Evaluating Sensitivity to the Stick-Breaking Prior in Bayesian Nonparametrics
arXiv (Cornell University)(2021)
摘要
Bayesian models based on the Dirichlet process and other stick-breaking priors have been proposed as core ingredients for clustering, topic modeling, and other unsupervised learning tasks. Prior specification is, however, relatively difficult for such models, given that their flexibility implies that the consequences of prior choices are often relatively opaque. Moreover, these choices can have a substantial effect on posterior inferences. Thus, considerations of robustness need to go hand in hand with nonparametric modeling. In the current paper, we tackle this challenge by exploiting the fact that variational Bayesian methods, in addition to having computational advantages in fitting complex nonparametric models, also yield sensitivities with respect to parametric and nonparametric aspects of Bayesian models. In particular, we demonstrate how to assess the sensitivity of conclusions to the choice of concentration parameter and stick-breaking distribution for inferences under Dirichlet process mixtures and related mixture models. We provide both theoretical and empirical support for our variational approach to Bayesian sensitivity analysis.
更多查看译文
关键词
prior,bayesian
AI 理解论文
溯源树
样例
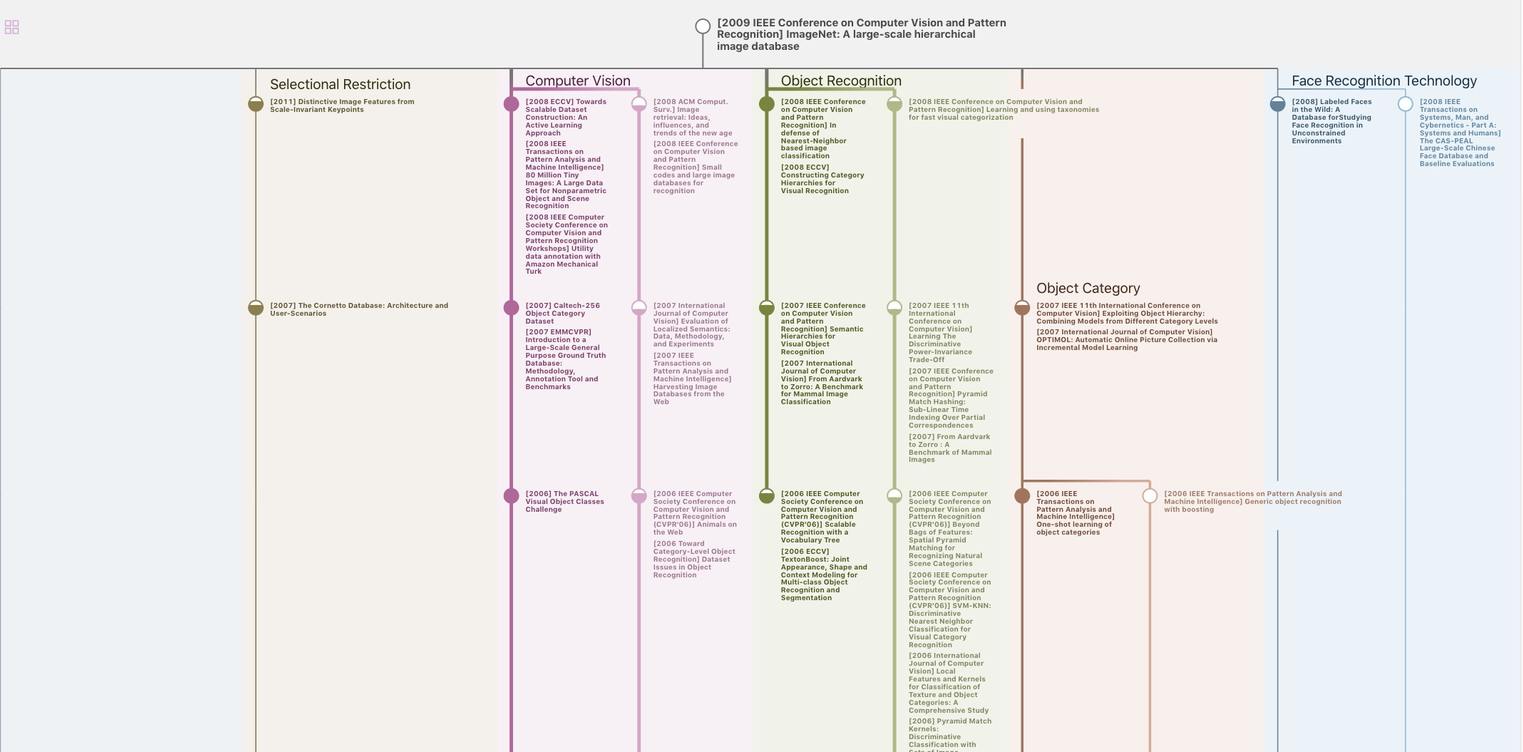
生成溯源树,研究论文发展脉络
Chat Paper
正在生成论文摘要