CLEVR_HYP: A Challenge Dataset and Baselines for Visual Question Answering with Hypothetical Actions over Images
arXiv (Cornell University)(2021)
摘要
Most existing research on visual question answering (VQA) is limited to information explicitly present in an image or a video. In this paper, we take visual understanding to a higher level where systems are challenged to answer questions that involve mentally simulating the hypothetical consequences of performing specific actions in a given scenario. Towards that end, we formulate a vision-language question answering task based on the CLEVR (Johnson et. al., 2017) dataset. We then modify the best existing VQA methods and propose baseline solvers for this task. Finally, we motivate the development of better vision-language models by providing insights about the capability of diverse architectures to perform joint reasoning over image-text modality. Our dataset setup scripts and codes will be made publicly available at https://github.com/shailaja183/clevr_hyp.
更多查看译文
关键词
challenge dataset,hypothetical actions,visual question
AI 理解论文
溯源树
样例
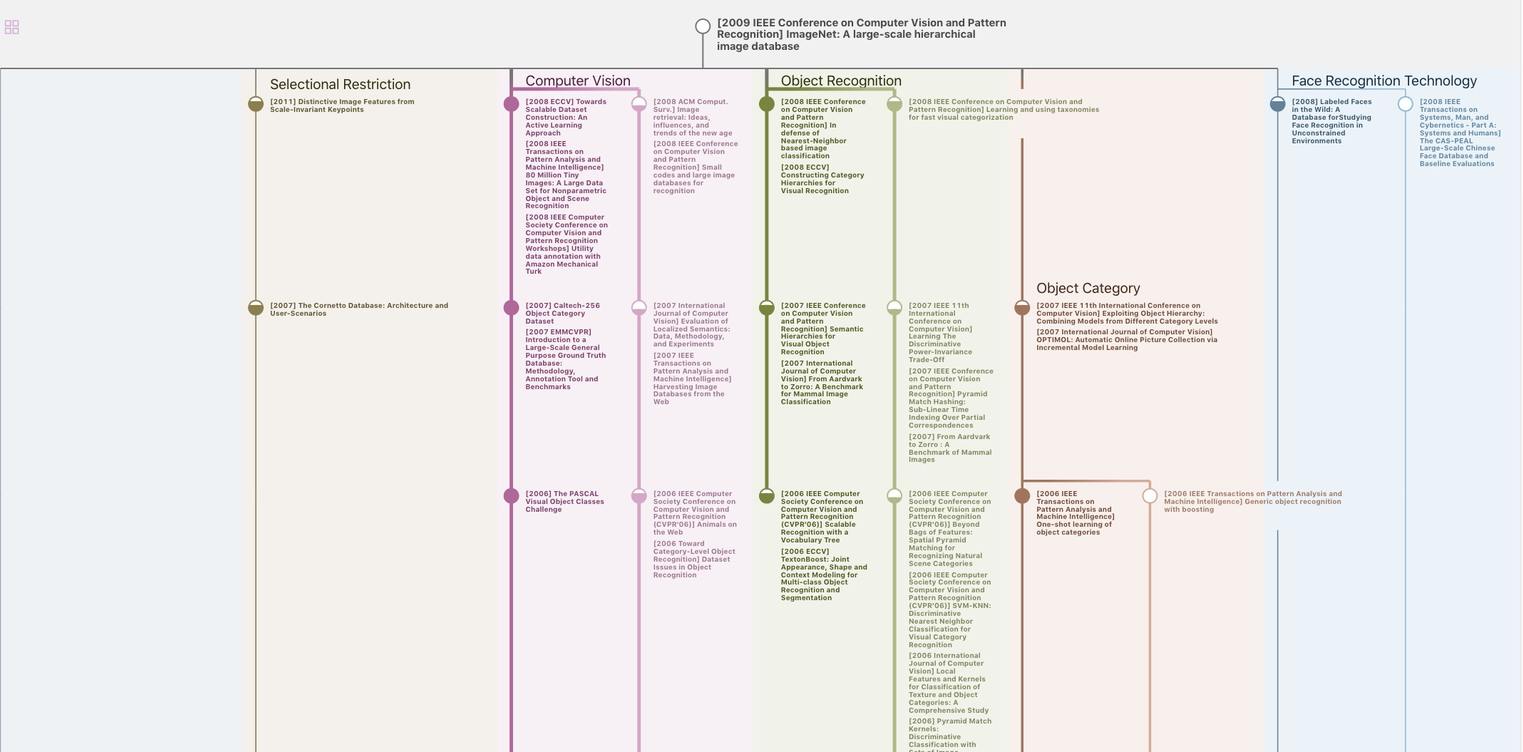
生成溯源树,研究论文发展脉络
Chat Paper
正在生成论文摘要