Tighter expected generalization error bounds via Wasserstein distance
arXiv (Cornell University)(2021)
摘要
In this work, we introduce several expected generalization error bounds based on the Wasserstein distance. More precisely, we present full-dataset, single-letter, and random-subset bounds on both the standard setting and the randomized-subsample setting from Steinke and Zakynthinou [2020]. Moreover, we show that, when the loss function is bounded, these bounds recover from below (and thus are tighter than) current bounds based on the relative entropy and, for the standard setting, generate new, non-vacuous bounds also based on the relative entropy. Then, we show how similar bounds featuring the backward channel can be derived with the proposed proof techniques. Finally, we show how various new bounds based on different information measures (e.g., the lautum information or several $f$-divergences) can be derived from the presented bounds.
更多查看译文
关键词
wasserstein distance,generalization error
AI 理解论文
溯源树
样例
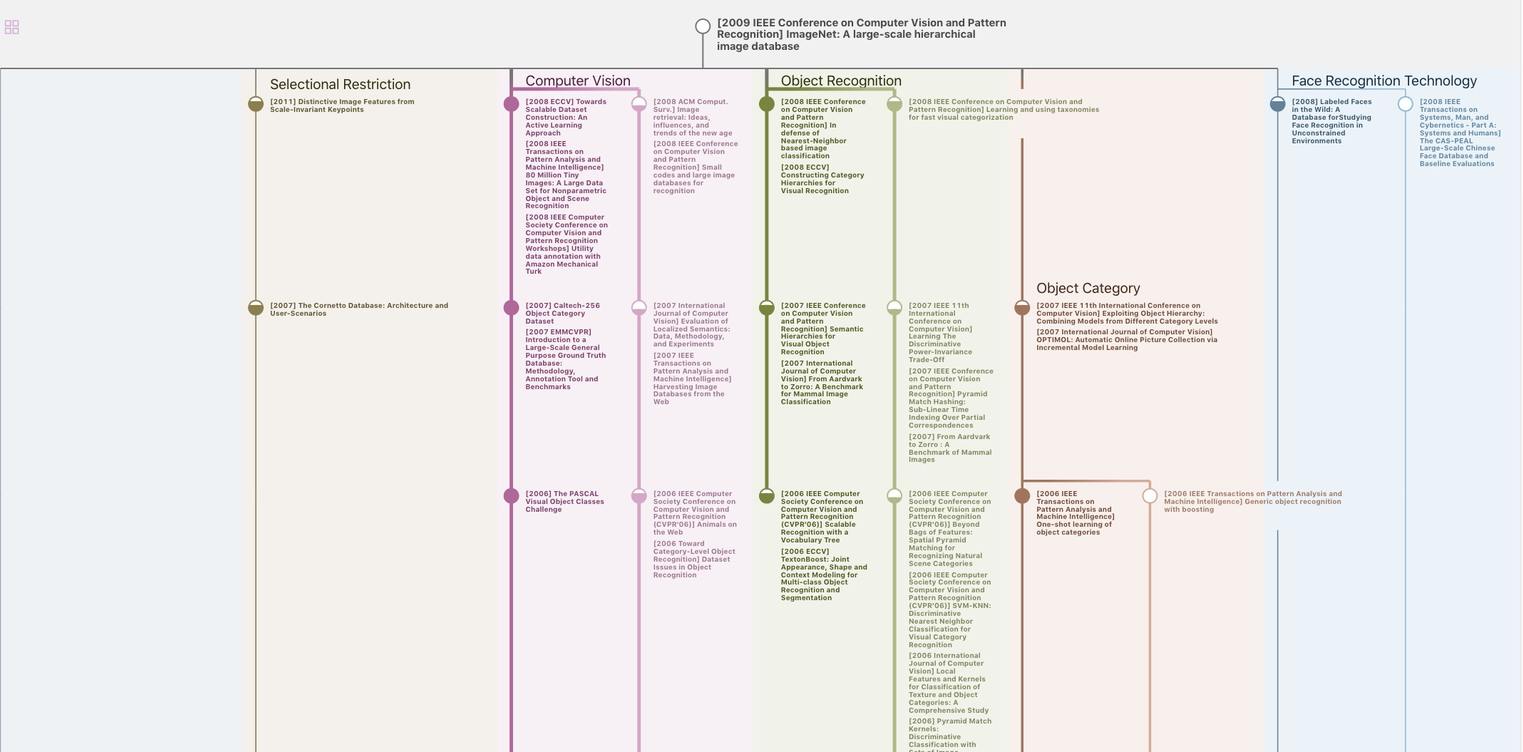
生成溯源树,研究论文发展脉络
Chat Paper
正在生成论文摘要