DPWTE: A Deep Learning Approach to Time-to-Event Analysis using a Sparse Weibull Mixture Layer
HAL (Le Centre pour la Communication Scientifique Directe)(2021)
摘要
n this paper, we propose a deep-network-based approach that leverages a finite mixture of Weibull distributions to address a key challenge in time-to-event modeling: it comes to a parametric estimation of survival time distribution from censored data. The proposed model predicts the parameters of the mixture of Weibull distributions with respect to input variables. In addition, given that we set beforehand the upper bound of the mixture size, the model finds the requisite number of Weibull distributions to combine in order to model the event time distribution as accurately as possible. For this purpose, we introduce the Sparse Weibull Mixture layer that selects indirectly, through its weights, the Weibull dis-tributions composing the mixture, whose mixing parameters are significant. To stimulate this selection, we apply a sparse regularization on this layer by adding a penalty term to the loss function that takes into account both observed and censored observations. We validate our method on both simulated and real datasets, showing that the proposed methodology yields a performance improvement over the state-of-the-art models proposed in this work.
更多查看译文
关键词
deep learning,deep learning approach,time-to-event
AI 理解论文
溯源树
样例
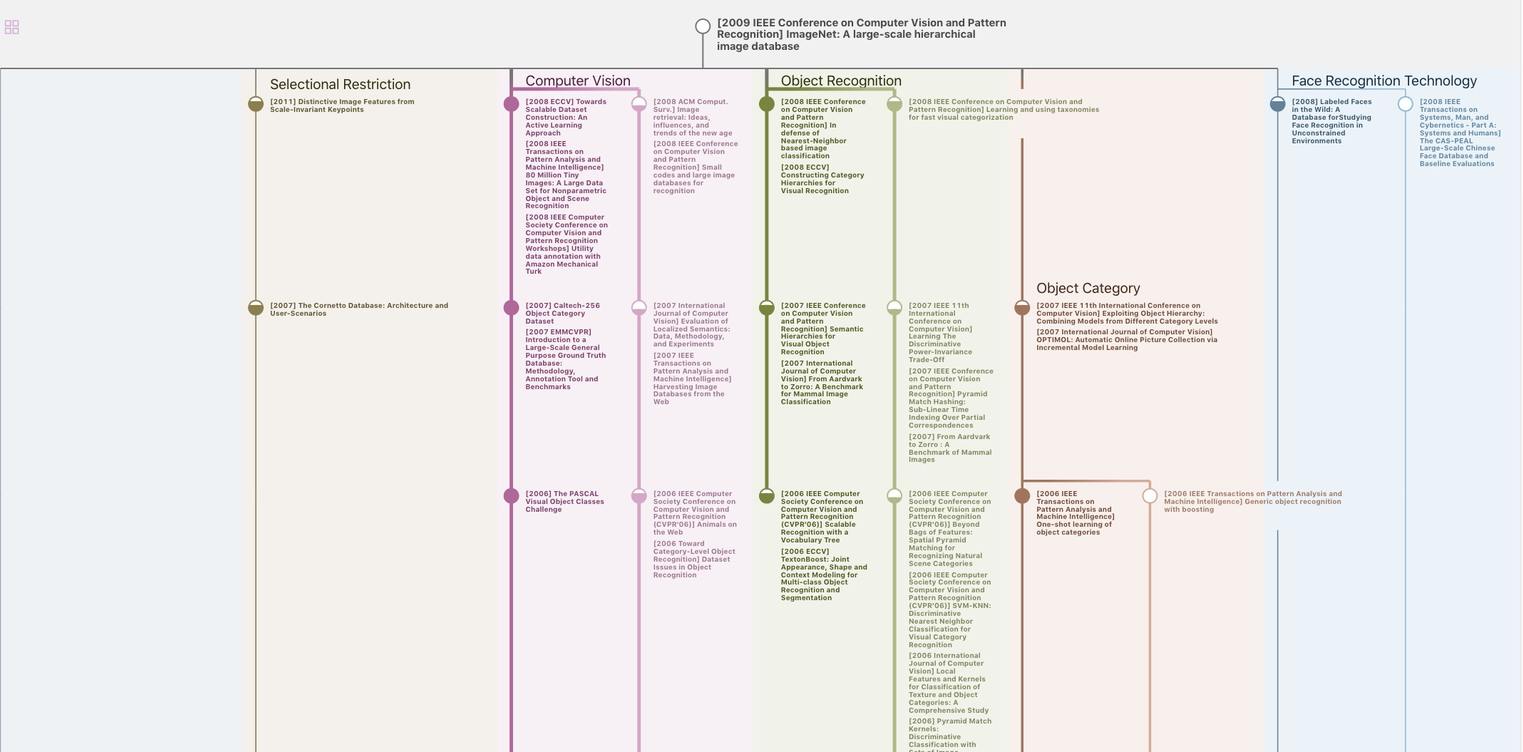
生成溯源树,研究论文发展脉络
Chat Paper
正在生成论文摘要