A general kernel boosting framework integrating pathways for predictive modeling based on genomic data
arXiv (Cornell University)(2020)
摘要
Predictive modeling based on genomic data has gained popularity in biomedical research and clinical practice by allowing researchers and clinicians to identify biomarkers and tailor treatment decisions more efficiently. Analysis incorporating pathway information can boost discovery power and better connect new findings with biological mechanisms. In this article, we propose a general framework, Pathway-based Kernel Boosting (PKB), which incorporates clinical information and prior knowledge about pathways for prediction of binary, continuous and survival outcomes. We introduce appropriate loss functions and optimization procedures for different outcome types. Our prediction algorithm incorporates pathway knowledge by constructing kernel function spaces from the pathways and use them as base learners in the boosting procedure. Through extensive simulations and case studies in drug response and cancer survival datasets, we demonstrate that PKB can substantially outperform other competing methods, better identify biological pathways related to drug response and patient survival, and provide novel insights into cancer pathogenesis and treatment response.
更多查看译文
关键词
general kernel,predictive
AI 理解论文
溯源树
样例
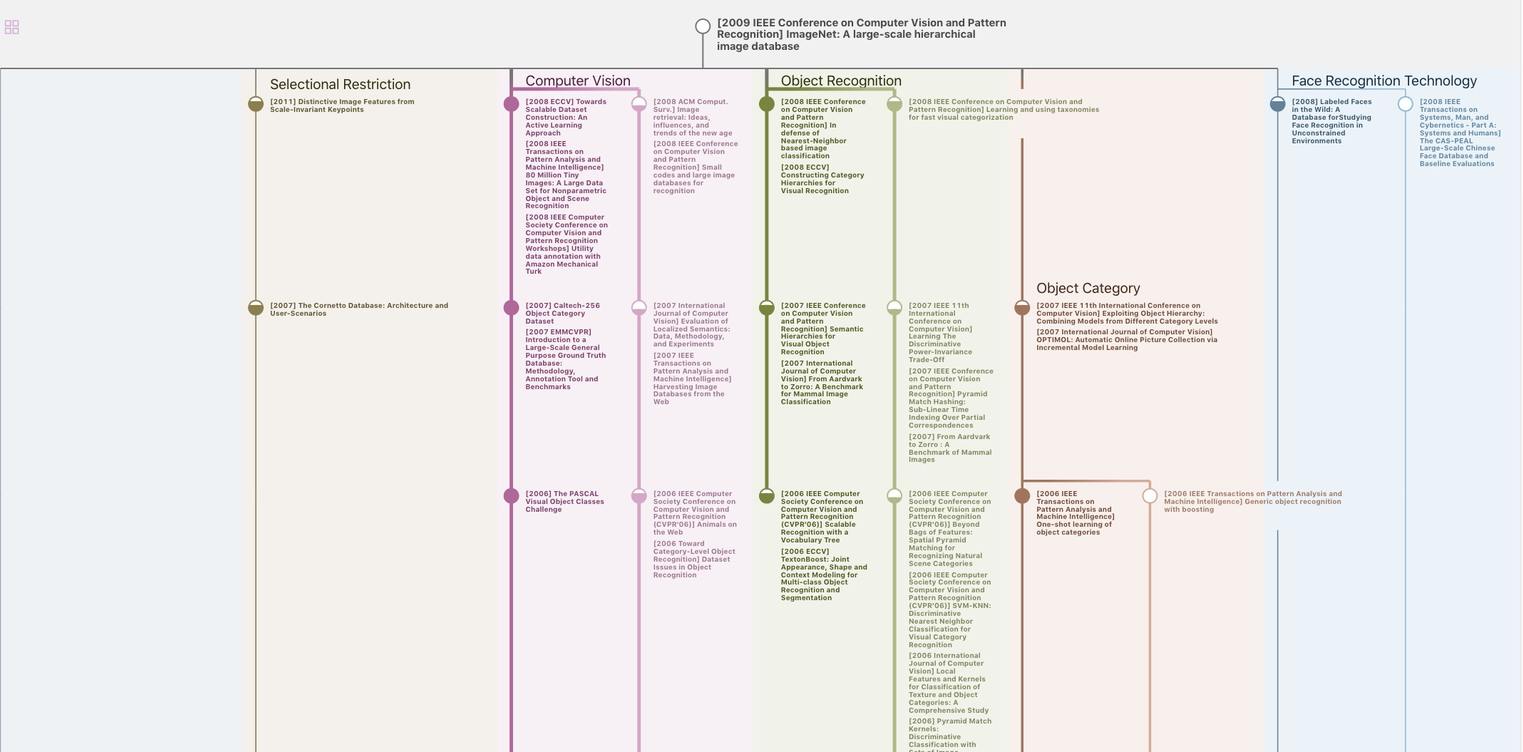
生成溯源树,研究论文发展脉络
Chat Paper
正在生成论文摘要