Accelerated, Optimal, and Parallel: Some Results on Model-Based Stochastic Optimization.
arXiv (Cornell University)(2021)
摘要
We extend the Approximate-Proximal Point (aProx) family of model-based methods for solving stochastic convex optimization problems, including stochastic subgradient, proximal point, and bundle methods, to the minibatch and accelerated setting. To do so, we propose specific model-based algorithms and an acceleration scheme for which we provide non-asymptotic convergence guarantees, which are order-optimal in all problem-dependent constants and provide linear speedup in minibatch size, while maintaining the desirable robustness traits (e.g. to stepsize) of the aProx family. Additionally, we show improved convergence rates and matching lower bounds identifying new fundamental constants for interpolation problems, whose importance in statistical machine learning is growing; this, for example, gives a parallelization strategy for alternating projections. We corroborate our theoretical results with empirical testing to demonstrate the gains accurate modeling, acceleration, and minibatching provide.
更多查看译文
关键词
stochastic optimization,optimal,parallel,model-based
AI 理解论文
溯源树
样例
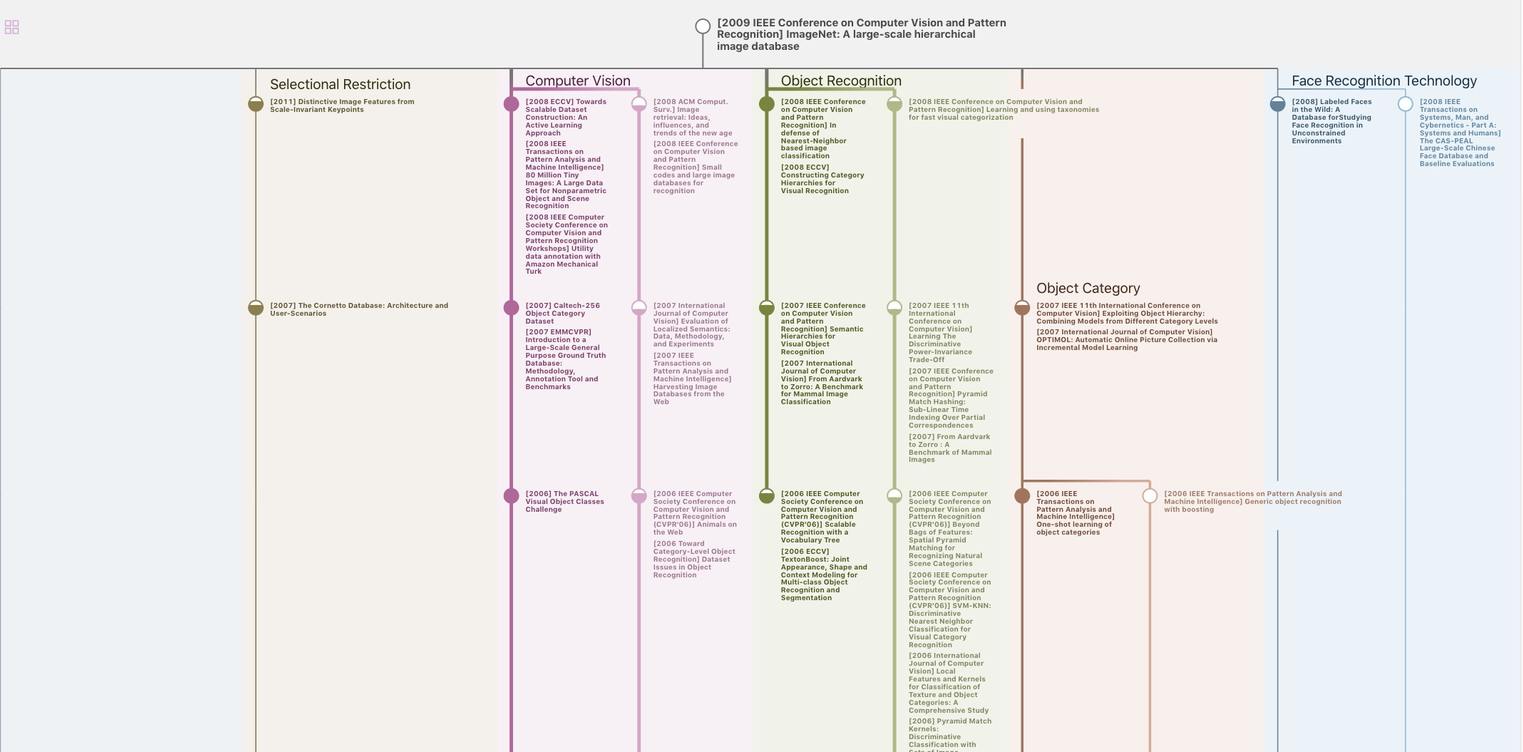
生成溯源树,研究论文发展脉络
Chat Paper
正在生成论文摘要