Hyperbolic Network Latent Space Model with Learnable Curvature
arxiv(2023)
摘要
Network data is ubiquitous in various scientific disciplines, including
sociology, economics, and neuroscience. Latent space models are often employed
in network data analysis, but the geometric effect of latent space curvature
remains a significant, unresolved issue. In this work, we propose a hyperbolic
network latent space model with a learnable curvature parameter. We
theoretically justify that learning the optimal curvature is essential to
minimizing the embedding error across all hyperbolic embedding methods beyond
network latent space models. A maximum-likelihood estimation strategy,
employing manifold gradient optimization, is developed, and we establish the
consistency and convergence rates for the maximum-likelihood estimators, both
of which are technically challenging due to the non-linearity and non-convexity
of the hyperbolic distance metric. We further demonstrate the geometric effect
of latent space curvature and the superior performance of the proposed model
through extensive simulation studies and an application using a Facebook
friendship network.
更多查看译文
AI 理解论文
溯源树
样例
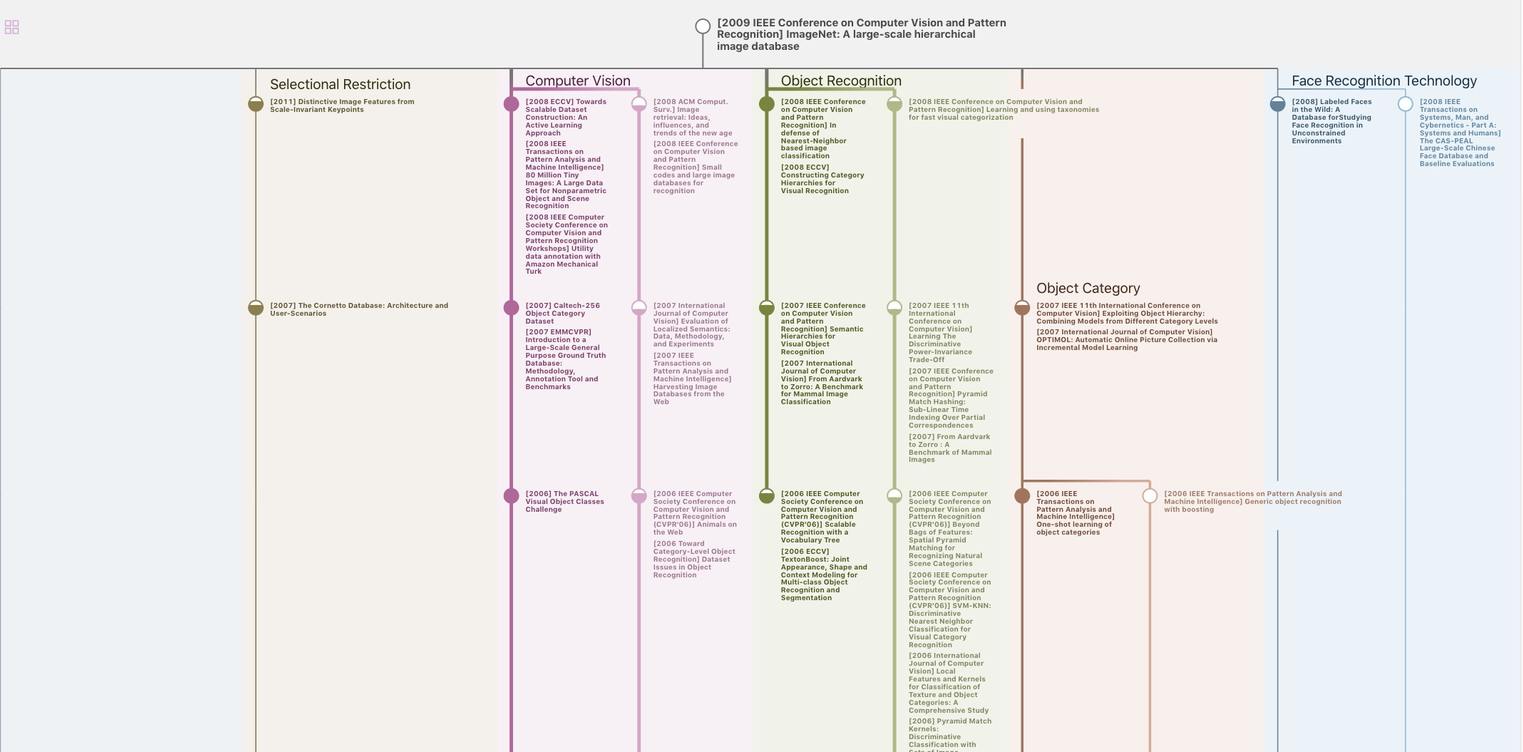
生成溯源树,研究论文发展脉络
Chat Paper
正在生成论文摘要