$\texttt{SpaceMath v.2.0}$ with Machine Learning. A $\texttt{Mathematica}$ package for Beyond the Standard Model parameter space searches
arXiv (Cornell University)(2020)
摘要
\texttt{SpaceMath v.2.0} with Machine Learning is an extension of the previous version which we implement observables related with LHC Higgs boson data and their projections for the High Luminosity and High Energy Large Hadron Collider. In this version we implemented processes with Flavor-Changing Neutral Currents at tree and one-loop level, namely, i) Radiative decays $\ell_i\to\ell_j \gamma$, ii) $\ell_i\to \ell_j \ell_k \bar{\ell}_k$ decays ($\ell_i=\tau,\,\mu$, $\ell_{j,\,k}=\mu,\,e$, with $\ell_i \neq\ell_j \neq\ell_k$) and iii) anomalous magnetic dipole moment of the muon $\delta a_\mu$. \texttt{SpaceMath v.2.0} is able to find allowed regions for free parameters of models with both real and complex singlets and real and complex doublets using the processes previously mentioned within a friendly interface and an intuitive environment in which the user enters the couplings symbolically, sets parameters and execute \texttt{Mathematica} in the traditional way. As result, both tables as plots with values and areas agree with experimental data are generated. We present examples using \texttt{SpaceMath v.2.0} to analyze the free \textit{Two-Higgs Doublet Model of type III} parameter space, step by step, in order to start new users in a fast and efficient way. Finally, we have implemented in this version of \texttt{SpaceMath} algorithms of Machine Learning to generate specific Benchmark Points to be used directly in numerical evaluations of calculations of physical observables.
更多查看译文
关键词
standard model parameter $\texttt{spacemath,machine learning
AI 理解论文
溯源树
样例
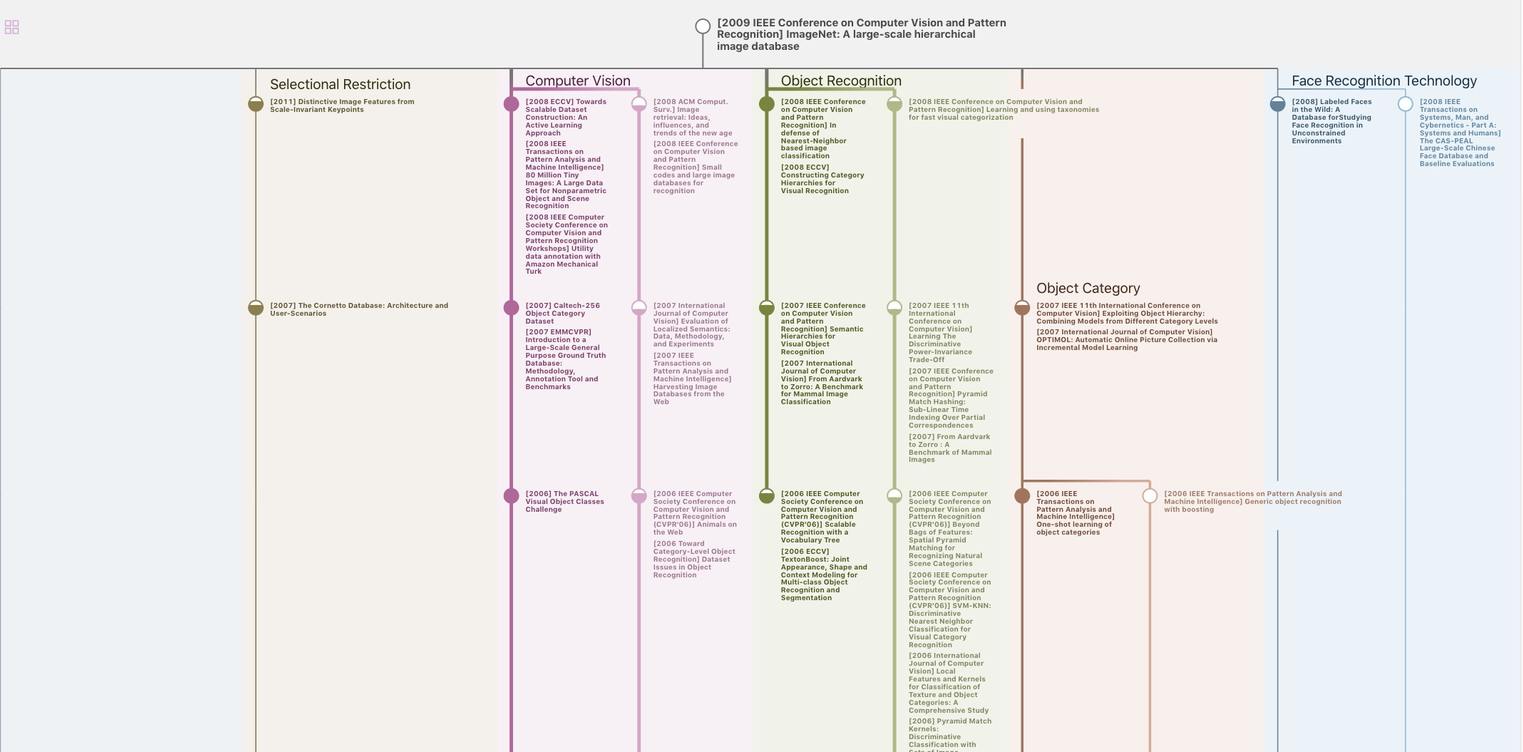
生成溯源树,研究论文发展脉络
Chat Paper
正在生成论文摘要