Pure Differential Privacy from Secure Intermediaries
arXiv (Cornell University)(2021)
摘要
Recent work in differential privacy has explored the prospect of combining local randomization with a secure intermediary. Specifically, there are a variety of protocols in the secure shuffle model (where an intermediary randomly permutes messages) as well as the secure aggregation model (where an intermediary adds messages). Most of these protocols are limited to approximate differential privacy. An exception is the shuffle protocol by Ghazi, Golowich, Kumar, Manurangsi, Pagh, and Velingker (arXiv:2002.01919): it computes bounded sums under pure differential privacy. Its additive error is $\tilde{O}(1/\varepsilon^{3/2})$, where $\varepsilon$ is the privacy parameter. In this work, we give a new protocol that ensures $O(1/\varepsilon)$ error under pure differential privacy. We also show how to use it to test uniformity of distributions over $[d]$. The tester's sample complexity has an optimal dependence on $d$. Our work relies on a novel class of secure intermediaries which are of independent interest.
更多查看译文
关键词
privacy
AI 理解论文
溯源树
样例
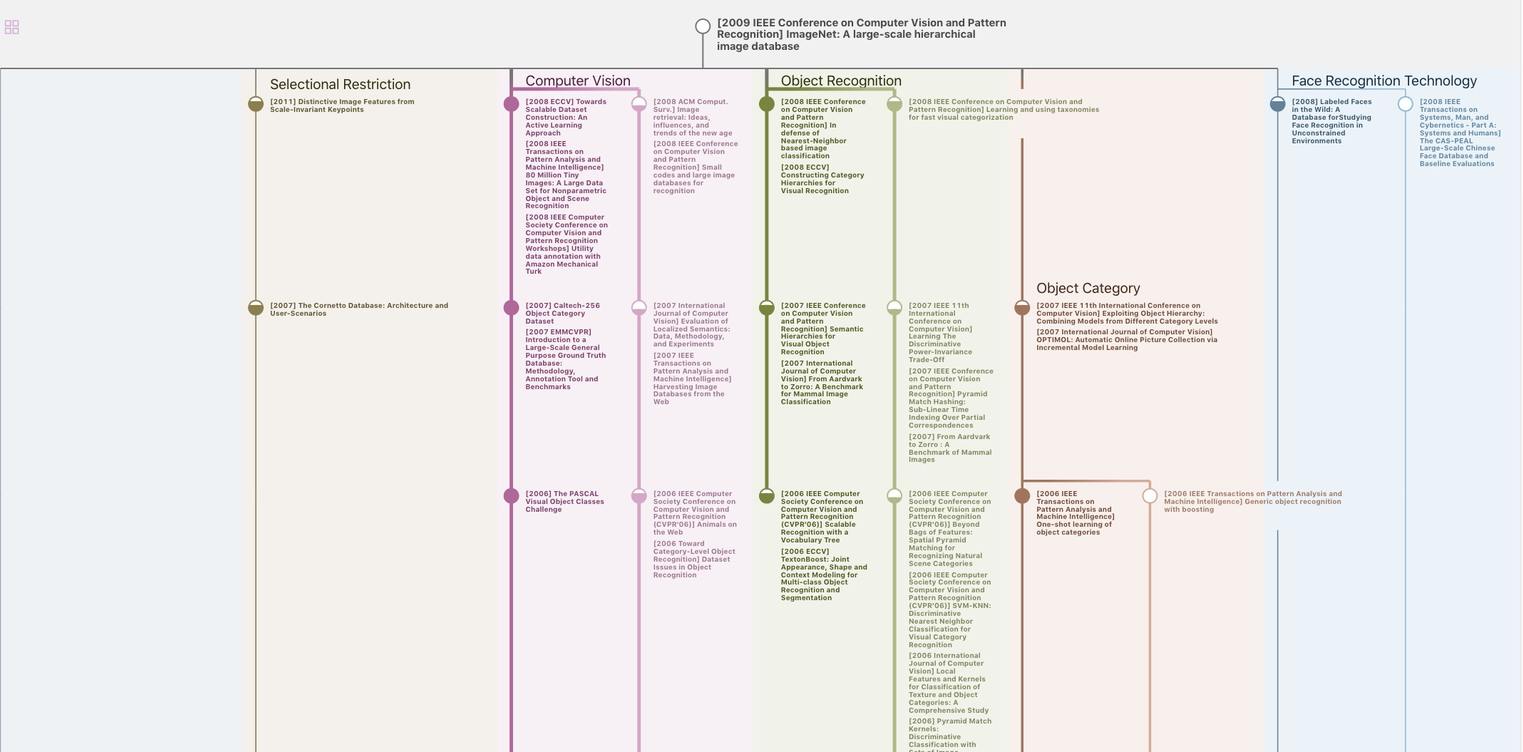
生成溯源树,研究论文发展脉络
Chat Paper
正在生成论文摘要