Challenges of Deep Learning Methods for COVID-19 Detection Using Public Datasets
medRxiv (Cold Spring Harbor Laboratory)(2020)
摘要
ABSTRACT A large number of studies in the past months have proposed deep learning-based Artificial Intelligence (AI) tools for automated detection of COVID-19 using publicly available datasets of Chest X-rays (CXRs) or CT scans for training and evaluation. Most of these studies report high accuracy when classifying COVID-19 patients from normal or other commonly occurring pneumonia cases. However, these results are often obtained on cross-validation studies without an independent test set coming from a separate dataset and have biases such as the two classes to be predicted come from two completely different datasets. In this work, we investigate potential overfitting and biases in such studies by designing different experimental setups within the available public data constraints and highlight the challenges and limitations of developing deep learning models with such datasets. We propose a deep learning architecture for COVID-19 classification that combines two very popular classification networks, ResNet and Xception, and use it to carry out the experiments to investigate challenges and limitations. The results show that the deep learning models can overestimate their performance due to biases in the experimental design and overfitting to the training dataset. We compare the proposed architecture to state-of-the-art methods utilizing an independent test set for evaluation, where some of the identified bias and overfitting issues are reduced. Although our proposed deep learning architecture gives the best performance with our best possible setup, we highlight the challenges in comparing and interpreting various deep learning algorithms’ results. While the deep learning-based methods using chest imaging data show promise in being helpful for clinical management and triage of COVID-19 patients, our experiments suggest that a larger, more comprehensive database with less bias is necessary for developing tools applicable in real clinical settings.
更多查看译文
关键词
deep learning methods,public datasets,deep learning,detection
AI 理解论文
溯源树
样例
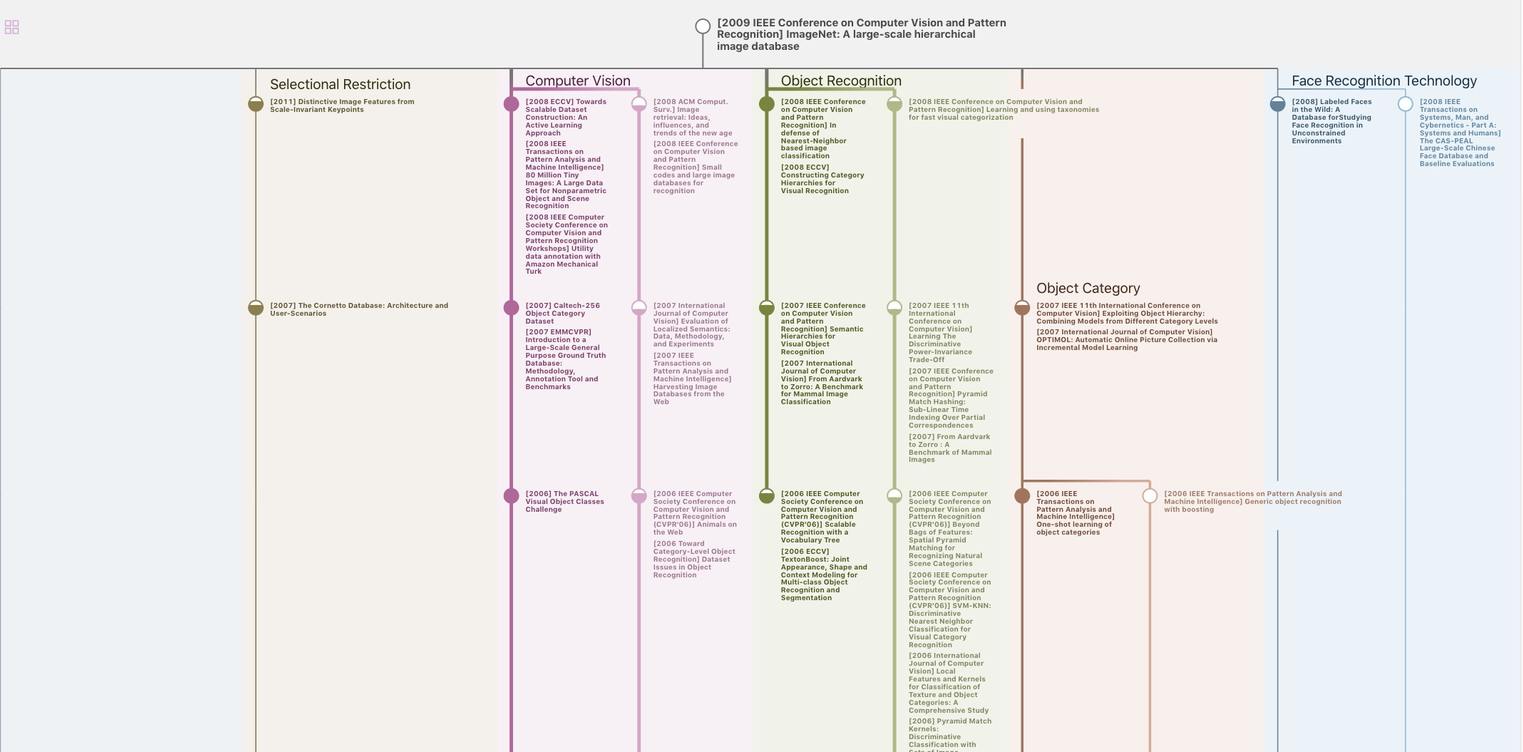
生成溯源树,研究论文发展脉络
Chat Paper
正在生成论文摘要