AesFA: An Aesthetic Feature-Aware Arbitrary Neural Style Transfer
AAAI 2024(2024)
摘要
Neural style transfer (NST) has evolved significantly in recent years. Yet, despite its rapid progress and advancement, existing NST methods either struggle to transfer aesthetic information from a style effectively or suffer from high computational costs and inefficiencies in feature disentanglement due to using pre-trained models. This work proposes a lightweight but effective model, AesFA---Aesthetic Feature-Aware NST. The primary idea is to decompose the image via its frequencies to better disentangle aesthetic styles from the reference image while training the entire model in an end-to-end manner to exclude pre-trained models at inference completely. To improve the network's ability to extract more distinct representations and further enhance the stylization quality, this work introduces a new aesthetic feature: contrastive loss. Extensive experiments and ablations show the approach not only outperforms recent NST methods in terms of stylization quality, but it also achieves faster inference. Codes are available at https://github.com/Sooyyoungg/AesFA.
更多查看译文
关键词
ML: Deep Generative Models & Autoencoders,CV: Applications,CV: Computational Photography, Image & Video Synthesis,General
AI 理解论文
溯源树
样例
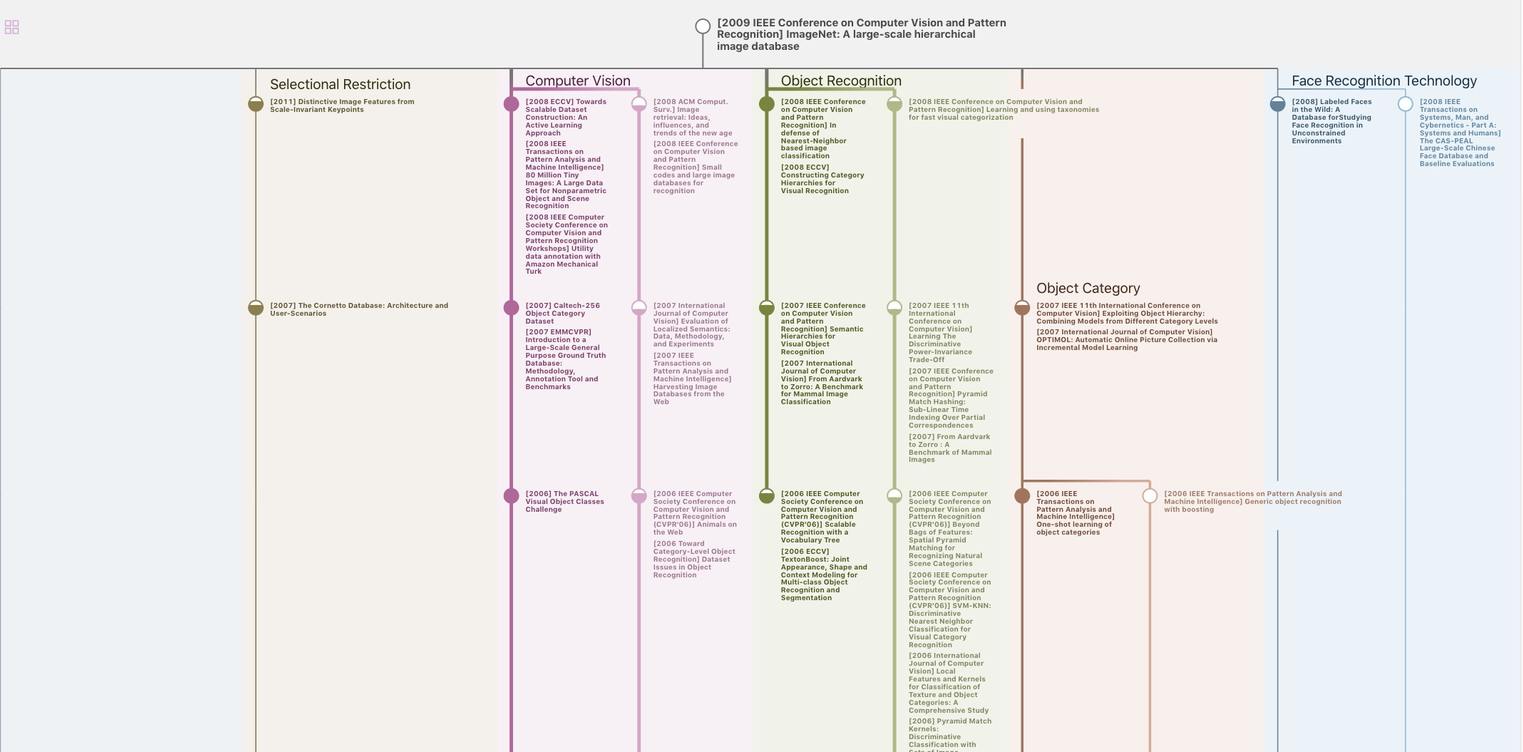
生成溯源树,研究论文发展脉络
Chat Paper
正在生成论文摘要