Learning Efficient Policies for Picking Entangled Wire Harnesses: A Solution to Industrial Bin Picking
arXiv (Cornell University)(2021)
摘要
Wire harnesses are essential connecting components in manufacturing industry but are challenging to be automated in industrial tasks such as bin picking. They are long, flexible and tend to get entangled when randomly placed in a bin. This makes it difficult for the robot to grasp a single one in dense clutter. Besides, training or collecting data in simulation is challenging due to the difficulties in modeling the combination of deformable and rigid components for wire harnesses. In this work, instead of directly lifting wire harnesses, we propose to grasp and extract the target following a circle-like trajectory until it is untangled. We learn a policy from real-world data that can infer grasps and separation actions from visual observation. Our policy enables the robot to efficiently pick and separate entangled wire harnesses by maximizing success rates and reducing execution time. To evaluate our policy, we present a set of real-world experiments on picking wire harnesses. Our policy achieves an overall 84.6% success rate compared with 49.2% in baseline. We also evaluate the effectiveness of our policy under different clutter scenarios using unseen types of wire harnesses. Results suggest that our approach is feasible for handling wire harnesses in industrial bin picking.
更多查看译文
关键词
industrial bin picking,efficient policies,wire harnesses
AI 理解论文
溯源树
样例
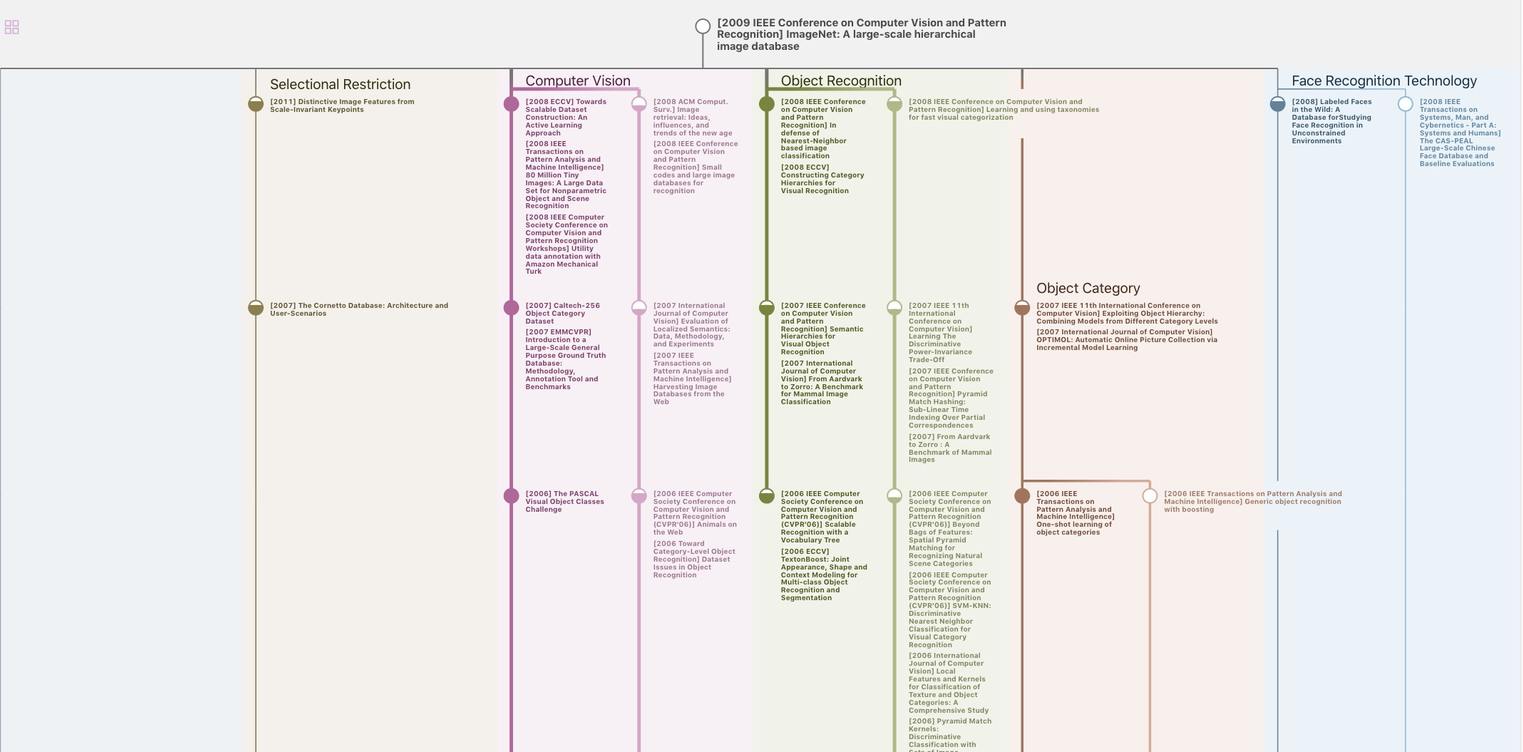
生成溯源树,研究论文发展脉络
Chat Paper
正在生成论文摘要