Decomposable Probability-of-Success Metrics in Algorithmic Search
arXiv (Cornell University)(2020)
摘要
Previous studies have used a specific success metric within an algorithmic search framework to prove machine learning impossibility results. However, this specific success metric prevents us from applying these results on other forms of machine learning, e.g. transfer learning. We define decomposable metrics as a category of success metrics for search problems which can be expressed as a linear operation on a probability distribution to solve this issue. Using an arbitrary decomposable metric to measure the success of a search, we demonstrate theorems which bound success in various ways, generalizing several existing results in the literature.
更多查看译文
关键词
algorithmic search,probability-of-success
AI 理解论文
溯源树
样例
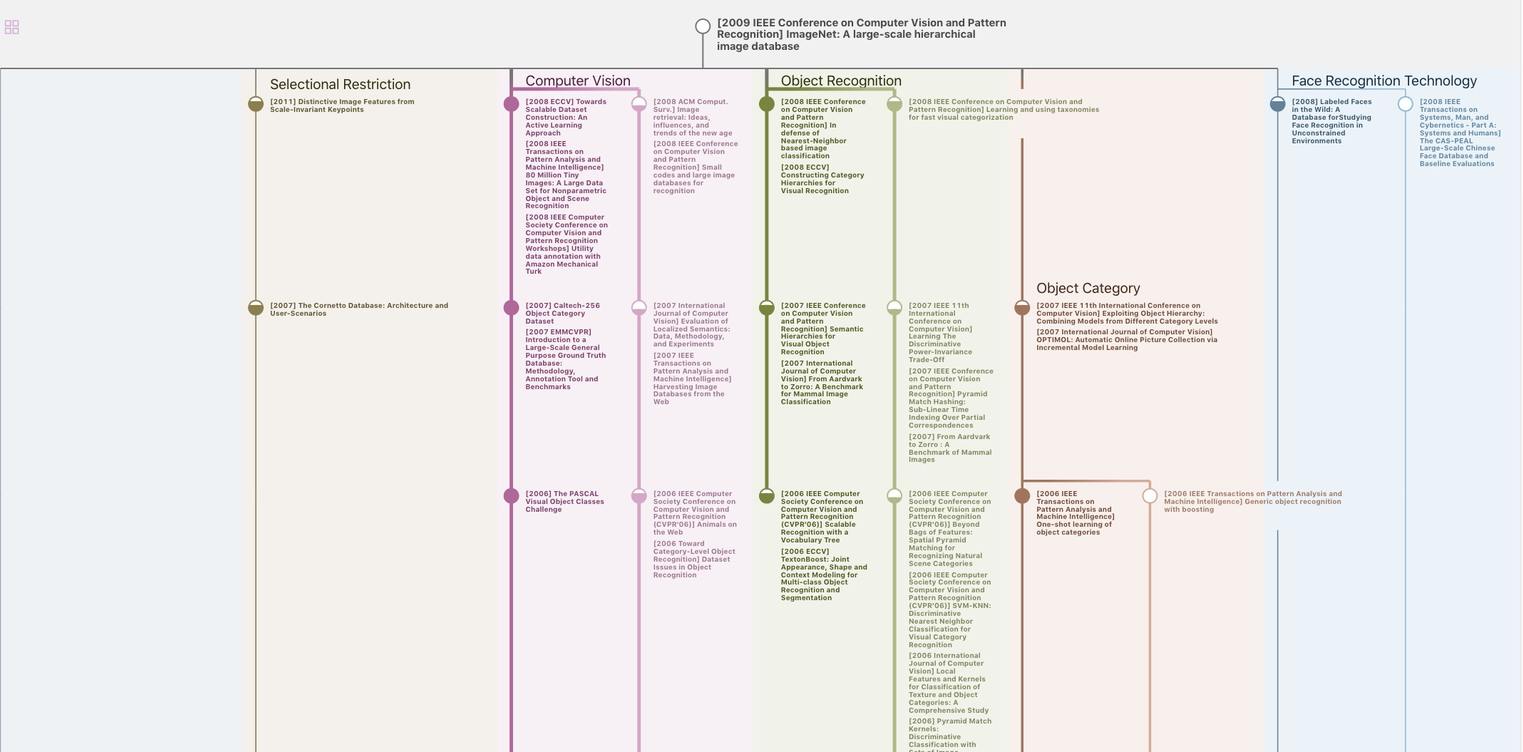
生成溯源树,研究论文发展脉络
Chat Paper
正在生成论文摘要