Machine-Learning-based Prediction of Lattice Thermal Conductivity for Half-Heusler Compounds using Atomic Information
arXiv (Cornell University)(2020)
摘要
The half-Heusler compound has drawn attention in a variety of fields as a candidate material for thermoelectric energy conversion and spintronics technology. This is because it has various electronic structures, such as semi-metals, semiconductors, and a topological insulator. When the half-Heusler compound is incorporated into the device, the control of high lattice thermal conductivity owing to high crystal symmetry is a challenge for the thermal manager of the device. The calculation for the prediction of lattice thermal conductivity, which is an important physical parameter for controlling the thermal management of the device, requires a calculation cost of several 100 times as much as the usual density functional theory calculation. Therefore, we examined whether lattice thermal conductivity prediction by machine learning was possible on the basis of only the atomic information of constituent elements for thermal conductivity calculated by the density functional theory calculation in various half-Heusler compounds. Consequently, we constructed a machine learning model, which can predict the lattice thermal conductivity with high accuracy from the information of only atomic radius and atomic mass of each site in the half-Heusler type crystal structure. Applying our results, the lattice thermal conductivity for an unknown half-Heusler compound can be immediately predicted. In the future, low-cost and short-time development of new functional materials can be realized, leading to breakthroughs in the search of novel functional materials.
更多查看译文
关键词
lattice thermal conductivity,thermal conductivity,machine-learning-based,half-heusler
AI 理解论文
溯源树
样例
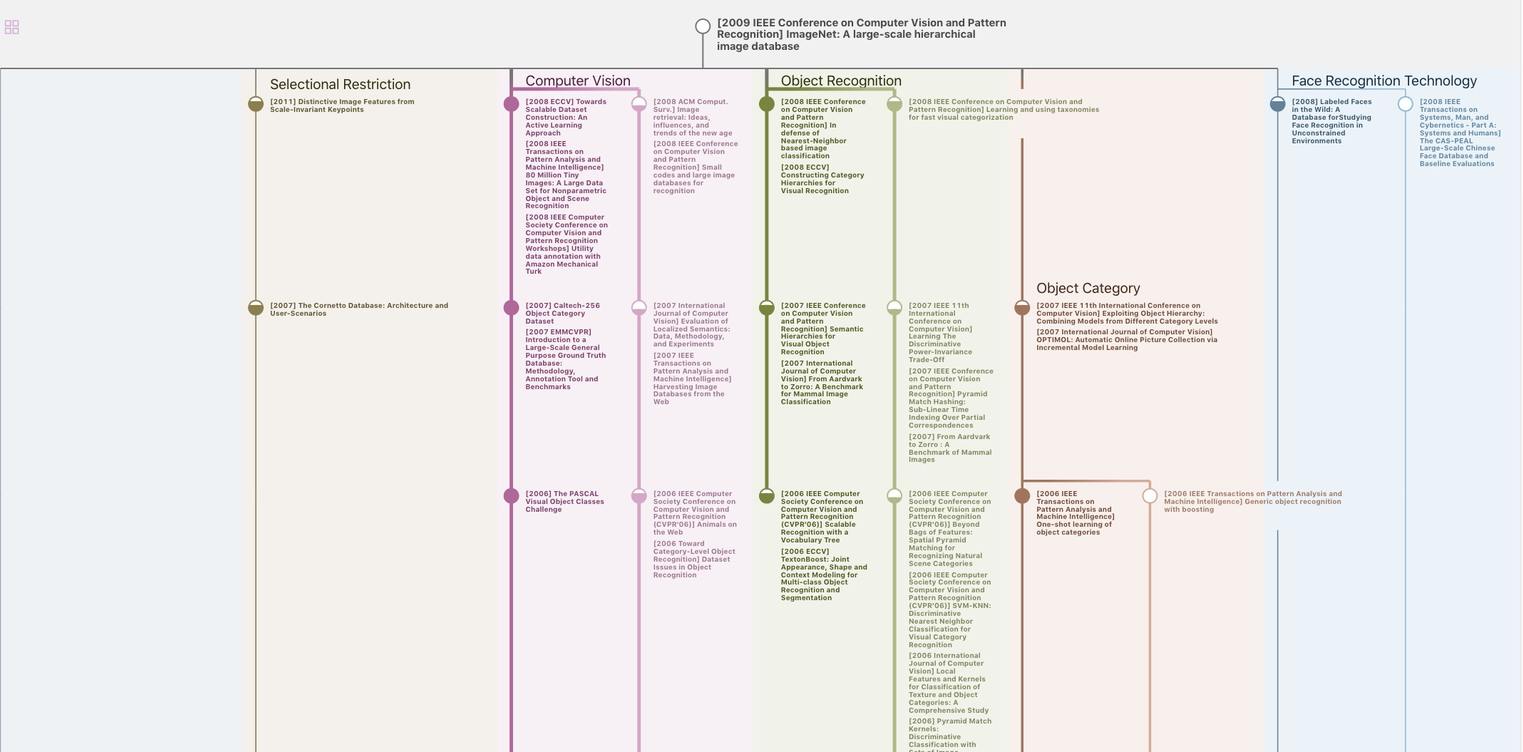
生成溯源树,研究论文发展脉络
Chat Paper
正在生成论文摘要