Brain-inspired global-local learning incorporated with neuromorphic computing
arXiv (Cornell University)(2020)
摘要
Two main routes of learning methods exist at present including error-driven global learning and neuroscience-oriented local learning. Integrating them into one network may provide complementary learning capabilities for versatile learning scenarios. At the same time, neuromorphic computing holds great promise, but still needs plenty of useful algorithms and algorithm-hardware co-designs for exploiting the advantages. Here, we report a neuromorphic hybrid learning model by introducing a brain-inspired meta-learning paradigm and a differentiable spiking model incorporating neuronal dynamics and synaptic plasticity. It can meta-learn local plasticity and receive top-down supervision information for multiscale synergic learning. We demonstrate the advantages of this model in multiple different tasks, including few-shot learning, continual learning, and fault-tolerance learning in neuromorphic vision sensors. It achieves significantly higher performance than single-learning methods, and shows promise in empowering neuromorphic applications revolution. We further implemented the hybrid model in the Tianjic neuromorphic platform by exploiting algorithm-hardware co-designs and proved that the model can fully utilize neuromorphic many-core architecture to develop hybrid computation paradigm.
更多查看译文
关键词
learning,brain-inspired,global-local
AI 理解论文
溯源树
样例
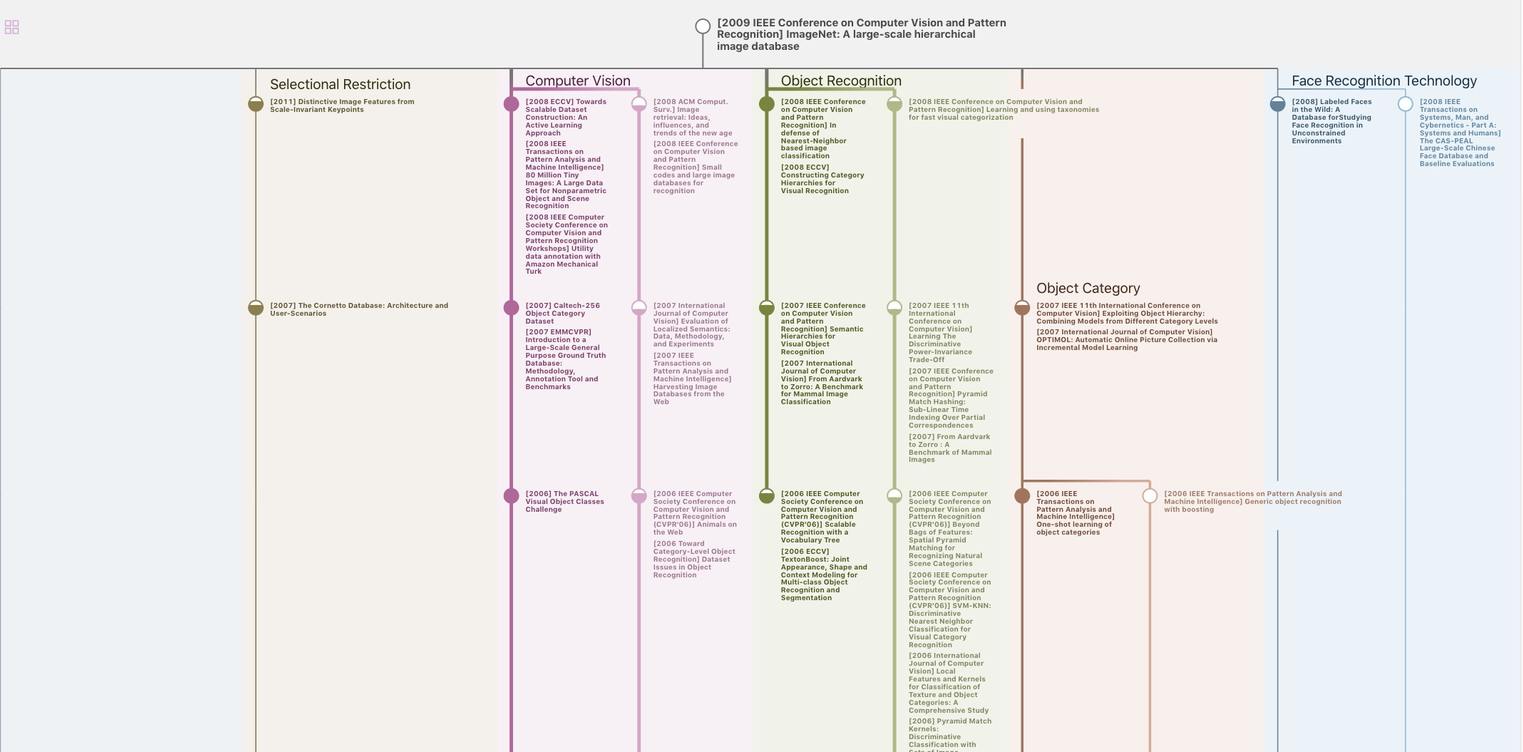
生成溯源树,研究论文发展脉络
Chat Paper
正在生成论文摘要