Model-free Policy Learning with Reward Gradients
arXiv (Cornell University)(2021)
摘要
Despite the increasing popularity of policy gradient methods, they are yet to be widely utilized in sample-scarce applications, such as robotics. The sample efficiency could be improved by making best usage of available information. As a key component in reinforcement learning, the reward function is usually devised carefully to guide the agent. Hence, the reward function is usually known, allowing access to not only scalar reward signals but also reward gradients. To benefit from reward gradients, previous works require the knowledge of environment dynamics, which are hard to obtain. In this work, we develop the \textit{Reward Policy Gradient} estimator, a novel approach that integrates reward gradients without learning a model. Bypassing the model dynamics allows our estimator to achieve a better bias-variance trade-off, which results in a higher sample efficiency, as shown in the empirical analysis. Our method also boosts the performance of Proximal Policy Optimization on different MuJoCo control tasks.
更多查看译文
关键词
policy,learning,model-free
AI 理解论文
溯源树
样例
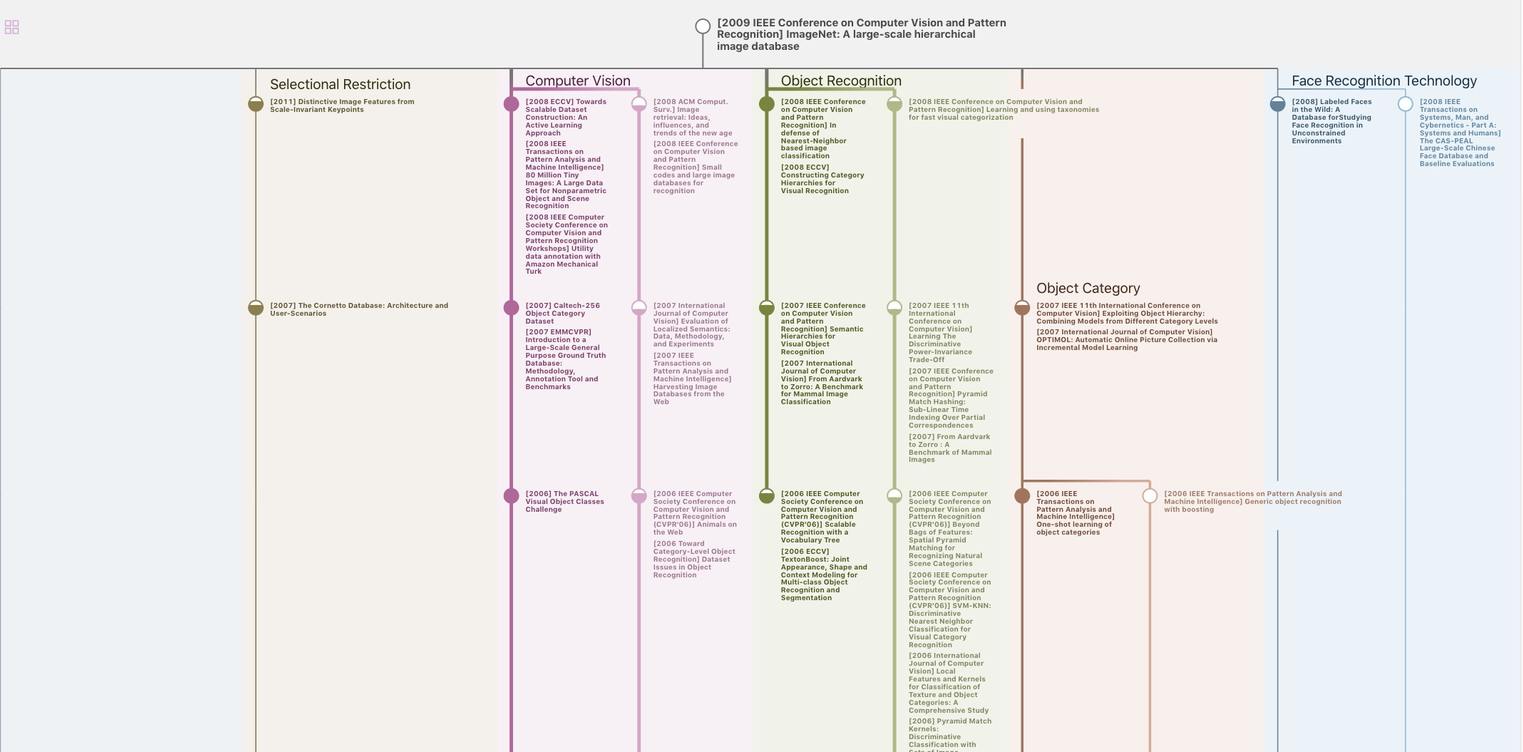
生成溯源树,研究论文发展脉络
Chat Paper
正在生成论文摘要