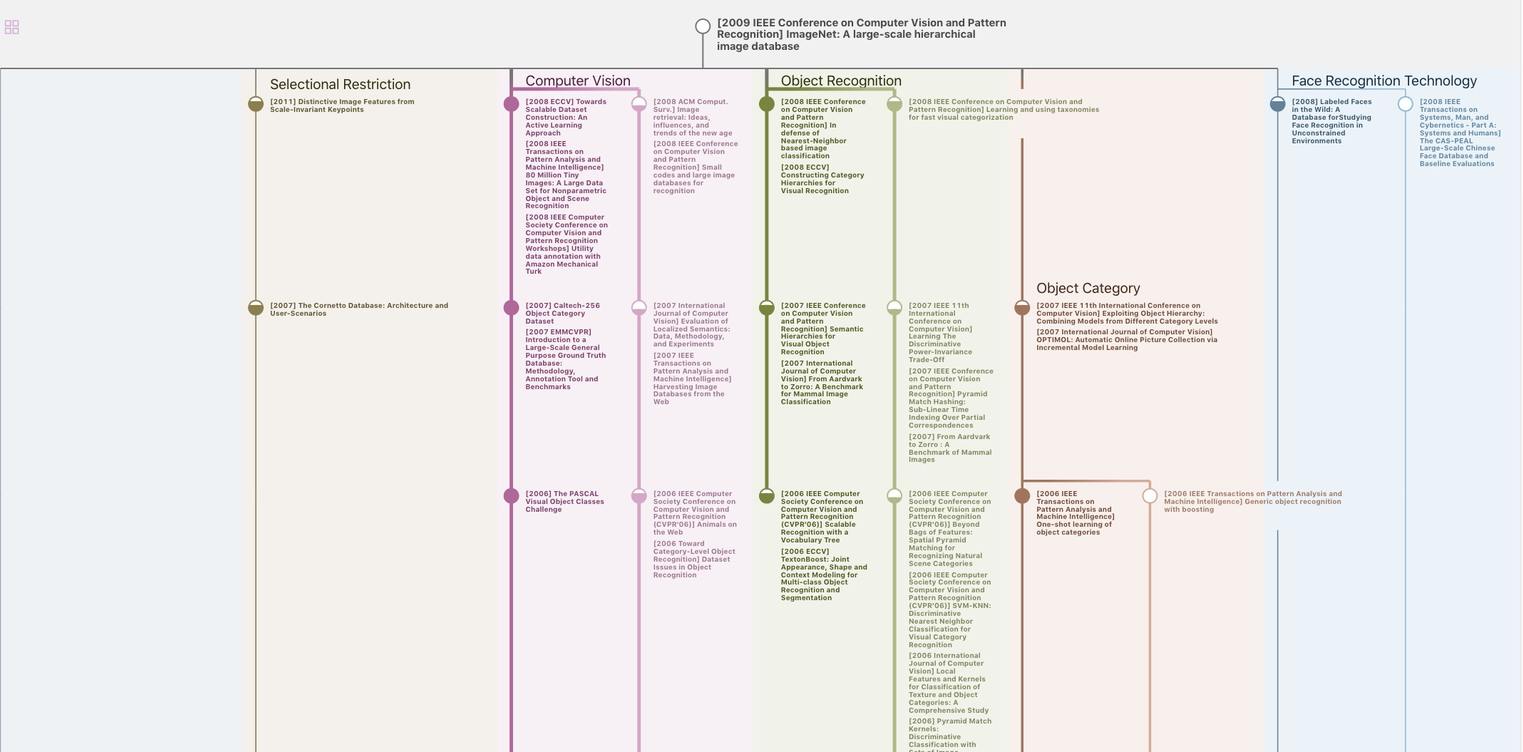
Generate MRT to find the research sequence of this paper
Chat Paper
Summary is being generated by the instructions you defined