Why Neural Machine Translation Prefers Empty Outputs
arXiv (Cornell University)(2020)
摘要
We investigate why neural machine translation (NMT) systems assign high probability to empty translations. We find two explanations. First, label smoothing makes correct-length translations less confident, making it easier for the empty translation to finally outscore them. Second, NMT systems use the same, high-frequency EoS word to end all target sentences, regardless of length. This creates an implicit smoothing that increases zero-length translations. Using different EoS types in target sentences of different lengths exposes and eliminates this implicit smoothing.
更多查看译文
关键词
translation
AI 理解论文
溯源树
样例
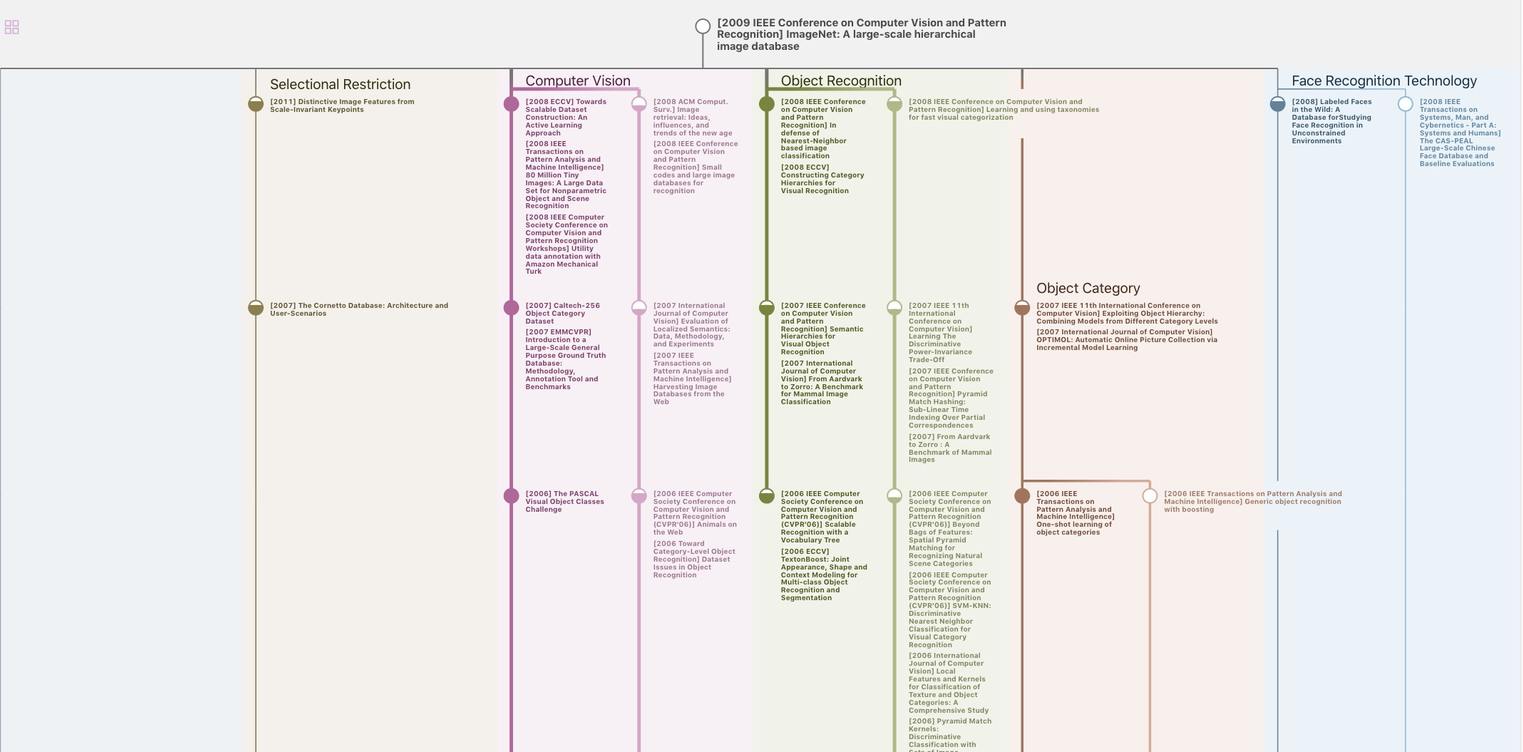
生成溯源树,研究论文发展脉络
Chat Paper
正在生成论文摘要