Robust Mean Estimation under Coordinate-level Corruption with Missing Entries
arXiv (Cornell University)(2020)
摘要
We study the problem of robust mean estimation and introduce a novel Hamming distance-based measure of distribution shift for coordinate-level corruptions. We show that this measure yields adversary models that capture more realistic corruptions than those used in prior works, and present an information-theoretic analysis of robust mean estimation techniques in these settings. We show that for structured distributions, methods that leverage the structure yield more accurate mean estimation. Finally, we introduce a novel two-step meta-algorithm for robust mean estimation that first fixes corruptions in the input data and then performs robust mean estimation. We demonstrate in real-world data with missing values that our two-step approach outperforms existing robust estimation methods and provides accurate mean estimation even in high-magnitude corruption settings.
更多查看译文
关键词
robust mean estimation,mean estimation,coordinate-level
AI 理解论文
溯源树
样例
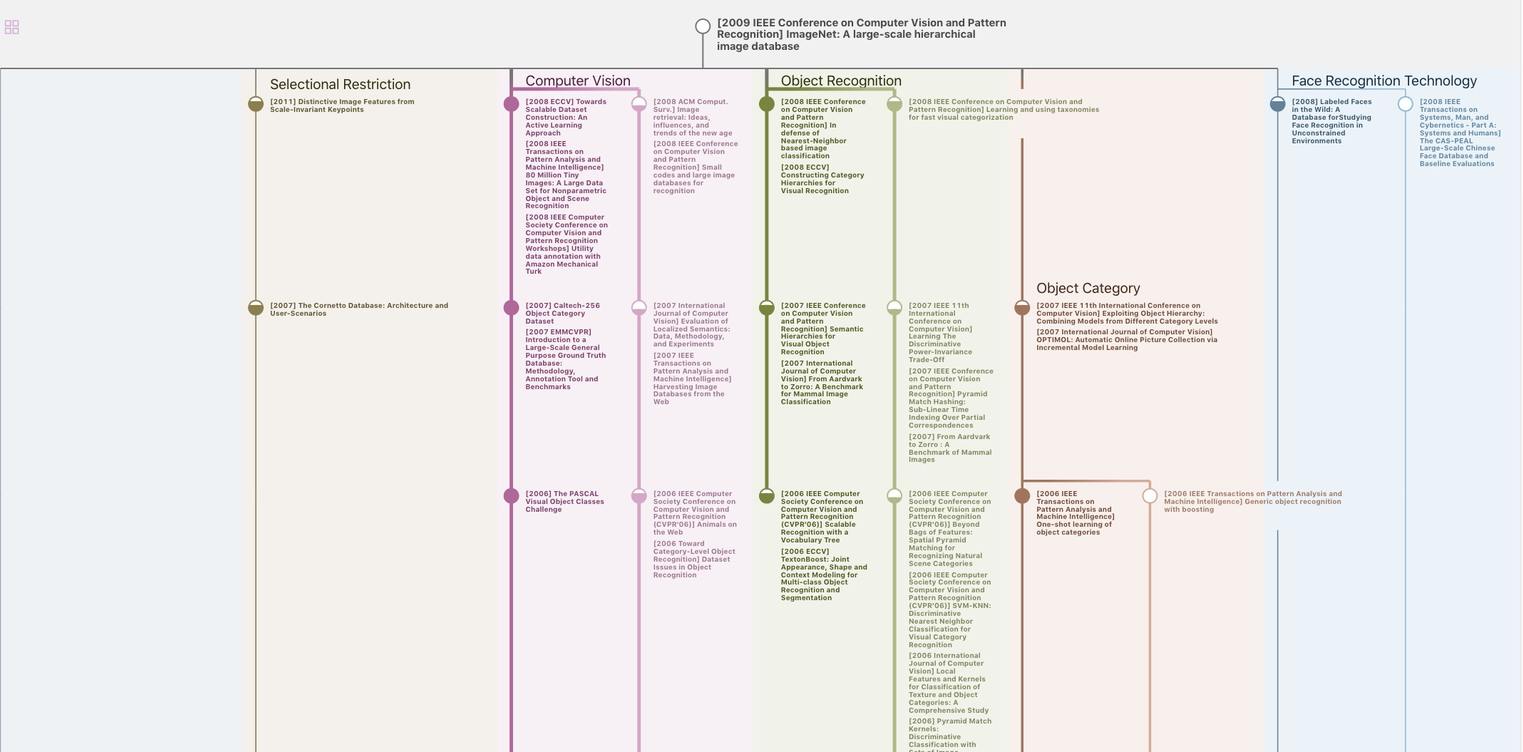
生成溯源树,研究论文发展脉络
Chat Paper
正在生成论文摘要