Pseudo-mask Matters inWeakly-supervised Semantic Segmentation
arXiv (Cornell University)(2021)
摘要
Most weakly supervised semantic segmentation (WSSS) methods follow the pipeline that generates pseudo-masks initially and trains the segmentation model with the pseudo-masks in fully supervised manner after. However, we find some matters related to the pseudo-masks, including high quality pseudo-masks generation from class activation maps (CAMs), and training with noisy pseudo-mask supervision. For these matters, we propose the following designs to push the performance to new state-of-art: (i) Coefficient of Variation Smoothing to smooth the CAMs adaptively; (ii) Proportional Pseudo-mask Generation to project the expanded CAMs to pseudo-mask based on a new metric indicating the importance of each class on each location, instead of the scores trained from binary classifiers. (iii) Pretended Under-Fitting strategy to suppress the influence of noise in pseudo-mask; (iv) Cyclic Pseudo-mask to boost the pseudo-masks during training of fully supervised semantic segmentation (FSSS). Experiments based on our methods achieve new state-of-art results on two changeling weakly supervised semantic segmentation datasets, pushing the mIoU to 70.0% and 40.2% on PAS-CAL VOC 2012 and MS COCO 2014 respectively. Codes including segmentation framework are released at https://github.com/Eli-YiLi/PMM
更多查看译文
关键词
pseudo-mask,inweakly-supervised
AI 理解论文
溯源树
样例
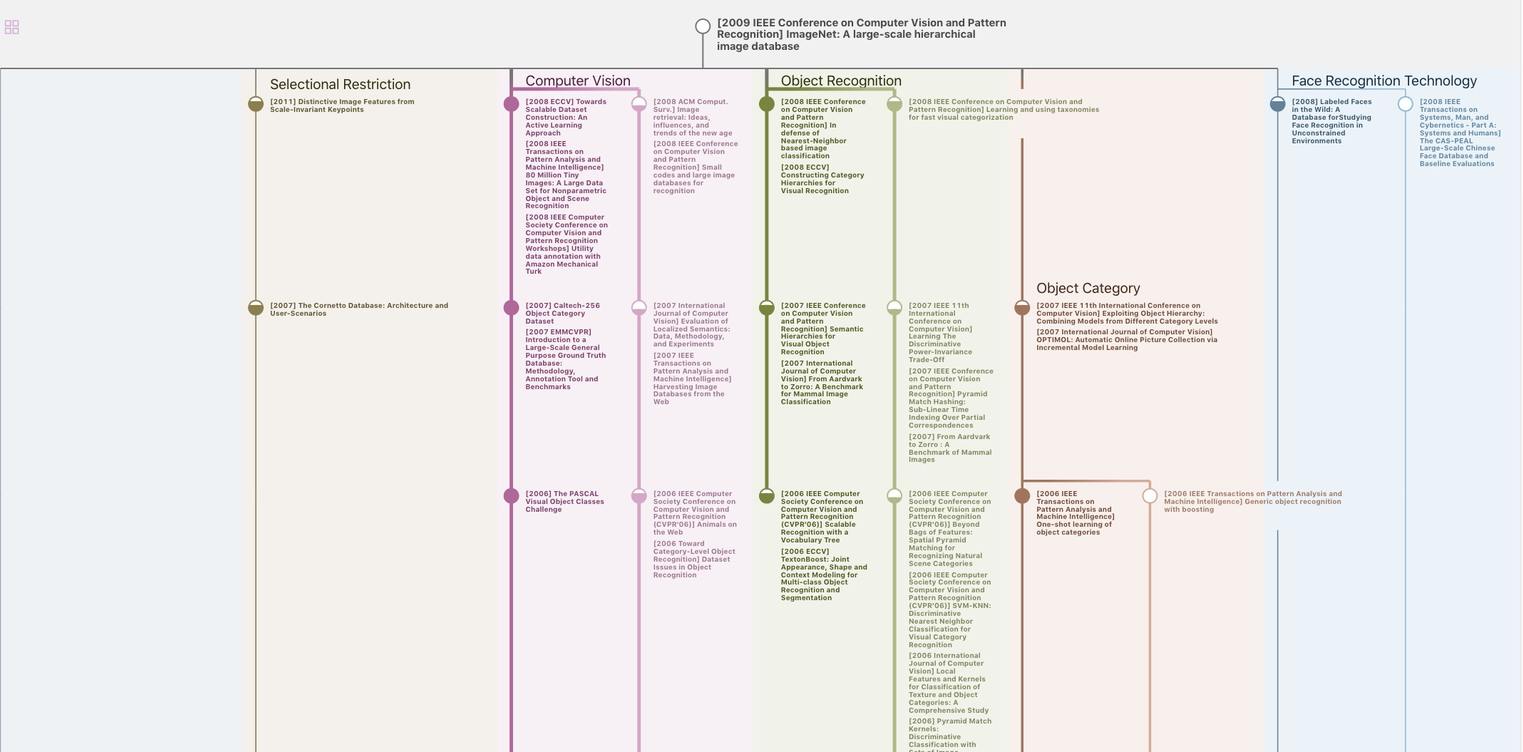
生成溯源树,研究论文发展脉络
Chat Paper
正在生成论文摘要