A Unified Frequency Domain Cross-Validatory Approach to HAC Standard Error Estimation
arXiv (Cornell University)(2023)
摘要
A unified frequency domain cross-validation (FDCV) method is proposed to obtain a heteroskedasticity and autocorrelation consistent (HAC) standard error. This method enables model/tuning parameter selection across both parametric and nonparametric spectral estimators simultaneously. The candidate class for this approach consists of restricted maximum likelihood-based (REML) autoregressive spectral estimators and lag-weights estimators with the Parzen kernel. Additionally, an efficient technique for computing the REML estimators of autoregressive models is provided. Through simulations, the reliability of the FDCV method is demonstrated, comparing favorably with popular HAC estimators such as Andrews-Monahan and Newey-West.
更多查看译文
关键词
Heteroskedasticity and autocorrelation consistency,Frequency domain cross-validation,Automatic tuning parameter selection,Spectrum estimation
AI 理解论文
溯源树
样例
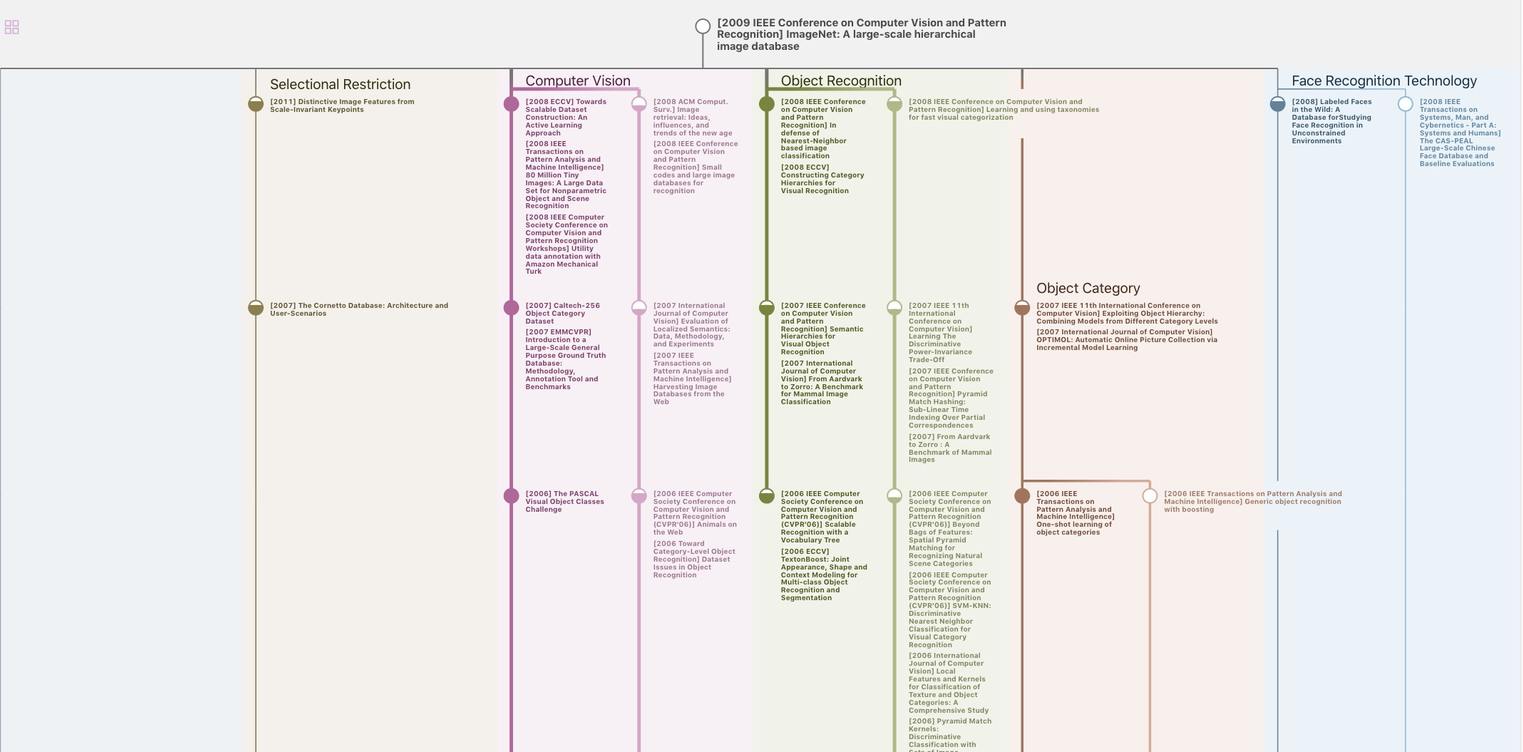
生成溯源树,研究论文发展脉络
Chat Paper
正在生成论文摘要