Fused Extended Two-Way Fixed Effects for Difference-in-Differences with Staggered Adoptions
arxiv(2023)
摘要
To address the bias of the canonical two-way fixed effects estimator for
difference-in-differences under staggered adoptions, Wooldridge (2021) proposed
the extended two-way fixed effects estimator, which adds many parameters.
However, this reduces efficiency. Restricting some of these parameters to be
equal (for example, subsequent treatment effects within a cohort) helps, but ad
hoc restrictions may reintroduce bias. We propose a machine learning estimator
with a single tuning parameter, fused extended two-way fixed effects (FETWFE),
that enables automatic data-driven selection of these restrictions. We prove
that under an appropriate sparsity assumption FETWFE identifies the correct
restrictions with probability tending to one, which improves efficiency. We
also prove the consistency, oracle property, and asymptotic normality of FETWFE
for several classes of heterogeneous marginal treatment effect estimators under
either conditional or marginal parallel trends, and we prove the same results
for conditional average treatment effects under conditional parallel trends. We
demonstrate FETWFE in simulation studies and an empirical application.
更多查看译文
AI 理解论文
溯源树
样例
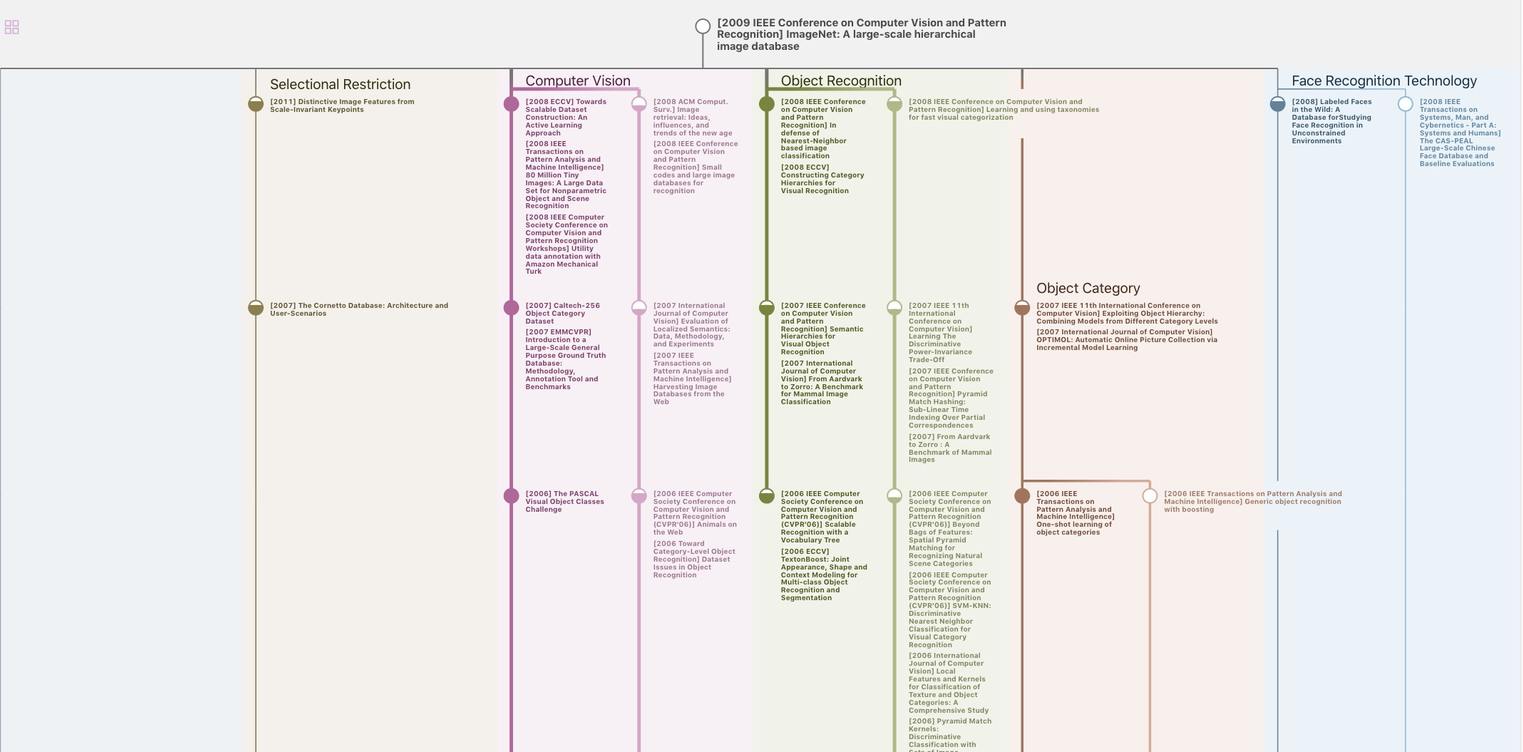
生成溯源树,研究论文发展脉络
Chat Paper
正在生成论文摘要